Data Observability Tool Comparison: great expectations vs. Sifflet
In the world of data management, ensuring the quality and reliability of your data is crucial. Data observability has emerged as a key practice to achieve this goal, enabling organizations to gain insights, make informed decisions, and drive business outcomes. In this article, we will compare two leading data observability tools: Great Expectations and Sifflet. By understanding their features, pros and cons, and other important factors, you can make an informed decision on which tool is the right fit for your organization's data observability needs.
Understanding Data Observability
Data observability is the practice of monitoring and managing your data quality, integrity, and reliability throughout its lifecycle. It involves ensuring that data meets specific criteria, conforms to expected patterns, and is free from errors or inconsistencies. By implementing effective data observability processes, organizations can gain confidence in their data and make accurate, data-driven decisions.
The Importance of Data Observability
Why is data observability important? The answer lies in the fact that data is the lifeblood of modern organizations. Without high-quality data, businesses may make faulty decisions, lose customer trust, or miss out on valuable opportunities. Data observability helps mitigate these risks by providing real-time insights into the health and quality of your data, empowering you to identify and rectify issues before they impact critical business processes.
Key Components of Data Observability
Before delving into the comparison of Great Expectations and Sifflet, let's understand the key components of data observability. These components lay the foundation for effective data management and quality assurance:
- Data Monitoring: Tracking the quality and performance of your data by measuring predefined metrics and thresholds.
- Data Validation: Verifying that your data adheres to specific rules, patterns, or expectations, ensuring its accuracy and integrity.
- Anomaly Detection: Identifying and flagging unusual or unexpected data patterns or behaviors that may indicate errors or issues.
- Data Documentation: Creating comprehensive documentation that describes your data, its structure, and its expected behavior to aid understanding and troubleshooting.
- Data Lineage: Tracing the origin, transformations, and dependencies of your data to provide a clear picture of its journey and ensure accountability.
Let's dive deeper into each of these key components to gain a better understanding of their significance:
Data Monitoring: Monitoring your data is crucial for maintaining its quality and performance. By tracking predefined metrics and thresholds, you can proactively identify any deviations or anomalies that may affect the reliability of your data. This real-time monitoring allows you to take immediate action, ensuring that your data remains accurate and trustworthy.
Data Validation: Data validation is the process of verifying that your data meets specific rules, patterns, or expectations. By implementing validation checks, you can ensure that your data is accurate, complete, and consistent. This helps prevent data errors and inconsistencies, allowing you to have confidence in the integrity of your data.
Anomaly Detection: Anomaly detection plays a crucial role in data observability by identifying unusual or unexpected data patterns or behaviors. These anomalies may indicate errors, issues, or even potential security breaches. By promptly detecting and addressing these anomalies, you can maintain the reliability and security of your data.
Data Documentation: Comprehensive documentation is essential for understanding and troubleshooting your data. It provides a clear description of your data, its structure, and its expected behavior. This documentation helps data analysts, scientists, and other stakeholders gain insights into the data, enabling them to make informed decisions and resolve any issues that may arise.
Data Lineage: Data lineage is the process of tracing the origin, transformations, and dependencies of your data. It provides a clear picture of how your data has evolved and ensures accountability throughout its journey. By understanding the lineage of your data, you can easily identify any potential issues, track data changes, and make informed decisions based on the data's history.
By incorporating these key components into your data observability practices, you can establish a robust framework for managing and ensuring the quality, integrity, and reliability of your data. This, in turn, empowers your organization to make accurate, data-driven decisions and stay ahead in today's data-driven world.
Introduction to Great Expectations
Great Expectations is an open-source data observability tool that helps you maintain data quality, validate data pipelines, and ensure that your data meets expectations. Let's explore its key features, as well as its pros and cons:
Features of Great Expectations
Great Expectations offers a wide range of features to support your data observability efforts:
- Declarative Assertions: Great Expectations allows you to define expectations about your data using a declarative syntax, making it easy to express complex validation rules.
- Data Profiling: Great Expectations automatically profiles your data, providing statistical summaries and insights to help you understand its characteristics and detect anomalies.
- Continuous Integration: Great Expectations integrates with popular data pipeline orchestration tools, enabling you to validate data in real-time as it flows through your pipelines.
- Extensive Data Connectors: Great Expectations supports a wide variety of data sources and offers connectors to popular databases, data lakes, and cloud platforms, ensuring seamless integration with your existing ecosystem.
- Data Documentation Generation: Great Expectations automatically generates comprehensive documentation for your data, keeping it up-to-date and readily accessible to your team.
Pros and Cons of Great Expectations
Like any tool, Great Expectations has its strengths and weaknesses. Let's explore some of its pros and cons:
Pros:
- Open-source and community-driven, which means a large and active user community.
- Flexible and extensible, allowing you to tailor it to your specific data observability needs.
- Integrates well with existing data stack components.
Cons:
- Steep learning curve for non-technical users due to its Python-based interface.
- Documentation can be overwhelming for beginners.
- Limited support for certain data sources or specific data validation requirements.
Introduction to Sifflet
Sifflet, on the other hand, is a commercial data observability tool that aims to simplify the process of monitoring and validating data quality. Here's an overview of its features, as well as its pros and cons:
Features of Sifflet
Sifflet offers a range of features designed to make data observability more accessible for users:
- Intuitive User Interface: Sifflet provides a user-friendly interface that allows users without extensive technical knowledge to configure and manage data observability tasks.
- Automated Data Validation: Sifflet automates data quality monitoring and validation, reducing the need for manual configuration and intervention.
