Data Quality Strategy: 10 Steps to Excellence
Discover 10 essential steps to creating a top-notch data quality strategy.

In today's data-driven world, organizations are becoming increasingly aware of the importance of data quality. Whether it is customer information, product data, or financial records, the accuracy and reliability of data are crucial for making informed business decisions. A well-defined data quality strategy is essential for organizations looking to achieve excellence in this area. This article outlines ten key steps to developing and implementing a data quality strategy that will ensure the accuracy, completeness, and consistency of your data.
Understanding the Importance of Data Quality
Defining Data Quality
Data quality refers to the degree to which data meets certain predefined standards or requirements. These standards can include accuracy, completeness, consistency, timeliness, and reliability. High-quality data is clean, consistent, and error-free. It is the foundation upon which organizations build their operations and make informed decisions.
Ensuring data accuracy involves validating information against reliable sources and cross-referencing data points to eliminate discrepancies. Completeness, on the other hand, requires that all necessary data fields are populated and up-to-date. Consistency involves ensuring that data is uniform across different databases and systems, avoiding duplication or conflicting information. Timeliness refers to the relevance of data in relation to the decision-making process, emphasizing the importance of real-time updates. Lastly, reliability pertains to the trustworthiness of data sources and the methods used to collect and process information.
The Impact of Poor Data Quality
Poor data quality can have severe consequences for businesses. Inaccurate or incomplete data can lead to unreliable reports, flawed analyses, and misguided decision-making. It can result in financial losses, missed opportunities, and damaged reputation. Therefore, organizations must proactively address data quality issues to mitigate risks and maximize the value of their data assets.
Moreover, poor data quality can also lead to compliance issues, as regulatory bodies increasingly require accurate and secure data handling practices. Non-compliance can result in hefty fines and legal implications, further underscoring the importance of maintaining high data quality standards. Additionally, in today's data-driven economy, where personalized marketing and customer experiences are paramount, low-quality data can hinder efforts to understand consumer behavior and preferences accurately, leading to ineffective targeting and reduced customer satisfaction.
Laying the Foundation for a Data Quality Strategy
Establishing a robust data quality strategy is crucial for organizations looking to leverage their data effectively and make informed decisions. By ensuring that data is accurate, consistent, and reliable, businesses can enhance operational efficiency, drive innovation, and gain a competitive edge in the market.
Identifying Key Stakeholders
The first step in developing a data quality strategy is to identify key stakeholders who play a pivotal role in managing and overseeing data quality initiatives. These stakeholders encompass a diverse group of individuals, including data stewards responsible for data governance, IT personnel tasked with data management and integration, business users who rely on data for daily operations, and executives who provide strategic direction and support. Collaborating effectively with these stakeholders is essential to establish a shared understanding of data quality requirements and priorities.
Setting Data Quality Goals
Once the key stakeholders have been identified, the next critical step is to establish clear and measurable data quality goals that align with the organization's overarching business objectives. These goals serve as guiding principles for data quality improvement efforts and help quantify the expected outcomes. For instance, organizations may aim to achieve a specific level of data accuracy, completeness, or timeliness to support better decision-making processes and enhance customer satisfaction. Setting ambitious yet achievable data quality goals is fundamental to driving continuous improvement and demonstrating the value of data quality initiatives within the organization.
Implementing Your Data Quality Strategy
Step-by-Step Guide to Data Cleansing
Data cleansing is the process of identifying and correcting or removing errors, inconsistencies, and inaccuracies in your data. It involves a series of steps, including data profiling, data standardization, duplicate identification and merging, and error correction. A well-defined data cleansing process ensures that your data is accurate and reliable.
One critical aspect of data cleansing is data profiling, which involves analyzing the content and structure of your data to understand its quality and completeness. This step helps in identifying anomalies and inconsistencies that need to be addressed. Data standardization is another key component, where data is transformed into a consistent format to improve its accuracy and usability. By standardizing data elements such as date formats or address structures, you can enhance the overall quality of your data.
Establishing Data Governance
Data governance is the framework of policies, procedures, and roles that ensure the effective management and control of data assets within an organization. It provides the structure and accountability needed to maintain data quality over time. Establishing data governance involves defining data ownership, creating data quality rules, and implementing data governance workflows.
