Data Quality Metrics: 5 Metrics to Monitor the Health of Your Data Estate
Learn how to ensure the accuracy and reliability of your data with these 5 essential data quality metrics.

Data quality is a critical aspect for any organization that relies on data to make informed business decisions. Without accurate and reliable data, organizations may face serious consequences, including financial losses, compliance issues, and a damaged reputation. To ensure the health of your data estate, it is essential to monitor and measure the quality of your data regularly. In this article, we will explore five key data quality metrics that can help you assess and improve the quality of your data.
Understanding the Importance of Data Quality
Data quality refers to the degree to which data meets the requirements of its intended use. In simple terms, it is about ensuring the accuracy, completeness, consistency, validity, and timeliness of your data. Poor data quality can have detrimental effects on various aspects of your business operations, such as decision-making, customer satisfaction, and operational efficiency.
In today's data-driven world, organizations collect and store vast amounts of data from various sources. This data includes customer information, sales data, financial records, inventory data, and more. However, data is only valuable if it is accurate and reliable. Without proper data quality management, organizations risk making flawed decisions based on inaccurate or incomplete data.
Moreover, data quality is crucial for regulatory compliance. Many industries, such as healthcare and finance, have strict regulations regarding the handling and reporting of data. Failing to maintain high data quality standards can result in severe penalties and legal consequences.
Ensuring data quality involves a combination of processes, tools, and people. Data quality management often includes data profiling, data cleansing, data validation, and data governance. Data profiling helps organizations understand the structure and content of their data, identifying inconsistencies and anomalies. Data cleansing involves correcting errors, removing duplicates, and standardizing data formats to improve overall quality. Data validation ensures that data meets specific criteria or standards set by the organization.
Furthermore, data governance plays a critical role in maintaining data quality. Data governance involves establishing policies, procedures, and responsibilities for ensuring data quality and security. It helps organizations define data ownership, establish data quality metrics, and enforce data quality standards across the organization. By implementing robust data governance practices, organizations can improve data quality, enhance decision-making processes, and mitigate risks associated with poor data quality.
The Role of Metrics in Data Quality Management
Metrics play a crucial role in data quality management. They provide a quantifiable and objective way to measure the quality of your data. By monitoring data quality metrics regularly, organizations can identify areas of improvement, track progress, and make data-driven decisions to enhance the overall quality of their data.
The Concept of Data Quality Metrics
Data quality metrics are specific measurements used to assess the quality of data. These metrics can be both quantitative and qualitative in nature, providing insights into different aspects of data quality. By defining and tracking these metrics, organizations can gain a deeper understanding of the strengths and weaknesses of their data estate.
For example, the completeness metric measures the extent to which data is present in a dataset. It helps organizations determine if any crucial information is missing, which could impact decision-making and analysis. Similarly, the uniqueness metric assesses the presence of duplicate records in a dataset, ensuring that data is not duplicated and causing inaccuracies.
There are various data quality metrics available, and the choice of metrics depends on the specific requirements and objectives of each organization. However, some commonly used metrics include completeness, uniqueness, timeliness, validity, and consistency.
How Metrics Enhance Data Quality
Metrics enable organizations to identify areas of data quality improvement. By establishing benchmarks and targets for each metric, organizations can set goals and track progress over time. For instance, if the completeness metric reveals that certain datasets consistently have missing values, organizations can implement data collection processes to ensure all required information is captured.
Moreover, metrics facilitate the identification of data anomalies and inconsistencies, allowing organizations to take corrective actions promptly. For example, the validity metric helps organizations identify data that does not conform to predefined rules or standards. By identifying and addressing these issues, organizations can improve the accuracy and reliability of their data.
Metrics also promote accountability and transparency within an organization. By making data quality metrics visible to stakeholders, organizations can foster a culture of data-driven decision-making and continuous improvement. When stakeholders have access to metrics such as timeliness, which measures the data's relevance and currency, they can make informed decisions based on up-to-date and reliable information.
In conclusion, metrics are essential tools in data quality management. They provide organizations with valuable insights into the strengths and weaknesses of their data, enabling them to make data-driven decisions and continuously improve the quality of their data. By tracking metrics and taking corrective actions, organizations can ensure that their data is accurate, reliable, and fit for purpose.
The Five Key Data Quality Metrics
Data quality is a critical aspect of any organization's operations. Ensuring that the data used for analysis and decision-making is accurate and reliable is paramount. In this article, we will explore the five key data quality metrics that organizations should monitor to maintain data integrity and enhance their overall performance.
Completeness of Data
Completeness measures the extent to which data contains all the required information. It is crucial to have complete data to avoid inaccurate analysis and flawed decision-making. Imagine trying to analyze customer behavior without having all the necessary data points. By monitoring the completeness metric, organizations can identify missing data elements and take corrective actions, such as data enhancement or data collection. Regular data profiling and data validation processes can help ensure data completeness.
Uniqueness of Data
Uniqueness measures the extent to which data contains duplicate records or values. Duplicate data can skew analysis results and lead to redundant processes or inaccurate reporting. It is essential to have a system in place to detect and eliminate duplicate data. By monitoring the uniqueness metric, organizations can improve the accuracy and efficiency of their operations. They can implement data deduplication techniques or utilize advanced algorithms to identify and remove duplicate records, ensuring that the data used for analysis is unique and reliable.
