Data Quality Management: The Ultimate Guide
Learn everything you need to know about data quality management

Data quality management is a crucial aspect of any organization's data strategy. In today's data-driven world, businesses heavily rely on high-quality data to make informed decisions, drive efficiency, and deliver exceptional customer experiences. This comprehensive guide will take you through the fundamentals of data quality management, its role in business, implementation steps, tools, techniques, and measuring success.
Understanding Data Quality Management
Data quality management refers to the process of maintaining and improving the quality of data within an organization. It ensures that data is accurate, consistent, reliable, and relevant for its intended use. Poor data quality can lead to erroneous insights, ineffective decision-making, and negatively impact multiple areas of the business.
Definition and Importance of Data Quality Management
Data quality management involves establishing standards, processes, and controls to ensure data accuracy, completeness, consistency, and timeliness. This encompasses data cleansing, data profiling, data validation, and ongoing data monitoring efforts. By prioritizing data quality, organizations can enhance the reliability and credibility of their data, ultimately leading to better business outcomes.
Key Components of Data Quality Management
Successful data quality management comprises various key components, including:
- Data Governance: Establishing policies, procedures, and responsibilities for managing data quality.
- Data Profiling: Analyzing data to identify inconsistencies, errors, and anomalies.
- Data Cleansing: Correcting, standardizing, and removing errors from data to ensure its accuracy.
- Data Validation: Verifying data against predefined rules to ensure its integrity.
- Data Monitoring: Continuously monitoring data quality to identify and address issues promptly.
Data governance is a critical aspect of data quality management. It involves defining and implementing a framework that outlines the roles, responsibilities, and processes for managing data within an organization. This framework ensures that data is managed consistently across different departments and functions, promoting data integrity and reliability.
Data profiling plays a crucial role in identifying inconsistencies, errors, and anomalies within the data. It involves analyzing the data to understand its structure, content, and quality. By conducting data profiling, organizations can gain insights into the overall health of their data, enabling them to prioritize data cleansing efforts and make informed decisions based on reliable information.
Data cleansing is a fundamental step in data quality management. It involves correcting, standardizing, and removing errors from the data. This process ensures that the data is accurate, complete, and consistent. By eliminating duplicate records, correcting misspellings, and standardizing formats, organizations can improve the quality and reliability of their data, enabling better decision-making and analysis.
Data validation is another critical component of data quality management. It involves verifying the data against predefined rules or criteria to ensure its integrity. By validating the data, organizations can identify any discrepancies or inconsistencies that may exist, allowing them to take corrective actions and maintain data accuracy.
Data monitoring is an ongoing process that involves continuously assessing and evaluating the quality of data. By implementing data monitoring mechanisms, organizations can proactively identify and address any data quality issues that may arise. This ensures that the data remains accurate, consistent, and reliable over time, enabling organizations to make sound business decisions based on trustworthy information.
The Role of Data Quality in Business
Data quality plays a pivotal role in various aspects of business operations, including:
Impact on Decision Making
High-quality data serves as a solid foundation for making well-informed business decisions. Decision-makers heavily rely on accurate and reliable data to assess market trends, identify opportunities, identify risks, and optimize operational strategies. Poor data quality can distort these insights, leading to erroneous decisions with potentially detrimental consequences.
Influence on Customer Satisfaction
Quality data is critical for delivering exceptional customer experiences. By ensuring accurate customer information, businesses can personalize interactions, deliver targeted marketing campaigns, and provide efficient customer support. Inaccurate or outdated data, on the other hand, can result in customer dissatisfaction, missed sales opportunities, and damaged brand reputation.
Moreover, data quality also impacts the efficiency of internal processes within an organization. When data is accurate and consistent, it streamlines various operational workflows such as inventory management, supply chain logistics, and financial reporting. This efficiency not only saves time and resources but also enhances overall productivity and competitiveness in the market.
Role in Regulatory Compliance
Another crucial aspect of data quality in business is its role in regulatory compliance. Many industries are subject to strict data protection regulations, such as GDPR or HIPAA, which require businesses to maintain accurate and secure data. Non-compliance can result in hefty fines and legal consequences. Therefore, ensuring data quality is not only essential for operational purposes but also for meeting legal obligations and safeguarding the company's reputation.
Steps to Implementing Data Quality Management
Implementing a robust data quality management strategy requires a systematic approach. Here are the key steps involved:
Identifying Data Quality Issues
The initial step is to analyze existing data and identify quality issues such as duplicate records, inconsistent formats, missing data, or inaccuracies. This helps in understanding the scope and scale of the data quality problem and forms the basis for subsequent actions.
Identifying data quality issues is a critical task that involves not only technical analysis but also a deep understanding of the business processes that generate and consume the data. It requires collaboration between data analysts, data scientists, and business stakeholders to ensure a comprehensive assessment of data quality across different systems and sources.
