Leveraging AI for Graph Creation: Enhance Your Data Representation
Discover how to harness the power of artificial intelligence to revolutionize your data representation with this insightful article on leveraging AI for graph creation.
The ability to effectively represent and interpret data is crucial for businesses and organizations. Graphs have long been a popular method of visualizing data, providing a clear and concise way to communicate complex information. However, traditional graphing techniques often fall short when faced with large and complex datasets.Enter artificial intelligence (AI). With the power of AI, we can revolutionize the way we create and interpret graphs, taking data representation to a whole new level. In this article, we will explore the intersection of AI and graph creation, the role of AI in enhancing data representation, the process of leveraging AI for graph creation, and the future of data representation with AI.
Understanding the Basics of AI and Graph Creation
Before we dive into the world of AI-driven graph creation, let's first take a moment to understand the basics of AI and how it intersects with data visualization. AI refers to the ability of machines or software to exhibit intelligence and mimic human cognitive processes. It involves the use of algorithms and statistical models to analyze and interpret data, enabling machines to learn and make predictions or decisions.
Artificial Intelligence (AI) has revolutionized various industries by automating processes, optimizing decision-making, and uncovering valuable insights from vast amounts of data. In the realm of data visualization, AI plays a pivotal role in transforming raw data into meaningful visual representations that are easily digestible for users.
The Intersection of AI and Data Visualization
Data visualization is the process of representing data in a visual form, such as graphs or charts, to facilitate understanding and analysis. The marriage of AI and data visualization opens up new possibilities for creating more accurate and insightful graphs.
By leveraging AI techniques such as machine learning and natural language processing, data visualization tools can dynamically adapt to user preferences and provide interactive visualizations that cater to specific needs. This fusion of AI and data visualization empowers users to explore data from multiple perspectives and extract actionable insights efficiently.
Key Components of AI-Driven Graph Creation
When it comes to AI-driven graph creation, there are several key components that play a crucial role in enhancing data representation. These components include data preprocessing, feature extraction, algorithm selection, and model evaluation.
Data preprocessing involves cleaning and transforming raw data to ensure its compatibility with the graph creation process. Feature extraction focuses on identifying relevant patterns and relationships within the data to highlight key insights in the graph. Algorithm selection determines the most suitable AI algorithm to generate visually appealing and informative graphs, while model evaluation assesses the performance and accuracy of the graph creation process.
The Role of AI in Enhancing Data Representation
Now that we have a basic understanding of AI and its connection to data visualization, let's explore how AI transforms traditional graphing techniques and the impact it has on data interpretation.
Artificial Intelligence (AI) has revolutionized the field of data representation by introducing advanced techniques that enhance the way we visualize and analyze information. In addition to automating manual processes, AI has the capability to learn and adapt, making it a powerful tool for transforming traditional graphing techniques.
How AI Transforms Traditional Graphing Techniques
Traditional graphing techniques often rely on manual processes and human intervention. With AI, we can automate many of these processes, reducing human error and saving valuable time. AI algorithms can analyze large datasets at lightning speed, identifying patterns and relationships that may not be immediately apparent to the human eye.
Furthermore, AI can generate interactive visualizations that allow users to explore data in real-time, enabling a more dynamic and engaging experience. By incorporating AI into graph creation, we can unlock new possibilities for data representation and analysis.
The Impact of AI on Data Interpretation
Data interpretation is a crucial step in the graph creation process. AI can assist in this process by providing insights and recommendations based on the patterns and relationships it uncovers. By leveraging AI, we can gain deeper insights from our data, leading to more informed decision-making.
Moreover, AI can help identify outliers and anomalies in the data, flagging potential errors or inconsistencies that may impact the accuracy of the visual representation. This proactive approach to data interpretation ensures that the graphs generated are not only visually appealing but also reliable and trustworthy.
The Process of Leveraging AI for Graph Creation
Now that we understand the role of AI in enhancing data representation, let's explore the steps involved in leveraging AI for graph creation and overcome the challenges that may arise.
When delving into the process of leveraging AI for graph creation, it is essential to recognize the foundational steps that pave the way for successful implementation. The journey typically commences with meticulous data collection and preprocessing, where raw data is transformed and refined to ensure its compatibility with the subsequent stages. This crucial phase sets the groundwork for the accuracy and reliability of the ensuing graph creation process.
Steps to Implement AI in Graph Creation
The process of implementing AI in graph creation begins with data collection and preprocessing. Once the data is prepared, the next step is to extract relevant features. This involves identifying the key attributes that will be used to create the graph. Once the features are extracted, the next step is to select an appropriate algorithm based on the nature of the data and the desired outcome. Finally, the model needs to be evaluated to ensure its effectiveness in representing the data accurately.
Following the extraction of relevant features, the subsequent step involves the meticulous selection of an algorithm that aligns with the unique characteristics of the data at hand and the specific objectives of the graph creation process. This critical decision significantly influences the efficacy and performance of the AI-driven graph creation endeavor. Subsequently, the model undergoes a rigorous evaluation process to ascertain its proficiency in accurately representing the underlying data, thereby validating its utility and effectiveness.
