McKinsey Data Governance Framework: 5 Steps to Use It
Discover how the McKinsey Data Governance Framework can revolutionize your data management strategy with these 5 actionable steps.
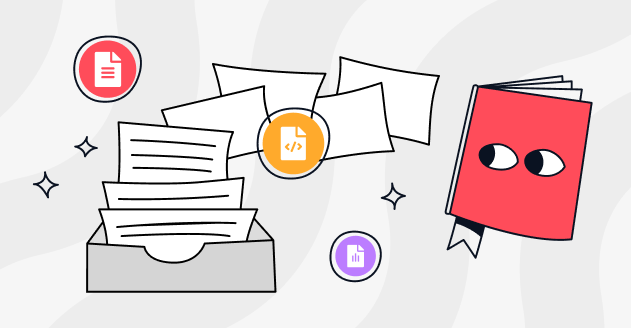
Data governance is crucial for effective data management in any organization. A solid data governance frameworkconnects different aspects of data management, ensuring data integrity, quality, and consistency, which leads to better decision-making and business results. The McKinsey Data Governance Framework is a popular choice for many companies. This article explores its main components and provides five steps to effectively implement it.
Understanding the McKinsey Data Governance Framework
Before we discuss implementation steps, let's look at the importance of data governance. Data governance is vital for organizations. It helps ensure data is high-quality, accessible, accurate, secure, and usable. As organizations collect more data, they need to establish a governance framework to guide data management. The McKinsey Data Governance Framework offers a complete approach to this challenge.
The Importance of Data Governance
Data governance is crucial for any company that uses data to make decisions. It's like having a set of rules for how to handle information. These rules help make sure the data is correct, consistent, and kept safe. They also support compliance with regulations like GDPR and CCPA.
When a company has good data governance:
- They can trust their data to make smart choices
- They follow laws about protecting people's information
- Everyone in the company uses data in the same way
- It's clear who can see what data and why
Without these rules, things can get messy. Data might be scattered all over the place, with different departments having their own versions. This can lead to confusion and mistakes. It's also risky because sensitive information might not be properly protected.
Good data governance is crucial when many people in a company use data. Without it, increasing data access can create more problems instead of being helpful—this is known as the self-service paradox. It's like giving everyone keys to a library without a system for checking out books; things could become chaotic quickly.
In short, data governance helps companies use their information wisely and safely, which is really important in today's data-driven world.
Key Components of the McKinsey Framework
The McKinsey Data Governance Framework has several key parts:
- Data Strategy: Sets the organization's data goals and aligns them with business objectives, addressing the relationship between data governance and AI.
- Data Organization: Assigns roles and responsibilities for data governance, including defining key stakeholders like data owners, stewards, and users.
- Data Practices and Policies: Establishes data standards and policies for consistency, accessibility, and security, including data quality metrics and security measures.
- Data Governance Execution: Implements procedures to monitor, measure, and improve data governance, balancing self-service analytics with governance.
- Data Governance Challenges: Addresses potential challenges, including the self-service paradox and AI's impact on data governance.
By using this framework, organizations can manage data effectively, make better decisions, and stay competitive.
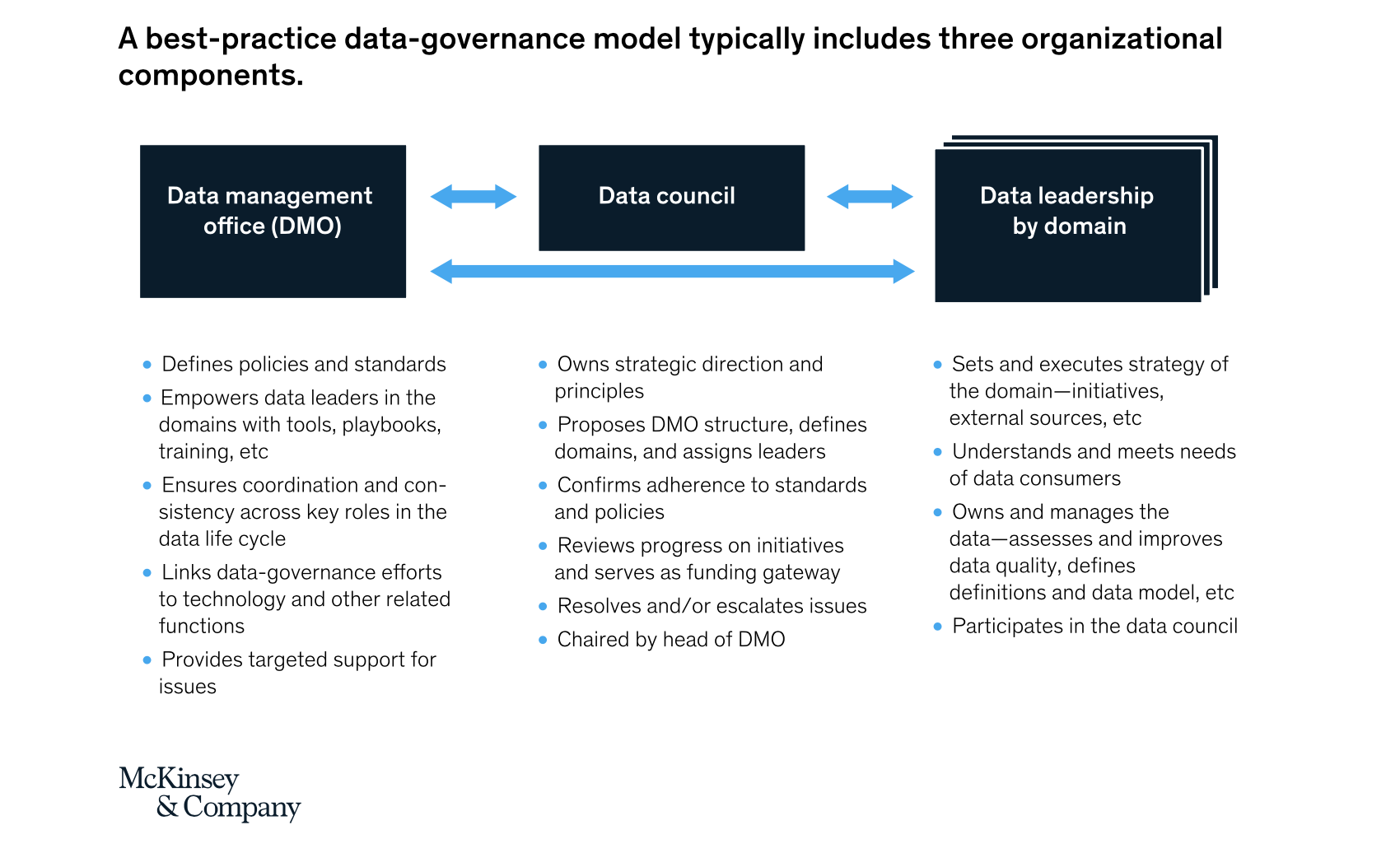
Step 1: Establishing Your Data Governance Vision
Defining Your Data Goals
