Unlocking the Power of Augmented Analytics: A Comprehensive Guide
Discover the transformative potential of augmented analytics with our comprehensive guide.
In this article, we will demystify augmented analytics, explore its evolution, and contrast it with traditional business intelligence. We will also unpack its essential features and services, address common queries, and discuss who can benefit from it. Lastly, we will provide strategies for navigating the analytical landscape and implementing augmented analytics. So, let's get started!
Demystifying Augmented Analytics
Augmented analytics has emerged as a transformative approach to data analysis. At its core, augmented analytics leverages artificial intelligence (AI) and machine learning (ML) technologies to automate data preparation, visualization, and insight generation. By augmenting the abilities of human analysts, augmented analytics streamlines the decision-making process and empowers organizations to extract maximum value from their data.
Understanding the Basics of Augmented Analytics
Augmented analytics combines AI and ML algorithms with human expertise to uncover hidden patterns, relationships, and insights within vast datasets. It automates time-consuming tasks, such as data cleansing, merging, and visualization, allowing analysts to focus on higher-value activities like interpreting results and making strategic recommendations.
But how exactly does augmented analytics achieve this level of automation and efficiency? It starts with natural language processing (NLP) and machine learning algorithms that enable augmented analytics platforms to understand user queries and generate relevant insights in real time. This means that business users, regardless of their technical skills, can access and analyze data intuitively, democratizing the analytics process across organizations.
Exploring the Evolution of Analytics
Traditional analytics approaches have relied heavily on manual data preparation and analysis, resulting in inefficiencies and limitations. Augmented analytics represents a significant shift, as it automates repetitive tasks and empowers analysts to make data-driven decisions faster and more accurately.
But the impact of augmented analytics goes beyond just improving efficiency. With the massive growth of data and the increasing demand for actionable insights, augmented analytics is poised to revolutionize the analytics landscape. It eliminates the barriers to entry for non-technical users and enables organizations of all sizes and industries to derive valuable insights from their data.
Imagine a small retail business that wants to understand customer preferences and optimize their product offerings. In the past, they would have to rely on manual analysis, which could be time-consuming and prone to human error. With augmented analytics, they can leverage AI and ML algorithms to automatically analyze customer data, identify trends, and make data-driven decisions. This not only saves time and resources but also allows the business to stay competitive in a rapidly changing market.
Furthermore, augmented analytics has the potential to uncover insights that may have been overlooked by human analysts alone. By leveraging AI and ML technologies, augmented analytics can process vast amounts of data at a speed and scale that would be impossible for humans to achieve. This opens up new possibilities for discovering hidden patterns and correlations, leading to more accurate predictions and actionable insights.
In conclusion, augmented analytics is not just a buzzword; it is a game-changer in the world of data analysis. By combining AI and ML technologies with human expertise, augmented analytics automates repetitive tasks, empowers analysts, and enables organizations to unlock the full potential of their data. As the analytics landscape continues to evolve, augmented analytics will undoubtedly play a crucial role in driving data-driven decision-making and innovation.
Contrasting Augmented Analytics with Business Intelligence
While both augmented analytics and traditional business intelligence (BI) aim to provide insights, they differ significantly in their approaches. While BI relies heavily on manual data modeling and report creation, augmented analytics automates these processes, allowing for faster and more agile decision-making. Let's delve deeper into the key differences between augmented analytics and traditional BI.
Differentiating Between Augmented Analytics and Traditional BI
In traditional BI, analysts often spend a significant amount of time preparing and cleansing data before they can analyze it. Augmented analytics, on the other hand, takes a different approach by leveraging the power of an AI co-pilot powered by a comprehensive data catalog. This innovative solution focuses on surfacing the most relevant insights from the vast amount of data that already exists within an organization, but is often hard to find or access.
With augmented analytics, the AI co-pilot intelligently navigates through the data catalog, identifying and extracting the most pertinent information based on the user's specific needs. This approach eliminates the need to create new reports or dashboards in most cases, as the required insights are already present within the existing data. In fact, studies show that 90% of the time, organizations do not need to generate new content, as the answers they seek are hidden within their current data assets.
Imagine a scenario where a business analyst is tasked with analyzing sales data to identify trends and opportunities. In the traditional BI approach, the analyst would have to manually sift through multiple data sources, cleanse and integrate the data, and create new visualizations to derive insights. This process is time-consuming and often leads to delays in decision-making.
However, with augmented analytics powered by an AI co-pilot and a robust data catalog, the analyst can simply input their query, and the system will automatically surface the most relevant insights. The AI co-pilot understands the context of the request, scans the catalog for related data points, and presents the findings in an easily digestible format. This not only saves time but also ensures that the analyst is working with the most accurate and up-to-date information.