Developing a Data Quality Plan
Once the issues are identified, a comprehensive data quality plan needs to be developed. This plan outlines the goals, objectives, and actions necessary to improve data quality. It should define data quality standards, establish data governance policies, specify data cleansing and validation procedures, and allocate resources accordingly.
Developing a data quality plan is not a one-time activity but an iterative process that evolves as new data challenges emerge and organizational priorities shift. It requires continuous collaboration between IT teams, data stewards, and business users to ensure that the plan remains relevant and effective in addressing evolving data quality needs.
Executing the Data Quality Plan
Execution involves implementing the data quality plan across the organization. This includes performing data cleansing, standardization, and validation activities as per the defined processes. Regular communication, training, and monitoring are crucial during this phase to ensure adherence to the plan and proactively address any challenges or issues that may arise.
Executing the data quality plan requires a coordinated effort from cross-functional teams within the organization. It involves not only technical implementation of data quality tools and processes but also change management activities to drive cultural and behavioral changes towards a data-driven and quality-focused mindset. Continuous feedback loops and performance metrics are essential to measure the effectiveness of the data quality initiatives and drive continuous improvement.
Tools and Techniques for Data Quality Management
Several tools and techniques assist organizations in effectively managing data quality. These include:
Data Profiling and Cleansing
Data profiling tools analyze data sets to identify anomalies, inconsistencies, and patterns. They help in understanding data quality issues and provide insights on data completeness, accuracy, and consistency. Data cleansing tools are used to correct errors, eliminate duplicates, and standardize data formats to ensure its reliability and usability.
Data Integration and Synchronization
Data integration tools facilitate combining data from multiple sources, ensuring consistency, and eliminating redundancies. These tools enable organizations to create a unified and reliable view of data, enabling accurate reporting, analysis, and decision-making.
Master Data Management
Master Data Management (MDM) tools enable organizations to establish a centralized and authoritative source of key data entities such as customers, products, and locations. MDM ensures data consistency, eliminates duplicates, and enhances data quality across systems and processes.
Another crucial aspect of data quality management is data governance. Data governance involves the overall management of the availability, usability, integrity, and security of data within an organization. It establishes processes and policies to ensure that data meets specific standards and is used effectively to achieve business objectives. Data governance frameworks help in defining roles and responsibilities, establishing data quality metrics, and ensuring compliance with regulations.
Furthermore, data quality management also encompasses data enrichment techniques. Data enrichment involves enhancing existing data sets with additional information to improve their value and accuracy. This process may include appending demographic data, geolocation information, or social media insights to existing records. By enriching data, organizations can gain a deeper understanding of their customers, enhance personalization efforts, and make more informed business decisions based on comprehensive and enriched data sets.
Measuring the Success of Data Quality Management
To gauge the effectiveness of data quality management efforts, organizations employ specific key performance indicators (KPIs) and conduct regular data audits. These steps help in assessing the impact of data quality improvements and identifying areas for further enhancement.
Key Performance Indicators for Data Quality
Common KPIs for measuring data quality include data accuracy, completeness, timeliness, consistency, and validity. By consistently monitoring these metrics, organizations can track the progress of data quality initiatives and make informed decisions regarding future enhancements.
Regular Data Audits
Data audits involve conducting periodic reviews of data to identify any quality issues or deviations from defined standards. These audits help in proactively identifying and rectifying data quality issues, ensuring that data remains accurate, reliable, and fit for purpose.
In conclusion, data quality management is a critical discipline that organizations must prioritize to ensure accurate and reliable data for decision-making and operational efficiency. By understanding its importance, following a structured approach, leveraging appropriate tools and techniques, and measuring success through KPIs and regular audits, organizations can effectively manage data quality and unlock its full potential.
You might also like
Get in Touch to Learn More
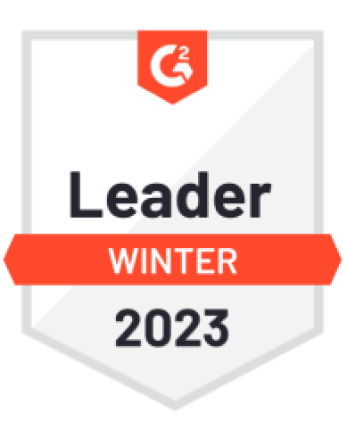
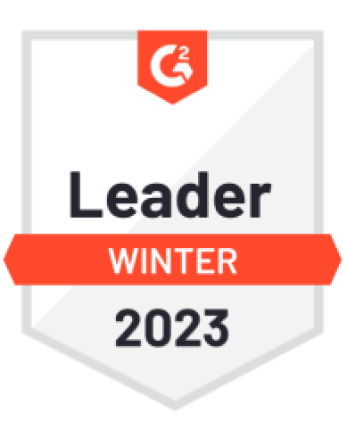

“[I like] The easy to use interface and the speed of finding the relevant assets that you're looking for in your database. I also really enjoy the score given to each table, [which] lets you prioritize the results of your queries by how often certain data is used.” - Michal P., Head of Data