To implement the McKinsey Data Governance Framework, first define your organization's data goals. Identify what you want to achieve through data governance, such as improving data quality or ensuring compliance with regulations.
Consider the needs of different stakeholders who use data in their daily work. This will help tailor your data governance vision to meet various departmental demands.
Aligning Vision with Business Objectives
Next, align your data governance vision with your organization's broader business goals. Determine how data governance can help achieve these objectives. For example, it could contribute to a customer-centric strategy by improving customer experiences.
Analyze your current data landscape to identify gaps or inefficiencies that data governance could address. This analysis can help build a strong case for data governance in your organization.
Step 2: Designing the Data Governance Organization
Identifying Key Roles and Responsibilities
In this step, identify the key roles needed for successful data governance implementation. These include data stewards, owners, custodians, and governance committee members. Assign clear responsibilities to these roles to establish accountability and foster a data governance culture.
Provide necessary training and resources to these key roles. Ongoing education is crucial to keep up with changes in the data landscape and regulatory requirements.
Structuring Your Data Governance Team
When structuring your data governance team, consider factors like organizational structure, reporting lines, and decision-making authority. This helps streamline communication and decision-making processes.
Foster collaboration within the data governance team and with other departments. This ensures data governance aligns with broader organizational goals.
Step 3: Defining Data Governance Practices and Policies
Setting Data Standards
Define data standards to ensure consistency and quality across your organization. Specify guidelines for data entry, format, and naming conventions. This reduces errors and improves data integrity.
Involve key stakeholders when setting these standards. Conduct training sessions to educate employees on the importance of these standards. Consider using automated data validation tools to enforce standards consistently.
Implementing Data Policies
Develop policies for data handling, storage, and access. Address data privacy, security, and compliance with relevant regulations. Review and update these policies regularly to align with changing business needs and regulations. Consider how AI can support policy enforcement and compliance monitoring.
Form a data governance committee with members from IT, legal, compliance, and business units to oversee policy implementation, monitor compliance, and address data issues. This committee should implement strategies that balance self-service analytics with governance to prevent the self-service paradox.
Example: Uber's Data Governance Framework
Uber operates in 70 countries and 10,000 cities, and they prioritize data governance as much as customer experience. Their system is flexible and regularly updated to comply with different local laws. Manikandan Thangarathnam, a Senior Director at Uber, explains that they integrate these laws into their software to ensure compliance. Uber has built a central platform to manage privacy and security, with customizable settings for local regulations. This approach helps them avoid problems like higher costs, unhappy customers, and legal issues.
