Visual Data Exploration Defined: Leveraging Data Visualization for Effective Analysis
Explore the power of visual data exploration
In today's data-driven world, the ability to effectively analyze and interpret large amounts of data is paramount. Data visualization, particularly through the concept of visual data exploration, has emerged as a powerful tool in this process. Visual data exploration involves the use of various techniques and tools to visually represent and interact with data, enabling analysts to gain valuable insights and make informed decisions.
Understanding Visual Data Exploration
Visual data exploration is the process of using graphical representations to explore and understand complex data sets. It goes beyond traditional data analysis methods by leveraging the power of visuals to uncover patterns, trends, and relationships that may not be readily apparent in raw data. By translating data into visual forms, analysts can enhance their understanding and identify meaningful insights more efficiently.
The Concept of Visual Data Exploration
Visual data exploration relies on the idea that humans are highly visual beings. We are naturally wired to process and interpret visual information more effectively than numbers or text alone. By transforming data into visual representations such as charts, graphs, and maps, we can tap into our innate visual processing abilities to uncover patterns, outliers, and correlations that might be missed when working with rows and columns of data.
Moreover, visual data exploration is not just about creating static visuals; it also involves interactive visualizations that allow users to manipulate and drill down into the data dynamically. This interactivity enables analysts to explore data from different angles, zoom in on specific data points, and filter out noise to focus on the most relevant information. By providing a more engaging and dynamic data exploration experience, interactive visuals enhance the analytical process and empower users to make data-driven decisions with confidence.
Importance of Visual Data Exploration in Data Analysis
Visual data exploration plays a crucial role in data analysis by enabling analysts to quickly identify key insights and derive actionable conclusions. It allows for the exploration and comparison of large data sets in a visually intuitive way, reducing the cognitive load and facilitating quicker decision-making. By presenting data visually, analysts can communicate complex information more effectively and engage stakeholders in a more meaningful way.
Furthermore, visual data exploration is not limited to just business applications; it also has significant implications in fields such as scientific research, healthcare, and urban planning. In scientific research, visualizing complex data sets can help researchers identify trends and patterns that lead to new discoveries. In healthcare, visual data exploration can aid in identifying correlations between patient data and treatment outcomes, leading to improved healthcare delivery. In urban planning, visualizing demographic and geographic data can inform decision-making processes related to infrastructure development and resource allocation, ultimately leading to more sustainable and inclusive urban environments.
The Intersection of Data Visualization and Analysis
Data visualization and analysis are two closely intertwined disciplines that complement each other. While data analysis focuses on extracting meaning from data, data visualization enhances the analysis process by presenting data in a visual format that is easier to understand and interpret.
Understanding the intersection of data visualization and analysis is crucial in today's data-driven world. Data visualization not only aids in making sense of complex data but also helps in identifying trends, patterns, and outliers that may not be apparent through traditional analysis methods. By visually representing data, analysts can uncover hidden insights and make informed decisions based on a comprehensive understanding of the data.
Role of Data Visualization in Analysis
Data visualization plays a crucial role in analysis by providing a visual framework that allows analysts to explore data, discover patterns, and communicate findings. It enables analysts to identify outliers, trends, and relationships within data sets, leading to a deeper understanding of the underlying phenomena.
Moreover, data visualization serves as a powerful tool for storytelling. By creating compelling visual narratives, analysts can effectively convey the significance of their findings to a wider audience. Through interactive charts, graphs, and dashboards, complex data can be simplified and presented in a way that resonates with viewers, facilitating better decision-making and driving actionable insights.
Advantages of Integrating Data Visualization in Analysis
Integrating data visualization in the analysis process offers several advantages. Firstly, it encourages exploratory analysis, allowing analysts to ask questions, test hypotheses, and gain new insights on the fly. Additionally, it promotes effective communication by presenting complex information in a visually appealing manner, making it easier for stakeholders to grasp and act upon.
Furthermore, data visualization enhances collaboration among team members by providing a common visual language for discussing and interpreting data. By fostering a data-driven culture within organizations, data visualization promotes transparency, accountability, and innovation, ultimately leading to improved decision-making processes and business outcomes.
Key Elements of Visual Data Exploration
Visual data exploration involves a combination of techniques and tools to effectively analyze and interact with data. Below are two key elements that form the foundation of visual data exploration:
Data Representation Techniques
Data representation techniques refer to the various graphical methods used to present data visually. These can include charts, graphs, heatmaps, scatter plots, and more. The choice of data representation technique depends on the nature of the data being analyzed and the insights the analyst aims to derive.
Charts are commonly used to represent data in a visual format, allowing for easy comparison and trend analysis. Graphs, on the other hand, are useful for showing relationships between different variables. Heatmaps provide a color-coded representation of data values, making it easier to identify patterns and anomalies. Scatter plots are effective in displaying the correlation between two variables, helping analysts identify trends and outliers.
Interactive Exploration Tools
Interactive exploration tools enable analysts to interact with visualizations and manipulate data in real-time. These tools allow for zooming, filtering, brushing, and other interactive techniques to gain different perspectives and dig deeper into the data. By providing interactive capabilities, analysts can uncover hidden insights and answer ad-hoc questions more effectively.
