Data Observability Tool Comparison: Soda vs. Datafold
Data observability has become crucial in the world of data management, where organizations rely heavily on data-driven decision-making. In this article, we will compare two prominent data observability tools: Soda and Datafold. By examining their features, functionality, and pricing structures, we aim to provide insights that will help you make an informed decision about which tool is best suited for your business needs.
Understanding Data Observability
Data observability refers to the ability to gain insights into the quality, accuracy, and reliability of data. It ensures that data pipelines and processes are monitored, enabling organizations to proactively identify and rectify issues that could compromise data integrity. With data observability, businesses can have confidence in the accuracy of their data analyses, enabling them to make informed decisions with confidence.
The Importance of Data Observability
Data observability plays a vital role in ensuring the trustworthiness of data. It enables organizations to identify and address anomalies, inconsistencies, and errors in their data pipelines. By monitoring the quality of data in real-time, businesses can identify and rectify issues promptly, reducing the risk of making decisions based on inaccurate or incomplete information.
Furthermore, data observability helps organizations meet regulatory compliance requirements, particularly in industries with strict data governance standards. By ensuring data accuracy and reliability, businesses can maintain compliance and avoid costly penalties associated with non-compliance.
But what exactly does data observability entail? Let's dive deeper into the key features of data observability tools that help organizations achieve these goals.
Key Features of Data Observability Tools
Data observability tools typically offer a range of features to help organizations monitor and improve data quality. Some key features to consider when evaluating data observability tools include:
- Real-time monitoring: The ability to monitor data pipelines and processes in real-time, allowing for prompt issue identification and resolution. This feature ensures that any issues or anomalies are detected and addressed as soon as they occur, minimizing the impact on data integrity.
- Data quality checks: Pre-built checks and validations to ensure data accuracy, integrity, and consistency. These checks help organizations identify and rectify any issues with data quality, such as missing or incorrect values, ensuring that the data used for analysis is reliable and trustworthy.
- Alerting and notifications: Customizable alerts and notifications to inform users of anomalies or potential data issues. This feature ensures that relevant stakeholders are promptly notified when there are deviations from expected data patterns, allowing for immediate action to be taken.
- Data lineage and metadata management: The ability to track data lineage and manage metadata, providing visibility into the origin and transformation of data. This feature helps organizations understand the journey of their data, from its source to its destination, ensuring transparency and accountability in data processes.
- Collaboration and reporting: Tools to facilitate collaboration among data teams and generate comprehensive reports on data quality and observability metrics. This feature enables seamless communication and collaboration between different stakeholders involved in data management, ensuring that everyone has access to the necessary information to make informed decisions.
By leveraging these key features, organizations can enhance their data observability capabilities, ensuring that their data is accurate, reliable, and trustworthy. With a robust data observability strategy in place, businesses can make data-driven decisions with confidence, driving growth and success in today's data-driven world.
An Introduction to Soda
Soda is a powerful data observability tool that helps organizations ensure the quality and reliability of their data. It offers a range of features designed to monitor and validate data pipelines, providing insights into data anomalies and issues.
Overview of Soda's Functionality
Soda offers a user-friendly interface that allows users to define and customize data quality checks. It supports a variety of data sources, including databases, data lakes, and streaming platforms. By defining data expectations, Soda verifies the accuracy, integrity, and completeness of data, thereby enabling users to identify and resolve data issues proactively.
Soda also provides real-time monitoring capabilities, allowing users to track data quality metrics and receive notifications for anomalies or outliers. With its robust collaboration features, teams can work together to improve data quality and ensure data observability across the organization.
Pros and Cons of Soda
Like any tool, Soda has its strengths and weaknesses. Some of the pros of using Soda include:
- Intuitive user interface that simplifies the process of defining and monitoring data quality checks.
- Real-time monitoring capabilities enable prompt issue identification and resolution.
- Robust collaboration features facilitate teamwork and knowledge sharing among data teams.
However, Soda does have a few drawbacks to consider:
- Limited integrations with external tools and platforms, which may require additional effort for data integration.
- Relatively higher learning curve compared to some other data observability tools.
An Introduction to Datafold
Datafold is another leading data observability tool that specializes in data testing and monitoring. Its comprehensive features aim to simplify the process of identifying and addressing data issues, ensuring data quality and observability.
Overview of Datafold's Functionality
Datafold provides a user-friendly interface that allows users to set up and manage data tests. It supports various data sources and offers pre-built tests for commonly encountered data quality issues. With Datafold, users can track data quality trends, identify data anomalies, and gain insights into the health of their data pipelines.
Additionally, Datafold's data lineage capabilities enable users to understand the origin and transformation of data, providing contextual information for issue diagnosis and resolution. Its alerting and reporting features allow users to stay informed about data issues and automate the generation of comprehensive reports.
Pros and Cons of Datafold
Datafold offers several advantages that make it a compelling choice for data observability:
- Easy-to-use interface, making it accessible to both technical and non-technical users.
- Comprehensive data testing capabilities, including pre-built tests for common data quality issues.
