Predictive Data Quality & Observability: A Complete Guide!
Unlock the power of predictive data quality and observability with our comprehensive guide! Learn how to harness the potential of data to drive informed decisions and maximize business performance.

In the digital age, data is the lifeblood of any organization. It drives decision-making, fuels innovation, and provides insights that can propel businesses to new heights. However, the quality of this data is paramount. Poor data quality can lead to misguided strategies, flawed insights, and ultimately, business failure. This is where predictive data quality and observability come into play.
Predictive Data Quality
Predictive data quality refers to the use of advanced algorithms and machine learning techniques to predict the quality of data before it is used for decision-making. This proactive approach ensures that only high-quality, accurate data is used, thereby improving the reliability of business decisions and strategies.
Implementing predictive data quality involves a series of steps. First, the data is collected from various sources. Then, it is cleaned and standardized. Next, predictive models are applied to the data to forecast its quality. Finally, the data is validated and used for decision-making.
Benefits of Predictive Data Quality
Predictive data quality offers numerous benefits. It improves the accuracy and reliability of business decisions by ensuring that they are based on high-quality data. It also reduces the risk of errors and inaccuracies in data, which can lead to costly mistakes. Furthermore, it saves time and resources that would otherwise be spent on manual data cleaning and validation.
Another significant benefit of predictive data quality is that it allows for continuous improvement. By constantly monitoring and predicting data quality, organizations can identify and address issues before they become problematic. This leads to improved data management and governance, and ultimately, better business outcomes.
Data Observability
Data observability, on the other hand, is the ability to fully understand the state of your data at any given time. It involves monitoring and visualizing data in real-time to identify issues, track changes, and ensure data integrity. Data observability is crucial for maintaining data quality and ensuring that data-driven decisions are based on accurate, up-to-date information.
Implementing data observability involves setting up systems and processes to monitor data continuously. This includes tracking data as it moves through different stages of the data lifecycle, from collection and processing to storage and use. It also involves using visualization tools to present data in a way that is easy to understand and interpret.
Benefits of Data Observability
Data observability offers several benefits. First, it provides a clear, real-time view of data, making it easier to identify and address issues promptly. This leads to improved data quality and reliability, which in turn enhances the accuracy of data-driven decisions.
Second, data observability improves data governance. By providing a clear view of data at all times, it makes it easier to enforce data policies and standards, ensuring compliance with regulations. Furthermore, it promotes transparency, as it allows stakeholders to see exactly how data is being used and managed.
Finally, data observability facilitates data-driven decision-making. By providing real-time insights into data, it enables businesses to make informed decisions quickly and confidently. This can lead to improved business performance and competitive advantage.
Implementing Predictive Data Quality and Observability
Implementing predictive data quality and observability requires a strategic approach. It involves several steps, including defining data quality metrics, setting up data observability systems, and training staff on data quality and observability practices.
First, organizations need to define what constitutes high-quality data. This involves setting data quality metrics, such as accuracy, completeness, consistency, and timeliness. These metrics serve as a benchmark for assessing data quality and guide the predictive data quality process.
Setting Up Data Observability Systems
Next, organizations need to set up data observability systems. This involves selecting and implementing data monitoring and visualization tools. These tools should provide real-time insights into data and be capable of tracking data throughout its lifecycle.
It's also important to integrate data observability systems with existing data infrastructure. This ensures that all data, regardless of its source, is monitored and visualized. It also enables seamless data flow, which is crucial for real-time data observability.
Training Staff
Finally, organizations need to train their staff on data quality and observability practices. This involves educating them on the importance of data quality, the role of predictive data quality and observability, and how to use data observability tools. Training should be ongoing to ensure that staff stay up-to-date with the latest data quality and observability practices and technologies.
In conclusion, predictive data quality and observability are crucial for any data-driven organization. They ensure that data is accurate, reliable, and up-to-date, enhancing the accuracy of data-driven decisions and strategies. By implementing predictive data quality and observability, organizations can improve their data management and governance, enhance their decision-making, and ultimately, drive business success.
You might also like
Get in Touch to Learn More
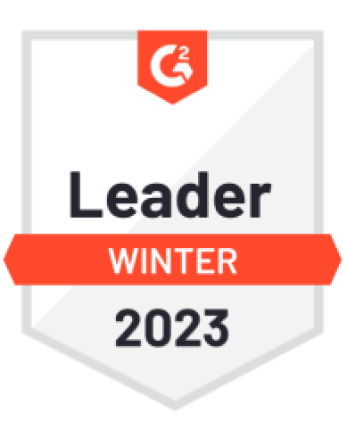
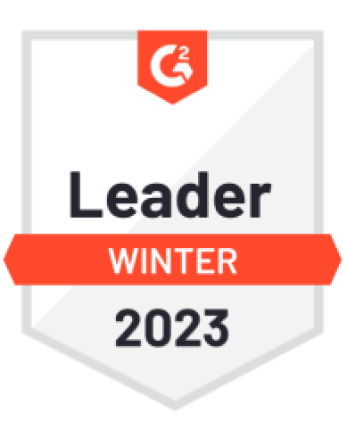

“[I like] The easy to use interface and the speed of finding the relevant assets that you're looking for in your database. I also really enjoy the score given to each table, [which] lets you prioritize the results of your queries by how often certain data is used.” - Michal P., Head of Data