Defining data ownership is crucial in data governance as it clarifies who is responsible for the accuracy and integrity of specific data sets. Data quality rules set the standards for data accuracy, completeness, and consistency, guiding data management practices within the organization. Implementing data governance workflows streamlines the processes for data validation, approval, and monitoring, ensuring that data quality standards are upheld consistently.
Monitoring and Maintaining Data Quality
Regular Data Audits
Data audits are systematic assessments of data quality, completeness, and accuracy. Regular data audits help identify any data quality issues and ensure that the data remains consistent, reliable, and up to date. It involves reviewing data against predefined quality metrics, identifying areas for improvement, and taking corrective actions.
During a data audit, various tools and techniques can be utilized to assess the quality of data, such as data profiling, data cleansing, and data validation. Data profiling helps in understanding the structure and content of the data, while data cleansing involves correcting or removing any inaccuracies or inconsistencies. Data validation ensures that the data conforms to predefined standards and rules, enhancing its overall quality.
Continuous Improvement of Data Quality
Data quality is not a one-time effort; it requires continuous monitoring and improvement. This involves adopting data quality best practices, leveraging advanced technologies, and implementing data quality metrics to measure and track data quality over time. Continuous improvement ensures that your data quality strategy remains effective and aligned with evolving business needs.
Implementing a data governance framework is essential for ensuring continuous improvement in data quality. Data governance establishes policies, procedures, and responsibilities for managing and improving data quality within an organization. It helps in defining data quality standards, ensuring data integrity, and promoting data stewardship across different departments and teams. By incorporating data governance practices, organizations can foster a culture of data quality consciousness and accountability.
Overcoming Common Data Quality Challenges
Dealing with Incomplete or Inaccurate Data
Incomplete or inaccurate data is one of the most common challenges organizations face. To overcome this challenge, organizations can implement data validation techniques, enforce stricter data entry rules, and provide training to data entry personnel. Additionally, integrating data from multiple sources and leveraging data cleansing techniques can help improve data completeness and accuracy.
One effective way to address incomplete data is through the use of data imputation techniques. Data imputation involves filling in missing values with estimated or calculated values based on existing data patterns. This method can help organizations maintain data integrity and ensure that analytical models are built on robust datasets.
Managing Data Quality in Large Datasets
Managing data quality in large datasets can be complex and challenging. Organizations can utilize data profiling tools and techniques to analyze large datasets and identify potential data quality issues. Implementing scalable data quality processes, such as parallel processing and distributed data validation, can help improve data quality management in large-scale environments.
Another approach to managing data quality in large datasets is through the implementation of data governance frameworks. Data governance involves defining policies, procedures, and responsibilities for ensuring data quality and integrity across an organization. By establishing clear guidelines and accountability structures, organizations can better manage and maintain data quality in their large datasets.
Conclusion
Achieving data quality excellence requires a well-defined data quality strategy that addresses the various aspects of data quality management. By following the ten steps outlined in this article, organizations can lay the foundation, implement effective data quality processes, and continuously monitor and improve data quality. By doing so, they can unlock the true value of their data assets and make informed decisions that drive business success.
You might also like
Get in Touch to Learn More
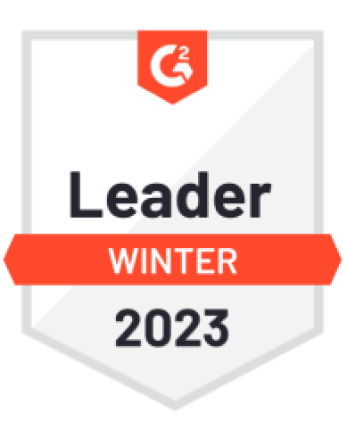
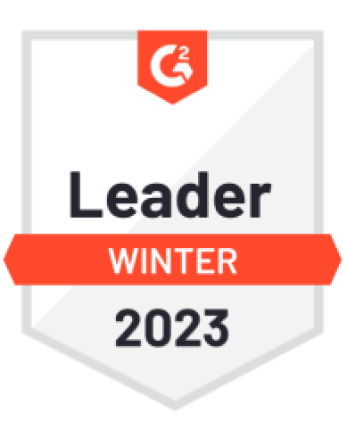

“[I like] The easy to use interface and the speed of finding the relevant assets that you're looking for in your database. I also really enjoy the score given to each table, [which] lets you prioritize the results of your queries by how often certain data is used.” - Michal P., Head of Data