Data Lake vs Data Swamp: Differences & Cautionary Steps
Uncover the key distinctions between a data lake and a data swamp in this insightful article.
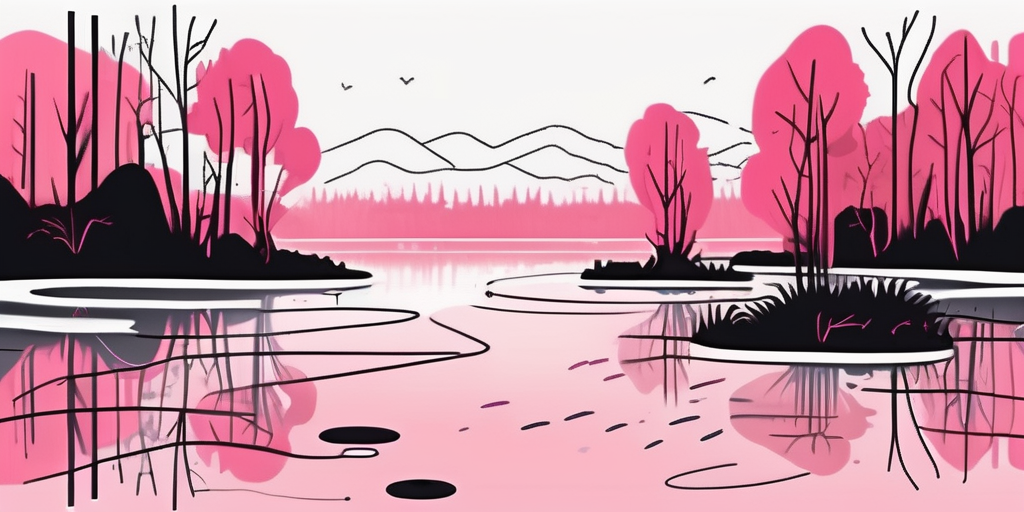
In the world of data management, two concepts that are often discussed are data lakes and data swamps. While they may sound similar, they are fundamentally different in terms of organization, accessibility, and data quality. In this article, we will explore the basics of data lakes and data swamps, analyze their key differences, discuss the journey from a data lake to a data swamp, and provide cautionary steps to prevent the transformation. We will also cover strategies for recovery if you find yourself dealing with a data swamp. Let's dive in!
Understanding the Basics: Data Lake and Data Swamp
Before we explore the differences between data lakes and data swamps, let's define each concept.
When delving deeper into the realm of data management, it's essential to understand the nuances between a data lake and a data swamp. These terms represent two contrasting states of data organization and governance within an organization's data infrastructure.
What is a Data Lake?
A data lake is a repository that stores vast amounts of raw data in its native format, whether structured or unstructured. It serves as a centralized storage system for diverse data sources, including logs, documents, and databases. Unlike traditional data warehouses, a data lake allows data to be stored as-is, without the need for upfront schema design or data transformation. This makes it highly flexible and scalable, enabling organizations to harness the power of big data.
Within a data lake, data is stored in its raw form, preserving its original structure and content. This raw data can later be transformed and analyzed to extract valuable insights, making data lakes a valuable asset for data-driven decision-making processes.
What is a Data Swamp?
A data swamp, on the other hand, is a state that a data lake can deteriorate into. It occurs when the data within the lake becomes disorganized, poorly structured, and lacks proper governance. A data swamp hampers data usability, making it difficult for stakeholders to find relevant information and derive meaningful insights. It can result from negligence, lack of data governance, or an inadequate understanding of data management best practices.
When a data lake transforms into a data swamp, it poses significant challenges for organizations. Data swamps lead to increased data redundancy, decreased data quality, and hindered data accessibility. Without proper data governance measures in place, organizations risk losing the potential benefits of their data assets, ultimately impeding their ability to make informed decisions based on reliable insights.
Key Differences Between Data Lake and Data Swamp
Now that we have a clear understanding of what data lakes and data swamps are, let's explore their key differences:
Data Structure and Organization
In a data lake, data is stored in its raw form, without predefined schemas. This allows for agility and faster data ingestion. However, in a data swamp, the lack of proper organization and structure makes it challenging to find specific datasets or understand their context. Without organization, a data swamp can quickly become an unmanageable mess, hindering data discovery and utilization.
Imagine walking into a data swamp. The murky waters are filled with scattered data, floating aimlessly without any clear structure. It's like trying to find a needle in a haystack, except the haystack is constantly shifting and expanding. Without a proper organizational framework, users find themselves wading through the swamp, desperately searching for the data they need. This lack of structure not only wastes valuable time but also hampers the ability to make informed decisions based on reliable data.
Accessibility and Usability
A well-structured data lake provides easy accessibility to a wide range of users, including data scientists, analysts, and business stakeholders. It promotes data democratization and enables self-service analytics. In contrast, a data swamp's disorganized state makes it difficult for users to locate relevant data or understand its reliability. This impacts productivity and can lead to data distrust within the organization.
Imagine a data lake as a well-maintained library, with neatly labeled shelves and a comprehensive catalog. Users can easily navigate through the library, finding the books they need with minimal effort. The library promotes a sense of empowerment, allowing users to explore and extract knowledge at their own pace. On the other hand, a data swamp resembles a chaotic storage room, with boxes piled haphazardly and no clear labeling system. Users are left feeling frustrated and lost, unsure if the information they find is trustworthy or even relevant. This lack of accessibility and usability hampers the organization's ability to leverage its data assets effectively.