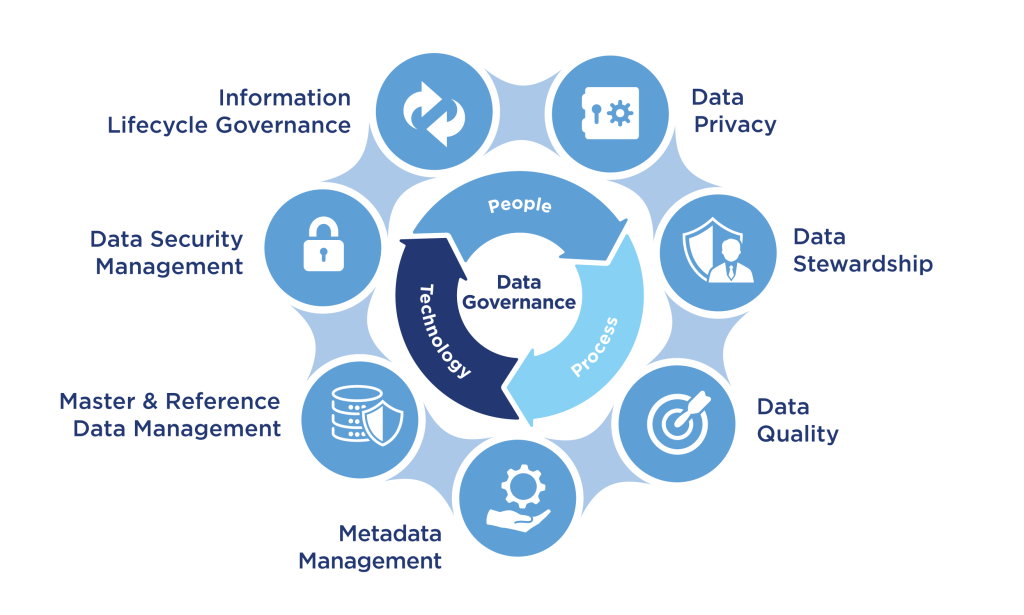
Step 4: Implementing the Data Governance Framework
Execution of the Data Governance Plan
With your vision, organization, and practices in place, it's time to execute the data governance plan. This involves putting the defined roles, responsibilities, and policies into action. Monitor and measure the effectiveness of your data governance practices regularly. Consider implementing AI-driven tools to enhance monitoring and measurement processes.
Integrate the framework into existing organizational processes. This may involve customizing policies for different departments. Establish clear communication channels and feedback mechanisms to address implementation issues.
Overcoming Implementation Challenges
Implementing a data governance framework can be challenging. It requires change management, stakeholder communication, and employee training. Create a change management plan, secure executive sponsorship, and invest in training programs to build data governance capabilities. ~~~~
Foster a culture that values data as a strategic asset and integrates data governance into daily operations. This enhances the overall effectiveness of your data governance initiatives.

Conclusion
By following these steps from the McKinsey Data Governance Framework, organizations can establish a robust data governance program. Effective data governance helps organizations harness their data's full potential, driving strategic decision-making and improving business outcomes.
CastorDoc offers an AI-powered solution that aligns with the McKinsey Data Governance Framework. Our platform combines advanced data governance, cataloging, and lineage capabilities with a user-friendly AI assistant, creating a powerful tool for implementing and maintaining robust data governance strategies.
By leveraging metadata and AI, CastorDoc helps organizations navigate the complexities of data management, balance self-service analytics with governance, and adapt to the era of AI-driven data governance. Don’t wait to turn data into business decisions - Try CastorDoc today.
You might also like
Get in Touch to Learn More
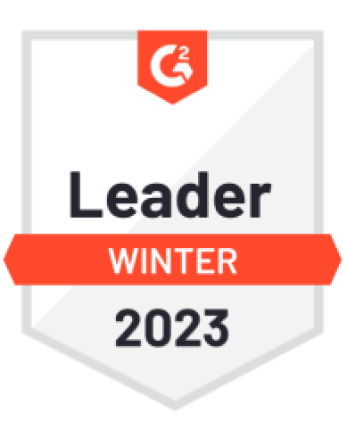
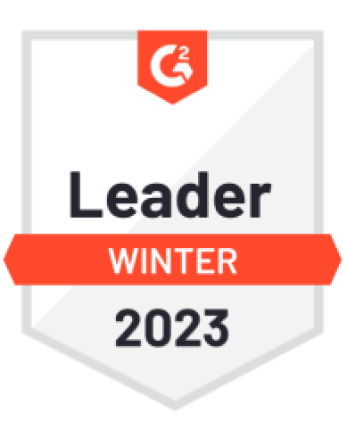

“[I like] The easy to use interface and the speed of finding the relevant assets that you're looking for in your database. I also really enjoy the score given to each table, [which] lets you prioritize the results of your queries by how often certain data is used.” - Michal P., Head of Data