Timeliness of Data
Timeliness measures how up-to-date the data is in relation to its intended use. Outdated data may result in missed opportunities, inefficient processes, or erroneous decisions. Imagine making crucial business decisions based on outdated market trends. By monitoring the timeliness metric, organizations can ensure that data is captured, processed, and made available in a timely manner. They can implement automated data capture and integration processes, utilize real-time data feeds, and establish regular data refresh cycles to improve data timeliness. This way, decision-makers can rely on the most recent and relevant information to drive their strategies.
Validity of Data
Validity measures the extent to which data conforms to predefined rules or constraints. Invalid data can result in skewed analysis or system failures. It is crucial to have mechanisms in place to identify and rectify data that violates predefined rules or fails data integrity checks. By monitoring the validity metric, organizations can maintain data quality and reliability. They can implement data validation checks, automated data cleansing processes, and establish well-defined data entry guidelines. These measures ensure that data is accurate, consistent, and adheres to the defined rules, enabling organizations to make informed decisions based on reliable information.
Consistency of Data
Consistency measures the degree of agreement or conformity between different data sources or data elements. Inconsistent data can lead to discrepancies in reporting, conflicting information, and unreliable analysis. It is crucial to establish data governance frameworks to maintain consistency across the data estate. By monitoring the consistency metric, organizations can identify data discrepancies, resolve conflicts, and ensure that data from various sources aligns. They can implement data integration processes, establish data quality rules, and utilize master data management techniques to achieve data consistency. This way, decision-makers can have confidence in the accuracy and reliability of the data they use.
By focusing on these five key data quality metrics - completeness, uniqueness, timeliness, validity, and consistency - organizations can enhance their data-driven decision-making processes, improve operational efficiency, and gain a competitive edge in today's data-driven world.
Implementing Data Quality Metrics in Your Data Estate
Steps to Implement Data Quality Metrics
Implementing data quality metrics requires a systematic approach. Here are the steps you can follow:
- Define data quality objectives: Identify the specific data quality goals and objectives for your organization.
- Select relevant metrics: Choose the data quality metrics that align with your objectives and requirements.
- Define data quality rules: Establish rules or criteria against which the metrics will be evaluated.
- Collect and analyze data: Gather data from various sources and apply the defined metrics to assess data quality.
- Identify improvement areas: Identify areas of improvement based on the results of the data quality analysis.
- Take corrective actions: Implement measures to improve data quality, such as data cleansing, data enrichment, or process optimization.
- Monitor and track progress: Continuously monitor data quality metrics and track progress towards your data quality objectives.
Challenges in Implementing Data Quality Metrics
Implementing data quality metrics is not without challenges. Organizations may face the following obstacles:
- Lack of data governance: In the absence of clear data governance policies and processes, ensuring data quality becomes challenging.
- Data integration complexities: Integrating data from multiple sources with varying formats and structures can introduce data quality issues.
- Data migration: During data migration or system upgrades, data quality may be compromised if proper measures are not taken.
- Limited resources and expertise: Implementing and managing data quality metrics require dedicated resources and expertise, which may be limited in some organizations.
Despite these challenges, organizations that prioritize data quality management and invest in the right tools, technologies, and processes can significantly improve the health of their data estate and gain a competitive advantage.
Conclusion
In today's data-driven world, data quality is paramount for organizations to make informed decisions and drive business success. By implementing and monitoring data quality metrics, organizations can ensure the accuracy, completeness, consistency, validity, and timeliness of their data. The five key data quality metrics discussed in this article - completeness, uniqueness, timeliness, validity, and consistency - provide a comprehensive framework to assess, improve, and maintain data quality. By following best practices and addressing the challenges associated with implementing data quality metrics, organizations can establish a solid foundation for their data-driven initiatives and unlock the true value of their data estate.
You might also like
Get in Touch to Learn More
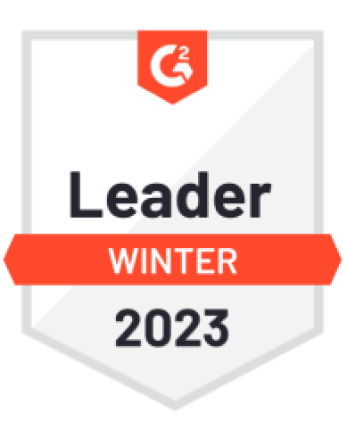
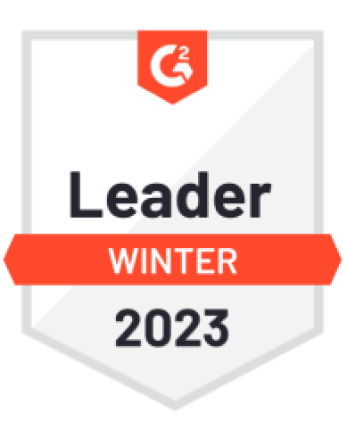

“[I like] The easy to use interface and the speed of finding the relevant assets that you're looking for in your database. I also really enjoy the score given to each table, [which] lets you prioritize the results of your queries by how often certain data is used.” - Michal P., Head of Data