Overcoming Challenges in AI-Driven Graph Creation
While AI-driven graph creation offers immense potential, it also comes with its fair share of challenges. One of the main challenges is the need for high-quality and well-curated data. AI algorithms heavily rely on the quality of the input data, so it is crucial to ensure that the data is clean, relevant, and representative of the problem at hand. Another challenge is the interpretability of AI-generated graphs. As AI algorithms become more complex, it is important to find ways to understand and interpret the results they produce.
Amidst the promising prospects presented by AI-driven graph creation, the landscape is not devoid of obstacles that necessitate adept navigation. A pivotal challenge lies in the prerequisite for high-quality data that is not only comprehensive but also meticulously curated to meet the exacting standards demanded by AI algorithms. The integrity and relevance of the input data play a pivotal role in determining the efficacy and accuracy of the ensuing graph creation process, underscoring the significance of data quality assurance measures.
The Future of Data Representation with AI
As we look to the future, AI holds great promise in advancing data representation and visualization. Let's explore some predicted trends in AI and graph creation as well as the potential of AI in advanced data visualization.
Predicted Trends in AI and Graph Creation
Experts predict that AI will continue to evolve and become more sophisticated in the coming years. This evolution will lead to improved accuracy and efficiency in graph creation, opening up new possibilities for data representation and interpretation.
One of the predicted trends in AI and graph creation is the development of automated graph generation algorithms. These algorithms will be able to analyze large datasets and automatically generate visually appealing and informative graphs. By eliminating the manual process of graph creation, researchers and data analysts will be able to save time and focus on analyzing the data itself.
Another trend that is expected to emerge is the integration of AI with natural language processing (NLP) techniques. This integration will enable users to interact with data visualization tools using natural language queries. For example, users will be able to ask questions like "Show me the trend of sales for the past year" and the AI-powered system will generate the corresponding graph in real-time. This seamless interaction between humans and machines will make data visualization more accessible and intuitive for users of all backgrounds.
The Potential of AI in Advanced Data Visualization
AI has already begun to make waves in the field of advanced data visualization. With AI, we can create dynamic and interactive graphs that adapt to the needs of individual users. This level of personalization and customization is set to revolutionize the way we visualize and interact with data.
Imagine a scenario where you are exploring a dataset and you want to compare the performance of different products over time. With AI-powered data visualization tools, you can simply input the names of the products and the system will automatically generate a comparative graph with all the relevant data. This not only saves time but also allows for a more comprehensive analysis of the data.
Furthermore, AI can help in uncovering hidden patterns and relationships within complex datasets. By applying machine learning algorithms to the data, AI can identify correlations and trends that may not be immediately apparent to human analysts. These insights can then be visualized in a way that is easily understandable, enabling users to make more informed decisions based on the data.
In conclusion, leveraging AI for graph creation allows us to enhance our data representation and gain deeper insights from our data. By understanding the basics of AI and its intersection with data visualization, we can harness the power of AI to transform traditional graphing techniques. Through the process of leveraging AI for graph creation, we can overcome challenges and pave the way for the future of data representation with AI. The potential of AI in advanced data visualization is vast, and as the technology continues to evolve, we can expect even more exciting developments in the field. So, embrace AI, enhance your data representation, and unlock the full potential of your data.
Ready to transform your data visualization and unlock the full potential of your data? CastorDoc is here to elevate your business intelligence with our cutting-edge AI Agent for Analytics. Experience the power of self-service analytics and gain trustworthy, instantaneous data answers to drive your strategic decisions. Say goodbye to data literacy barriers and maximize your data stack's ROI with CastorDoc. Empower your business teams with the autonomy and trust they need to access, understand, and utilize data effectively. Reduce the burden on your data teams and analysts, and start making more informed decisions today. Try CastorDoc today and witness the revolution in data representation and analytics.
You might also like
Get in Touch to Learn More
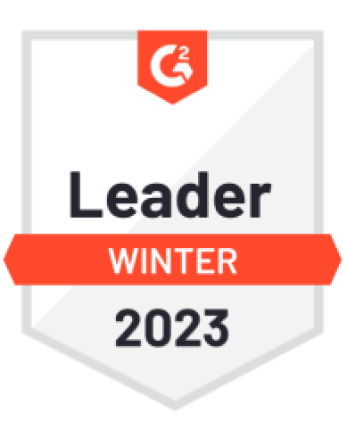
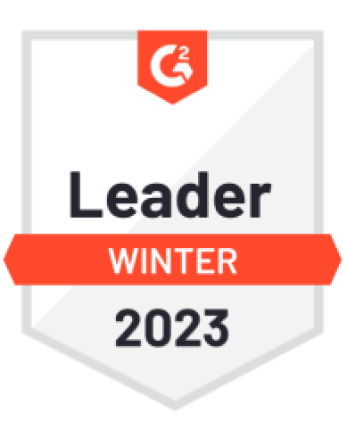

“[I like] The easy to use interface and the speed of finding the relevant assets that you're looking for in your database. I also really enjoy the score given to each table, [which] lets you prioritize the results of your queries by how often certain data is used.” - Michal P., Head of Data