7 Data Integrity Best Practices You Need to Know
Discover the 7 essential data integrity best practices that will safeguard your organization's information and ensure accuracy, reliability, and security.
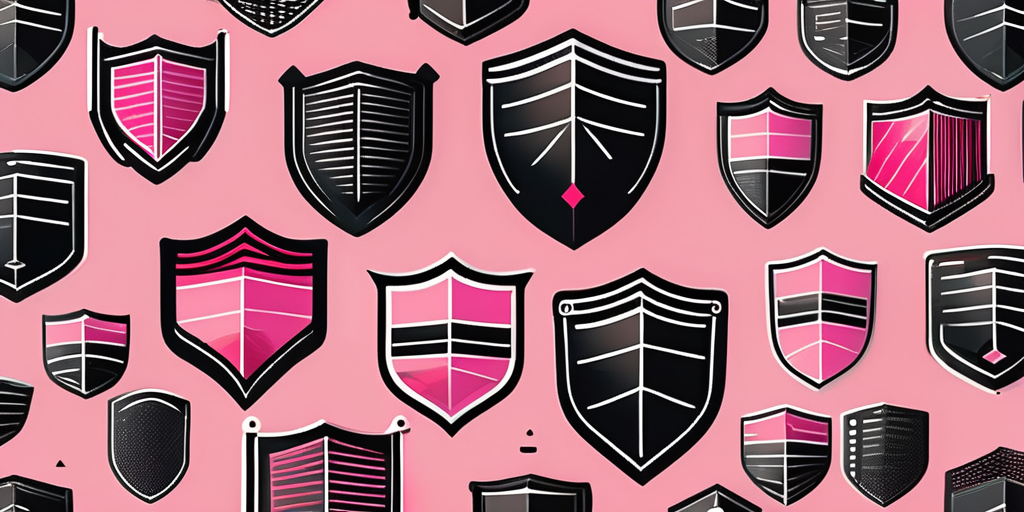
Data integrity is a critical aspect of any data-driven organization. Ensuring the accuracy, consistency, and reliability of data is essential for making informed decisions and maintaining trust with stakeholders. In this article, we will explore seven data integrity best practices that every organization should implement.
Understanding Data Integrity
Data integrity refers to the accuracy, completeness, and reliability of data throughout its lifecycle. It involves the proper handling, storage, and protection of data to prevent unauthorized access, corruption, or loss. By maintaining data integrity, organizations can trust that their data is reliable and can be used confidently for decision-making.
What is Data Integrity?
Data integrity encompasses several aspects, including data accuracy, consistency, and reliability. Accuracy ensures that data is correct and free from errors, while consistency refers to data being uniform and matching across different systems or databases. Reliability implies that data is trustworthy and reflects the true state of affairs.
Why is Data Integrity Important?
Data integrity is crucial to organizations for several reasons. First and foremost, it helps maintain the trust of stakeholders, including customers, partners, and regulators. By ensuring the accuracy and reliability of data, organizations can build strong relationships and avoid reputational damage caused by data errors or breaches.
Furthermore, data integrity enables organizations to make informed decisions based on reliable information. Managers and executives rely on accurate and consistent data to analyze trends, identify opportunities, and mitigate risks. Without data integrity, organizations may face costly errors and flawed decision-making processes.
Lastly, data integrity is vital for compliance with legal and regulatory requirements. Many industries, such as healthcare and finance, have strict regulations regarding data privacy and security. Adhering to these regulations demonstrates a commitment to protecting sensitive information and reduces the risk of legal consequences.
Ensuring data integrity involves implementing robust data management practices. Organizations must establish data governance frameworks that define roles, responsibilities, and processes for data handling. This includes implementing data quality controls, such as data validation and verification procedures, to identify and rectify any inaccuracies or inconsistencies in the data.
Moreover, organizations need to invest in secure data storage and backup systems to protect against data loss or corruption. This may involve implementing encryption techniques, access controls, and regular data backups to ensure data availability and prevent unauthorized access or tampering.
In addition to technical measures, organizations must also focus on creating a culture of data integrity. This involves training employees on data handling best practices, emphasizing the importance of data accuracy and reliability, and promoting a proactive approach to data security. By instilling a sense of responsibility and accountability among employees, organizations can further strengthen their data integrity efforts.
The Role of Data Governance in Ensuring Data Integrity
Data governance plays a significant role in maintaining data integrity within an organization. It provides a framework for establishing policies, procedures, and responsibilities related to data management. By implementing effective data governance practices, organizations can proactively address data integrity issues and ensure data quality.
Furthermore, data governance helps organizations comply with regulations such as GDPR and HIPAA, which require strict data integrity measures to protect sensitive information. By having a robust data governance framework in place, organizations can demonstrate accountability and transparency in their data handling processes.
Defining Data Governance
Data governance refers to the overall management and oversight of data assets within an organization. It involves defining data ownership, establishing data quality standards, and implementing processes for data integration, access, and security. Data governance provides the structure and guidelines necessary to maintain data integrity.
In addition, data governance helps organizations align their data management practices with business objectives, ensuring that data is used effectively to drive decision-making and innovation. By integrating data governance into their strategic planning, organizations can leverage their data assets more efficiently and gain a competitive edge in the market.
Data Governance and Data Integrity
Data governance directly supports data integrity by establishing procedures for data validation, verification, and quality control. It ensures that data is accurate, consistent, and reliable throughout its lifecycle. By assigning clear responsibilities and implementing data governance practices, organizations can prevent data integrity issues and address any potential problems promptly.
Moreover, data governance promotes a culture of data stewardship within an organization, where employees are empowered to take ownership of data quality and accuracy. This collaborative approach fosters a data-driven mindset across departments, leading to improved data integrity and organizational performance.