Zooming functionality allows analysts to focus on specific data points or sections of a visualization for a closer inspection. Filtering tools enable the selection of specific data subsets for analysis, helping to isolate trends or outliers. Brushing techniques allow for the highlighting of data points of interest, making it easier to compare different parts of the dataset. These interactive exploration tools empower analysts to explore data dynamically and extract meaningful insights efficiently.
Challenges in Visual Data Exploration
While visual data exploration offers powerful capabilities, it also comes with its fair share of challenges. Below are two key challenges that analysts often encounter:
Dealing with Large Data Sets
One of the primary challenges is dealing with large data sets. As data continues to grow exponentially, it becomes increasingly difficult to visualize and explore massive amounts of information effectively. Analysts must employ strategies like data sampling, aggregation, and scalable visualization techniques to navigate and make sense of large data sets.
Moreover, the sheer volume of data can overwhelm traditional visualization tools, leading to performance issues and hindering the exploration process. To address this challenge, analysts are turning to advanced technologies such as parallel processing and distributed computing to handle and analyze vast data sets efficiently.
Ensuring Accuracy and Precision
Another challenge lies in ensuring the accuracy and precision of visualizations. Incorrect visual representation or misleading graphics can lead to erroneous conclusions and flawed decision-making. Analysts need to understand the limitations of visualization techniques and ensure they accurately depict the underlying data to maintain the integrity of the analysis.
Furthermore, achieving accuracy in visual data exploration requires a deep understanding of the data sources, potential biases, and the context in which the visualizations will be interpreted. Analysts must critically evaluate the data quality and relevance to avoid misinterpretations that could impact the validity of their findings.
Future Trends in Visual Data Exploration
As the field of data visualization continues to evolve, several trends are shaping the future of visual data exploration:
Predicted Developments in Data Visualization
Advancements in technologies like virtual reality (VR), augmented reality (AR), and mixed reality (MR) are expected to revolutionize data visualization. These immersive experiences will enable analysts to explore data in three-dimensional spaces, providing new perspectives and insights.
Imagine being able to step into a virtual world where data points float around you, allowing you to physically interact with them and gain a deeper understanding of complex datasets. With VR, analysts can immerse themselves in the data, uncovering hidden patterns and relationships that traditional two-dimensional visualizations might miss.
Furthermore, the combination of AR and MR with data visualization opens up possibilities for real-time data overlays onto the physical world. Analysts could walk through a factory floor, for example, and see real-time performance metrics superimposed on machines, enabling quick decision-making and troubleshooting.
The Impact of AI and Machine Learning on Visual Data Exploration
AI and machine learning will play a significant role in automating certain aspects of visual data exploration. Machine learning algorithms can assist in data cleaning, pattern recognition, and predictive analytics, allowing analysts to focus more on interpreting results and making informed decisions.
Imagine a scenario where AI algorithms analyze vast amounts of data, identify trends, and automatically generate visualizations that highlight key insights. Analysts can then fine-tune these visualizations to extract even more value, combining the power of machine intelligence with human expertise.
In conclusion, visual data exploration is a powerful approach that leverages data visualization techniques to enhance the analysis process. By presenting data visually, analysts can quickly identify patterns, trends, and relationships that may not be readily apparent in raw data. With the integration of interactive exploration tools and advancements in technology, visual data exploration will continue to evolve and empower analysts to make more informed decisions based on comprehensive, accessible, and visually appealing insights.
Ready to transform your data analysis and decision-making processes? CastorDoc is here to elevate your visual data exploration journey. As the most reliable AI Agent for Analytics, CastorDoc empowers your business teams to harness instantaneous, trustworthy data answers for strategic challenges. Experience the power of self-service analytics, overcome data literacy barriers, and maximize your data stack's ROI. Activate your data's full potential and give your business users the autonomy and trust to make informed decisions with ease. Try CastorDoc today and start leveraging your data like never before.
You might also like
Get in Touch to Learn More
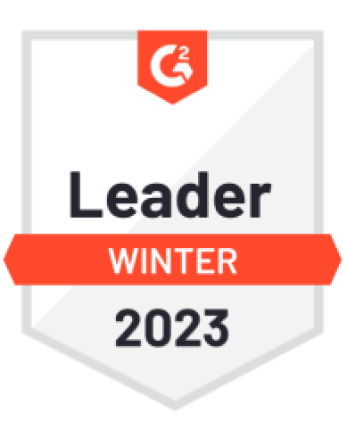
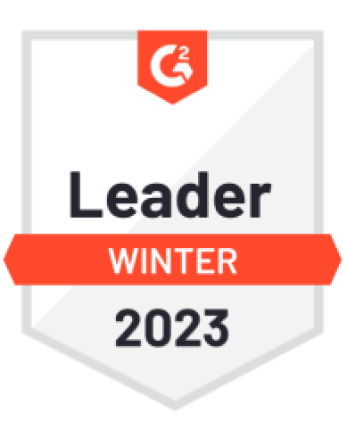

“[I like] The easy to use interface and the speed of finding the relevant assets that you're looking for in your database. I also really enjoy the score given to each table, [which] lets you prioritize the results of your queries by how often certain data is used.” - Michal P., Head of Data