Data Quality and Integrity
Data lakes can accommodate both raw and curated data. With proper data governance and quality measures in place, data lakes can ensure high-quality data and maintain data integrity. However, in a data swamp, the lack of governance and quality controls means that data can be unreliable, inaccurate, or outdated. It erodes trust in the data and undermines decision-making processes.
Imagine a data lake as a well-guarded fortress, with strict security measures and vigilant guards ensuring the integrity of its contents. Data is carefully curated, verified, and validated before being admitted into the lake. This ensures that the data is of high quality, enabling users to make informed decisions with confidence. On the other hand, a data swamp resembles a neglected wasteland, with no safeguards in place to protect the data from corruption or contamination. Users are left questioning the accuracy and reliability of the data they encounter, leading to skepticism and hesitation when making critical business decisions.
The Journey from Data Lake to Data Swamp
Transforming a well-designed data lake into a data swamp doesn't happen overnight. It is the result of various factors that gradually degrade the state of the lake. Let's explore some common causes for this transformation:
One significant factor contributing to the transition from a data lake to a data swamp is the lack of proper data stewardship. Data stewardship involves defining clear roles and responsibilities for managing and maintaining data within the lake. When there is a lack of designated data stewards or a clear governance structure, data can quickly become chaotic and overwhelming, leading to the formation of a data swamp.
Additionally, another common cause of this transformation is the absence of a data lifecycle management strategy. Without a well-defined plan for data retention, archival, and deletion, the data lake can become bloated with unnecessary or obsolete data, making it challenging to sift through and extract valuable insights.
Common Causes of Transformation
One of the primary causes of the data lake turning into a swamp is a lack of data governance. Without proper governance policies and practices, data can become disorganized, duplicated, or even lost within the lake. Inadequate metadata management, failure to define data ownership, and absence of data quality controls can further contribute to this transformation.
Signs Your Data Lake is Becoming a Swamp
There are several indicators that your data lake might be transforming into a swamp. Some of these signs include difficulty in locating specific datasets, frequent encounter of irrelevant or outdated data, and an increase in data quality issues. Monitoring these signs can help you take timely actions to prevent further deterioration.
Cautionary Steps to Prevent a Data Swamp
Prevention is always better than cure, especially when it comes to data management. Let's explore some cautionary steps you can take to prevent your data lake from transforming into a swamp:
Implementing Data Governance
Data governance is essential to maintain the health of your data lake. It involves defining data ownership, establishing data quality standards, and implementing policies and procedures for data management. By implementing a robust data governance framework, you can ensure data integrity, accessibility, and usability within your data lake.
Ensuring Data Quality
Data quality directly impacts the value and reliability of the insights derived from a data lake. Implementing data quality controls, such as data profiling, validation, and cleansing, is crucial to prevent your lake from becoming a swamp. By regularly monitoring and improving data quality, you can maintain the trustworthiness of your data assets.
Regular Data Audits and Clean-ups
Periodic data audits and clean-ups are vital to keep your data lake organized and clutter-free. Identify and remove redundant, outdated, or irrelevant data that no longer adds value. By conducting these audits and clean-ups, you can optimize the performance and usability of your data lake.
Recovering from a Data Swamp
If your data lake has already transformed into a swamp, don't lose hope. With the right strategies and tools, you can recover from this state and regain control over your data. Let's explore some strategies for data swamp remediation:
Strategies for Data Swamp Remediation
One effective strategy is to perform a comprehensive data profiling and assessment to understand the depth of the data swamp. This will help you identify areas that require immediate attention and prioritize your recovery efforts. Additionally, investing in data management tools, such as data cataloging and metadata management solutions, can greatly assist in organizing, cleansing, and governing your data.
Role of Data Management Tools in Recovery
Data management tools play a crucial role in recovering from a data swamp. By leveraging data cataloging tools, you can create a searchable inventory of your data assets and improve data discoverability. Metadata management tools enable you to capture and manage data definitions, lineage, and relationships, ensuring proper contextual understanding of your data. These tools, when used in conjunction with data governance practices, can help you reclaim the value of your data lake.
In conclusion, understanding the differences between data lakes and data swamps is essential for effective data management. By taking cautionary steps and implementing proper data governance, quality control, and regular audits, you can prevent your data lake from deteriorating into a swamp. However, if you find yourself in a data swamp, don't panic. With appropriate strategies, tools, and dedication, you can recover and regain the full potential of your data assets.
You might also like
Get in Touch to Learn More
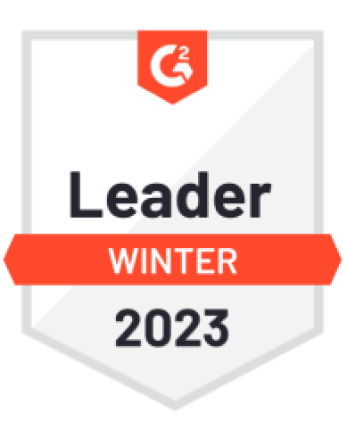
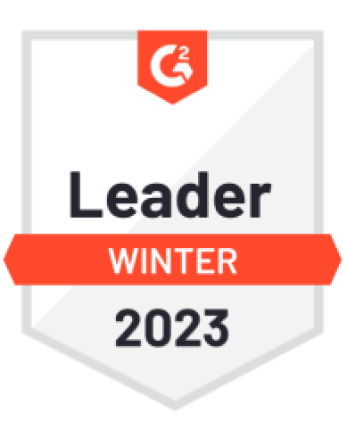

“[I like] The easy to use interface and the speed of finding the relevant assets that you're looking for in your database. I also really enjoy the score given to each table, [which] lets you prioritize the results of your queries by how often certain data is used.” - Michal P., Head of Data