- Powerful data lineage and metadata management features that provide valuable context for issue resolution.
However, it's important to consider the following potential drawbacks of using Datafold:
- Limited integration options with some data sources and platforms, requiring additional effort for data integration.
- Relatively higher pricing compared to some other data observability tools.
Detailed Comparison of Soda and Datafold
Comparison of User Interface
Both Soda and Datafold offer user-friendly interfaces, but there are some differences to consider. Soda's interface is intuitive and visually appealing, making it easy for users to define and monitor data quality checks. On the other hand, Datafold's interface is designed to be accessible to both technical and non-technical users, focusing on simplicity and ease of use.
The choice between the two ultimately depends on the preferences and technical expertise of your data team. If you value a visually pleasing and intuitive interface, Soda might be the better choice. However, if simplicity and accessibility are top priorities, Datafold could be the preferred option.
Comparison of Data Monitoring Capabilities
When it comes to data monitoring, both Soda and Datafold excel in providing real-time insights into data quality. Soda allows users to define and customize data quality checks, providing comprehensive visibility into the health of data pipelines. Datafold, on the other hand, emphasizes data testing and offers pre-built tests for common data quality issues.
Ultimately, the choice between the two depends on your organization's specific data monitoring requirements. If you prefer a tool that offers flexibility in defining custom data quality checks, Soda may be the better fit. However, if you prioritize pre-built tests and simplicity in data testing, Datafold could be the preferred choice.
Comparison of Alerting and Reporting Features
Both Soda and Datafold offer robust alerting and reporting features to keep users informed about anomalies and issues. Soda provides customizable notifications and allows users to set up alerts based on specific data quality thresholds. Datafold, on the other hand, offers automated alerts and notifications, ensuring that users are promptly informed about data issues.
When it comes to reporting, Soda enables users to generate comprehensive reports on data quality metrics and observability. Datafold also offers reporting capabilities, with an emphasis on providing valuable insights into data trends and issue resolution.
The choice between Soda and Datafold in terms of alerting and reporting depends on your organization's preferences and reporting needs. If you require a high level of customization and control over your alerts, Soda might be the better choice. However, if you value automated alerts and insights into data trends, Datafold could be the preferred option.
Pricing Analysis: Soda vs. Datafold
Understanding Soda's Pricing Structure
Soda's pricing structure is typically based on factors such as the volume of data processed, the complexity of data quality checks, and the number of users. To get accurate pricing information for your specific requirements, it's recommended to reach out to Soda's sales team who can provide you with detailed pricing and licensing options.
Understanding Datafold's Pricing Structure
Datafold also offers a flexible pricing structure based on factors such as the volume of data processed, the number of users, and additional features required. To obtain specific pricing details tailored to your organization's needs, it's advisable to get in touch with Datafold's sales representatives.
In conclusion, both Soda and Datafold are powerful data observability tools that offer valuable insights into data quality and integrity. Soda provides a user-friendly interface and robust collaboration features, while Datafold emphasizes simplicity and comprehensive data testing capabilities. Ultimately, the decision between the two tools depends on your organization's specific requirements, preferences, and budget. By evaluating their features, functionality, and pricing structures, you can make an informed decision that best aligns with your data observability needs.
While Soda and Datafold offer compelling features for data observability, CastorDoc takes a holistic approach to data management by integrating advanced governance, cataloging, and lineage capabilities with a user-friendly AI assistant. This powerful combination enables businesses to engage in self-service analytics and empowers users across the spectrum, from data professionals to business stakeholders, to harness the full potential of their data. For a deeper understanding of how CastorDoc can complement your data observability and governance strategy, and to explore more tool comparisons, check out more tools comparisons here.
You might also like
Get in Touch to Learn More
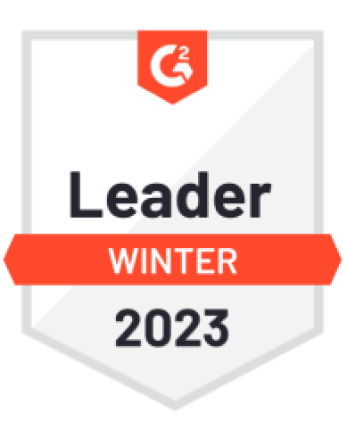
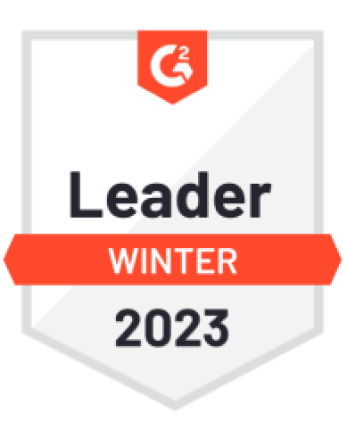

“[I like] The easy to use interface and the speed of finding the relevant assets that you're looking for in your database. I also really enjoy the score given to each table, [which] lets you prioritize the results of your queries by how often certain data is used.” - Michal P., Head of Data