Best Practice 1: Implementing Data Validation
Data validation is an essential practice for ensuring data integrity. It involves the verification of data accuracy and completeness against predefined criteria. By implementing robust data validation processes, organizations can identify and correct data errors and discrepancies before they impact decision-making or business operations.
The Importance of Data Validation
Data validation helps identify and prevent errors or inconsistencies in data. It ensures that data entered into systems or databases meets predefined standards and requirements. By validating data, organizations can minimize the risk of data integrity issues and maintain the quality and reliability of their data assets.
Steps to Implement Data Validation
- Define data validation rules: Organizations should establish clear validation rules and criteria to ensure data accuracy and consistency. These rules can include format checks, range restrictions, or logical validations.
- Automate data validation processes: Implementing automated data validation tools or scripts can help streamline and standardize the validation process. Automation enables organizations to validate large volumes of data efficiently and identify potential issues promptly.
- Regularly monitor and review data validation results: Organizations should continuously monitor data validation results to identify trends, patterns, or recurring errors. Regular reviews help identify areas for improvement and enable organizations to take corrective actions to maintain data integrity.
Best Practice 2: Regular Data Audits
Regular data audits are another crucial best practice for maintaining data integrity. Data audits involve the systematic examination and evaluation of data sources, processes, and controls to ensure their compliance with data integrity guidelines. By conducting data audits, organizations can identify data integrity risks and take corrective actions promptly.
The Purpose of Data Audits
Data audits help organizations assess the overall health and quality of their data assets. They provide insights into data integrity risks, weaknesses in data management processes, and areas that require improvement. By conducting regular data audits, organizations can proactively identify and address data integrity issues before they escalate.
Conducting Effective Data Audits
- Define audit objectives: Clearly define the objectives of the data audit, including the areas and processes to be audited. This helps focus the audit efforts and ensure that all critical areas related to data integrity are covered.
- Perform a comprehensive data analysis: Analyze data from various sources, including databases, file systems, and data warehouses. Evaluate data quality, accuracy, consistency, and completeness. Identify any discrepancies, anomalies, or outliers that may indicate data integrity issues.
- Assess data governance practices: Evaluate the effectiveness of data governance processes, including data quality controls, data management procedures, and security measures. Assess the roles and responsibilities of data stewards and ensure that data governance policies align with data integrity objectives.
- Develop action plans: Based on the audit findings, develop action plans to address identified data integrity issues. Assign responsibilities and establish timelines for implementing corrective actions. Regularly monitor progress to ensure the effectiveness of the action plans.
Best Practice 3: Establishing Data Standards
Establishing data standards is a fundamental best practice for maintaining data integrity. Data standards define the format, structure, and quality requirements for data across the organization. By defining and enforcing data standards, organizations can ensure consistency and reliability in data management processes.
The Need for Data Standards
Data standards provide a common framework for data management and integration. They help organizations prevent data inconsistencies, reduce errors, and improve data quality. By establishing data standards, organizations can facilitate data sharing, streamline business processes, and support better decision-making.
Creating and Enforcing Data Standards
To establish data standards, organizations should follow these steps:
- Define data standards: Clearly define and document the required data standards, including format, naming conventions, and data quality requirements. Involve relevant stakeholders, including data owners, users, and subject matter experts, in the standardization process.
- Communicate data standards: Ensure that all employees understand and follow the established data standards. Provide training and educational materials to promote awareness and compliance with data standards. Regularly communicate any updates or changes to the data standards.
- Implement data quality controls: Develop measures to monitor and enforce data standards. Implement data quality checks, automated validations, and data profiling techniques to identify deviations from the established data standards. Take corrective actions whenever deviations are identified.
- Maintain data standards: Regularly review and update data standards to ensure their relevance and alignment with organizational goals and evolving industry requirements. Continuously monitor the effectiveness of data standards and make necessary adjustments based on feedback and changing business needs.
Conclusion
Implementing effective data integrity best practices is essential for organizations to ensure the accuracy, consistency, and reliability of their data assets. By understanding the importance of data integrity, the role of data governance, and adopting practices such as data validation, regular data audits, and establishing data standards, organizations can safeguard their data and make data-driven decisions with confidence.
By prioritizing data integrity, organizations can gain a competitive edge, build trust with stakeholders, and achieve better business outcomes in today's data-driven landscape.
You might also like
Get in Touch to Learn More
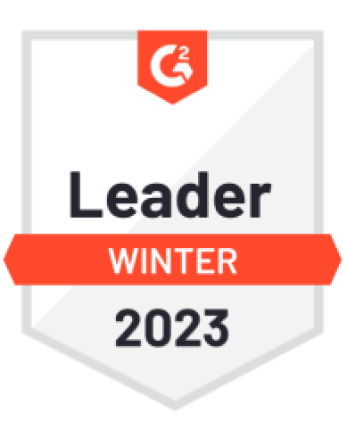
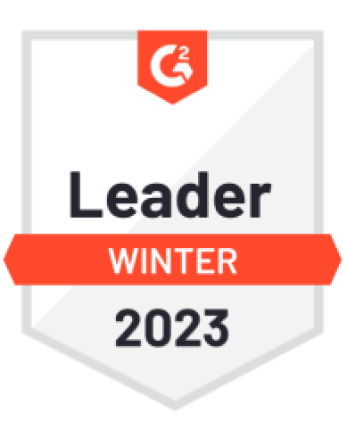

“[I like] The easy to use interface and the speed of finding the relevant assets that you're looking for in your database. I also really enjoy the score given to each table, [which] lets you prioritize the results of your queries by how often certain data is used.” - Michal P., Head of Data