- Alerting and Notifications: Sifflet proactively alerts users about data issues or anomalies, enabling timely remediation.
- Prebuilt Connectors: Sifflet offers prebuilt connectors for popular data sources, simplifying integration with your existing data infrastructure.
- Collaboration and Sharing: Sifflet allows users to collaborate on data observability projects and share insights and best practices.
Pros and Cons of Sifflet
Let's consider some of the pros and cons of using Sifflet for data observability:
Pros:
- Intuitive and user-friendly interface, making it accessible to users with limited technical expertise.
- Automated configuration and management of data observability tasks.
- Centralized dashboard for monitoring and managing data quality, providing a holistic view of your data ecosystem.
Cons:
- Commercial tool with licensing costs, which may be a barrier for organizations with tight budgets.
- Less customization and extensibility compared to open-source alternatives like Great Expectations.
- May have limited flexibility for complex data validation rules or unique use cases.
Detailed Comparison Between Great Expectations and Sifflet
Now that we have explored the features and pros and cons of both Great Expectations and Sifflet, let's delve deeper into a detailed comparison of the two tools. This comparison will focus on three key aspects: performance, user experience, and integration and compatibility.
Performance Comparison
When it comes to performance, Great Expectations and Sifflet have their own strengths and weaknesses. Great Expectations, being an open-source tool, provides greater customization and flexibility. However, this flexibility comes at the cost of a steeper learning curve and potential performance trade-offs. On the other hand, Sifflet offers a more user-friendly interface and simplified configuration, but may have limitations in terms of customizability for complex data validation scenarios.
User Experience Comparison
User experience is a critical aspect to consider when choosing a data observability tool. Great Expectations, being more technical in nature, requires users to have a strong understanding of Python and data validation concepts. This may be a barrier for non-technical users. In contrast, Sifflet's intuitive interface and automated configuration make it more accessible to users without extensive technical knowledge. However, it is important to note that this ease of use may come at the cost of flexibility and extensibility.
Integration and Compatibility Comparison
Integration and compatibility with existing data infrastructure are crucial considerations for any data observability tool. Great Expectations, being open source, offers extensive support for various data sources and integration with popular data orchestration tools. This makes it highly compatible with a wide range of data environments. Sifflet, on the other hand, provides prebuilt connectors for popular data sources, simplifying integration but potentially limiting compatibility with less common or customized data ecosystems.
Choosing the Right Data Observability Tool
Choosing the right data observability tool depends on various factors, including your organization's specific data requirements, technical expertise, and budgetary constraints. Here are some key factors to consider:
Factors to Consider
- Data Complexity: Consider the complexity of your data and the level of customization required for your data validation rules.
- Technical Expertise: Assess the technical expertise of your team and their familiarity with programming languages like Python.
- Integration Requirements: Evaluate the compatibility of the tool with your existing data infrastructure and the availability of prebuilt connectors.
- Usability and Learning Curve: Determine the ease of use and learning curve associated with the tool, keeping in mind the skill set of your team.
- Budget: Consider the licensing costs and ongoing support expenses associated with commercial tools like Sifflet.
Making the Decision
Ultimately, the decision between Great Expectations and Sifflet depends on your organization's unique needs and circumstances. Consider conducting a proof of concept or pilot project to evaluate the tools in your specific environment. This will help you gain hands-on experience and make an informed decision based on real-world scenarios.
In conclusion, both Great Expectations and Sifflet are powerful data observability tools that offer unique features and advantages. By understanding their strengths, weaknesses, and considerations, you can select the tool that aligns best with your organization's goals and requirements. Remember, data observability is not a one-size-fits-all approach. It requires careful evaluation and consideration to ensure your data is truly observable, reliable, and valuable to your organization's success.
As you consider the right data observability tool for your organization, remember that the journey doesn't end there. With CastorDoc, you can elevate your data management to new heights. Our platform integrates advanced governance, cataloging, and lineage capabilities with a user-friendly AI assistant, enabling self-service analytics that cater to both data professionals and business users alike. CastorDoc's conversational interface and natural language processing make it an indispensable partner in your data governance lifecycle. Ready to transform how your organization manages and leverages data? Check out more tools comparisons here and discover how CastorDoc can help you achieve a seamless, informed decision-making process.
You might also like
Get in Touch to Learn More
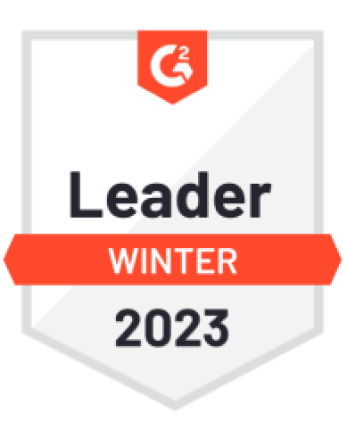
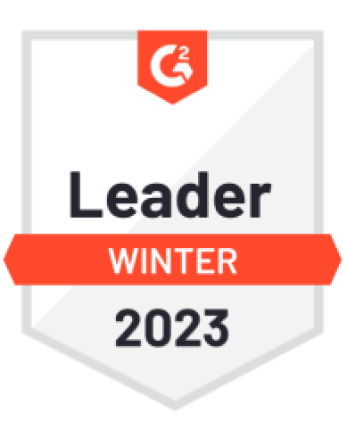

“[I like] The easy to use interface and the speed of finding the relevant assets that you're looking for in your database. I also really enjoy the score given to each table, [which] lets you prioritize the results of your queries by how often certain data is used.” - Michal P., Head of Data