Data Integrity vs Data Quality: Why They Aren’t the Same
Discover the crucial distinctions between data integrity and data quality in this insightful article.

Today, data has become the lifeblood of organizations. It drives decision-making, enhances operational efficiency, and fuels growth. However, not all data is created equal. Two critical aspects that businesses need to consider are data integrity and data quality. While these terms may often be used interchangeably, they are not the same. Understanding their differences is crucial for organizations aiming to optimize their data management processes. In this article, we will explore the nuances between data integrity and data quality, their importance in business, the impact of poor data, and strategies for ensuring both data integrity and data quality.
Understanding the Basics of Data
What is Data Integrity?
Data integrity refers to the accuracy, consistency, and completeness of data throughout its lifecycle. It ensures that data remains intact and unaltered, maintaining its reliability and trustworthiness. Data integrity encompasses various dimensions such as accuracy, validity, and reliability, which are vital for making informed decisions.
Ensuring data integrity involves implementing measures to prevent unauthorized access, data corruption, or loss. It also includes validation processes to check for errors or inconsistencies in the data. By maintaining data integrity, organizations can enhance data security, build customer trust, and comply with regulatory requirements.
What is Data Quality?
Data quality, on the other hand, focuses on the fitness for use of data. It assesses the level of usefulness and relevance of data for specific purposes. Data quality encompasses several attributes, including accuracy, completeness, consistency, and timeliness, all of which determine the overall value of data.
Improving data quality involves data profiling to understand the structure and content of datasets, data cleansing to remove errors and inconsistencies, and data enrichment to enhance the value of existing data. Organizations rely on high-quality data to drive business decisions, improve operational efficiency, and gain a competitive edge in the market.
The Importance of Data Integrity and Data Quality
Role of Data Integrity in Business
Effective data integrity ensures that data remains uncorrupted and reliable, enabling organizations to trust and rely on the information at hand. Maintaining data integrity is crucial for regulatory compliance, as inaccurate or compromised data can lead to severe legal and financial repercussions. Moreover, data integrity supports data-driven decision-making processes, providing a solid foundation for strategic planning and operational efficiency.
Ensuring data integrity involves implementing robust data validation processes, encryption methods, and access controls. By establishing data integrity measures, organizations can safeguard against data breaches, unauthorized modifications, and data loss. This not only protects sensitive information but also enhances the overall credibility and trustworthiness of the organization in the eyes of stakeholders and customers.
Role of Data Quality in Business
Data quality is paramount for organizations aiming to extract actionable insights and derive accurate conclusions from their data. High-quality data ensures that analysis and reporting are based on reliable information, enabling businesses to identify trends, patterns, and anomalies with confidence. Data quality is vital for customer relationship management, market analysis, and overall business performance evaluation.
Improving data quality involves data cleansing, normalization, and deduplication processes to eliminate inconsistencies, errors, and redundancies. By enhancing data quality, organizations can streamline operations, enhance decision-making processes, and gain a competitive edge in the market. High-quality data also fosters innovation and enables organizations to adapt quickly to changing market dynamics and customer preferences.
Distinguishing Between Data Integrity and Data Quality
When delving into the realm of data management, it is crucial to understand the nuanced differences between data integrity and data quality. While data integrity primarily focuses on maintaining the accuracy and reliability of data, ensuring that it remains unaltered and consistent throughout its lifecycle, data quality takes a more comprehensive approach. Data quality not only encompasses the aspect of accuracy but also delves into assessing the fitness for use of the data. This means evaluating whether the data is complete, consistent, valid, relevant, and ultimately useful for its intended purpose.
Expanding on the concept of data integrity as a subset of data quality, it is essential to highlight that data integrity plays a foundational role in upholding the overall quality of data. By preventing data corruption and maintaining the integrity of data, organizations lay a solid groundwork for ensuring data quality. However, it is crucial to recognize that data quality extends beyond just integrity. It involves a multifaceted approach that includes dimensions such as relevance and usefulness, which require robust data management strategies to be fully realized.
Key Differences
While data integrity focuses on maintaining the accuracy and reliability of data, data quality goes beyond by assessing the fitness for use. Data integrity is largely concerned with preventing data corruption, while data quality aims to ensure data completeness, consistency, and validity. In essence, data integrity is a subset of data quality, emphasizing the preservation of the data's integrity throughout its lifecycle.
Common Misconceptions
It is imperative to debunk a common misconception that data integrity alone guarantees data quality. While data integrity is a fundamental aspect, it is not sufficient to ensure the overall quality of data. Data quality incorporates additional dimensions such as relevance and usefulness, which require comprehensive data management strategies beyond preservation alone. By recognizing and addressing this misconception, organizations can embrace a holistic approach towards data management.