Furthermore, augmented analytics goes beyond simply presenting data by providing contextual recommendations and actionable insights. By leveraging advanced machine learning algorithms, the AI co-pilot can identify patterns, trends, and anomalies that may have otherwise been overlooked. This deeper understanding of the data enables organizations to make more informed decisions and drive better business outcomes.
For example, let's say a retail company wants to optimize its product placement strategy. With traditional BI, the analyst would have to manually analyze sales data, customer behavior, and store layout information to identify potential improvements. However, with augmented analytics, the AI co-pilot can automatically correlate these data points, identify high-performing product combinations, and recommend optimal shelf placement strategies. This level of granular insight empowers the organization to make data-driven decisions that directly impact revenue growth.
The Future of Data Analysis: Augmented Analytics
As organizations embrace augmented analytics, they position themselves for a data-driven future. The ability to leverage AI-powered insights to make informed decisions will separate successful organizations from the competition.
Augmented analytics improves efficiency and accuracy by automating routine tasks and augmenting human intelligence. Instead of spending valuable time on data preparation, analysts can focus on interpreting results, exploring alternative scenarios, and formulating strategies based on accurate insights.
Moreover, the future of augmented analytics holds even more promise. With advancements in natural language processing (NLP), users will be able to interact with data using plain language, making data analysis accessible to a wider audience. Imagine a scenario where a marketing manager can simply ask, "What are the top-selling products in the past month?" and receive instant, actionable insights. This democratization of data analysis will empower decision-makers at all levels of the organization, driving innovation and growth.
In conclusion, while traditional BI has been the go-to approach for data analysis, augmented analytics is revolutionizing the field by automating processes, providing contextual insights, and empowering users with AI-powered recommendations. As organizations embrace this data-driven future, they will unlock new opportunities and gain a competitive edge in the ever-evolving business landscape.
Unpacking the Essential Features and Services
Now that we have a better understanding of augmented analytics, let's dive into its key components and how they enhance decision-making processes.
Augmented analytics is a cutting-edge technology that leverages machine learning and natural language processing to automate data analysis and generate actionable insights. By combining the power of artificial intelligence with human expertise, augmented analytics empowers organizations to make data-driven decisions quickly and effectively.
Key Components of Augmented Analytics Solutions
Augmented analytics solutions typically consist of three primary components: data integration and preparation, automated insight generation, and augmented data discovery. These components work together to automate the entire analytics workflow – from data ingestion to actionable insights.
Data integration and preparation involve collecting and blending datasets from various sources. Augmented analytics platforms employ advanced algorithms to cleanse, standardize, and harmonize the data, ensuring accuracy and consistency.
Automated insight generation is another critical component of augmented analytics solutions. By analyzing data patterns and trends, machine learning algorithms can identify correlations and anomalies that human analysts may overlook. This automated process accelerates the generation of insights, enabling organizations to respond to changing market conditions swiftly.
Ready to harness the transformative power of augmented analytics in your organization? CastorDoc is your ideal partner, blending advanced governance features with a user-friendly AI assistant to elevate your self-service analytics capabilities. With CastorDoc, you can streamline data cataloging, ensure compliance, and enhance data quality with ease. Plus, our intuitive AI copilot allows for seamless natural language interactions, making data accessible to all users, regardless of technical expertise. Don't miss the opportunity to empower your data teams and business users alike. Try CastorDoc today and unlock the full potential of your data for smarter, faster decision-making.
You might also like
Get in Touch to Learn More
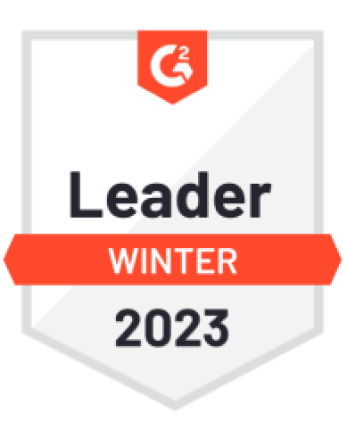
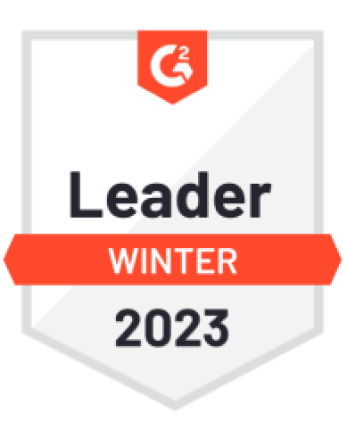

“[I like] The easy to use interface and the speed of finding the relevant assets that you're looking for in your database. I also really enjoy the score given to each table, [which] lets you prioritize the results of your queries by how often certain data is used.” - Michal P., Head of Data