Impact of Poor Data Integrity and Data Quality
When it comes to data management, maintaining high levels of data integrity and data quality is paramount for organizations across various industries. Data integrity refers to the accuracy, consistency, and reliability of data throughout its lifecycle. On the other hand, data quality encompasses the completeness, validity, and relevance of data for its intended use. Both aspects are crucial for ensuring that organizations make informed decisions, drive innovation, and maintain a competitive edge in the market.
Consequences of Low Data Integrity
Low data integrity can have far-reaching consequences that extend beyond the immediate operational challenges. Inaccurate or corrupted data not only erodes trust within an organization but also impacts external stakeholders such as customers, partners, and regulatory bodies. The ripple effects of compromised data integrity can lead to costly compliance violations, legal repercussions, and erosion of brand reputation. Moreover, the lack of data integrity can introduce inefficiencies in processes, increase the likelihood of errors, and impede the seamless flow of information across departments.
Consequences of Poor Data Quality
Similarly, poor data quality can act as a significant roadblock for organizations striving to extract meaningful insights from their data assets. Data that is incomplete, inconsistent, or outdated can skew analytical results, misrepresent performance metrics, and distort strategic planning efforts. This can have a direct impact on customer relationships, as inaccurate data may lead to misguided marketing campaigns, subpar customer service experiences, and diminished loyalty. Furthermore, the reliance on poor data quality can hinder organizations from identifying emerging market trends, anticipating customer needs, and adapting to dynamic business environments.
Strategies for Ensuring Data Integrity and Data Quality
Best Practices for Data Integrity
Organizations can adopt several best practices to maintain data integrity. Implementing data validation procedures, encryption mechanisms, and role-based access controls helps prevent data corruption and unauthorized modifications. Regular data backups, audit trails, and data provenance tracking contribute to the preservation of data integrity. Adopting data governance frameworks and data quality standards also ensures accountability and adherence to data integrity principles.
Moreover, it is essential for organizations to conduct regular data integrity checks and assessments to identify any potential vulnerabilities or weaknesses in their data management processes. By conducting thorough data integrity testing, organizations can proactively address any issues that may compromise the accuracy and reliability of their data. This proactive approach not only enhances data integrity but also instills confidence in stakeholders regarding the trustworthiness of the organization's data assets.
Best Practices for Data Quality
To enhance data quality, organizations should establish robust data cleansing processes, addressing inconsistencies and inaccuracies. Employing data profiling techniques and data quality tools can help identify and rectify data anomalies. Establishing data quality metrics, data quality audits, and data quality improvement plans promotes a proactive approach toward data quality management. Additionally, ensuring data quality controls across data sources, data integration, and data transformation processes mitigates the risk of data degradation.
Furthermore, organizations can leverage advanced technologies such as artificial intelligence and machine learning algorithms to automate data quality checks and validations. By incorporating these cutting-edge tools into their data quality management processes, organizations can achieve higher levels of accuracy and efficiency in maintaining data quality standards. This technological integration not only streamlines data quality processes but also enables organizations to adapt to evolving data challenges in a rapidly changing digital landscape.
Conclusion
In summary, data integrity and data quality are distinct yet interconnected concepts that play a pivotal role in modern organizations. While data integrity safeguards the accuracy and reliability of data, data quality assesses its fitness for use. Organizations must recognize their unique contributions and implement strategies to address both aspects. By prioritizing data integrity and data quality, businesses can optimize decision-making, drive operational excellence, and gain a competitive edge in today's data-driven landscape.
You might also like
Get in Touch to Learn More
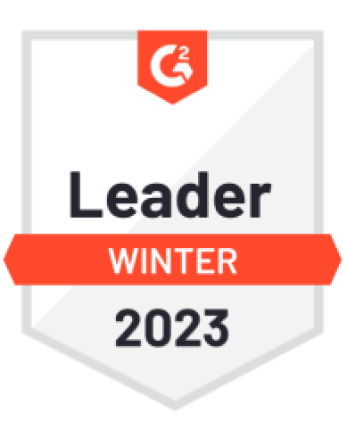
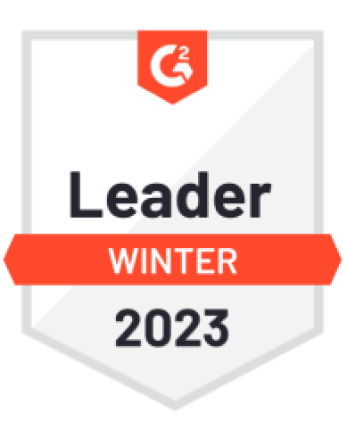

“[I like] The easy to use interface and the speed of finding the relevant assets that you're looking for in your database. I also really enjoy the score given to each table, [which] lets you prioritize the results of your queries by how often certain data is used.” - Michal P., Head of Data