The Different Types of Data Lineage
Explore the fascinating world of data lineage with this comprehensive article.

Data lineage is a critical concept in data management and plays a significant role in ensuring the reliability and accuracy of data. Understanding the different types of data lineage is essential for organizations to make informed decisions and mitigate potential risks associated with data management. This article aims to provide a comprehensive overview of the various types of data lineage, their definitions, importance, evolution, and future trends.
Understanding the Concept of Data Lineage
Data lineage refers to the ability to trace the origin, movement, and transformation of data throughout its lifecycle. It provides a detailed record of each data element's source, its transformations, and the destinations to which it is ultimately delivered. Data lineage helps organizations maintain data integrity, understand the data's context, and ensure compliance with regulatory requirements.
Definition and Importance of Data Lineage
Data lineage is crucial for organizations in today's data-driven world. It enables data professionals to have a clear understanding of where the data comes from, how it has been modified, and where it is used. This knowledge improves data quality, reduces data breaches, enhances decision-making, and promotes regulatory compliance.
Imagine a scenario where a financial institution is analyzing customer data to identify potential fraud. With data lineage, they can trace the origin of each data point, ensuring that it comes from a trusted source. They can also track the transformations applied to the data, such as aggregations or calculations, to ensure accuracy. By having a complete picture of the data's lineage, the institution can confidently make decisions based on reliable information, safeguarding their customers' interests.
Data lineage provides a transparent view of the data's lifecycle, allowing organizations to identify any potential data issues, such as inconsistencies, inaccuracies, or gaps. By understanding the data's lineage, organizations can quickly locate the source of an error or anomaly and rectify it, thus ensuring data reliability and trustworthiness. This level of visibility is particularly crucial in industries where data accuracy is paramount, such as healthcare or scientific research.
The Role of Data Lineage in Data Management
Data lineage is an integral part of data management processes. It helps organizations track and manage the flow of data, ensuring its accuracy and completeness. Data lineage provides insight into data dependencies and relationships, enabling organizations to identify the impact of changes made to data sources or transformations. This knowledge is essential when implementing changes to data structures, data integration projects, or data governance initiatives.
Consider a large retail company that decides to migrate its customer data to a new database system. Without data lineage, this process could be risky, as the company might lose track of the data's origin and its relationships with other datasets. However, with data lineage, the company can confidently plan and execute the migration, knowing exactly how the data is connected and what changes need to be made to ensure a seamless transition.
Furthermore, data lineage plays a vital role in data governance initiatives. It helps organizations establish data quality standards, define data ownership, and ensure compliance with data privacy regulations. By having a comprehensive understanding of the data's lineage, organizations can effectively manage data assets, mitigate risks, and maintain trust with stakeholders.
The Evolution of Data Lineage
Data lineage has evolved significantly over the years, primarily driven by advancements in technology and the increasing complexity of data environments. The manual documentation of data lineage has given way to automated tools and processes that streamline the tracking and management of data lineage.
As data continues to play a crucial role in decision-making processes across industries, the evolution of data lineage remains a key focus for organizations looking to ensure data quality, traceability, and compliance with regulations. The journey from manual to automated data lineage has not only improved efficiency but also enhanced data governance practices, providing organizations with a holistic view of their data ecosystem.
From Manual to Automated Data Lineage
In the past, data lineage was predominantly documented manually, relying on spreadsheets, diagrams, and documentation created by data professionals. However, as data volumes and complexity increased, manual methods became impractical and error-prone. Organizations started adopting automated data lineage tools that capture and visualize data lineage automatically. These tools extract metadata from various sources and provide a visual representation of the data flow, making it easier to understand and manage.
Automated data lineage tools not only offer a comprehensive view of data flow but also enable organizations to perform impact analysis, troubleshoot data quality issues, and ensure regulatory compliance more effectively. By automating the tracking of data lineage, organizations can save time and resources while mitigating the risks associated with data errors and inconsistencies.
The Impact of Technology on Data Lineage
The advancement of technology, such as cloud computing, big data platforms, and data integration tools, has had a profound impact on data lineage. These technologies have increased the volume, velocity, and variety of data, making it more challenging to track and manage data lineage manually. Consequently, automated data lineage tools have become essential for organizations to keep pace with the growing complexity of data ecosystems.
Furthermore, the integration of artificial intelligence and machine learning algorithms into data lineage tools has revolutionized the way organizations analyze and interpret data relationships. These advanced capabilities not only enhance data discovery and lineage mapping but also empower organizations to predict data lineage impact and optimize data management strategies proactively.
Types of Data Lineage
Data lineage can be classified into different types based on the aspects it focuses on. Each type serves a specific purpose in understanding the data's journey throughout its lifecycle. The three main types of data lineage are technical data lineage, operational data lineage, and business data lineage.
Technical Data Lineage
Technical data lineage focuses on the technical aspects of data, such as data sources, transformations, and destinations. It traces the data flow at a granular level, providing insights into data transformations, data quality checks, and data governance processes. Technical data lineage helps organizations ensure data accuracy, understand the impact of changes, and identify any potential bottlenecks or vulnerabilities in the data flow.
For example, let's consider a scenario where a company collects customer data from multiple sources, such as online forms, mobile applications, and third-party integrations. Technical data lineage would track the journey of this data, detailing how it is transformed and stored in the company's databases. It would provide information on any data cleansing or enrichment processes that take place, ensuring that the data is accurate and reliable for further analysis or reporting.
Operational Data Lineage
Operational data lineage focuses on the operational aspects of data, such as data movement and processing. It tracks the data flow across various systems, applications, and platforms, providing visibility into data movement and ensuring data consistency throughout the operational processes. Operational data lineage is crucial for organizations to monitor the data flow, identify operational inefficiencies, and optimize data processing.
Consider a scenario where a manufacturing company collects real-time sensor data from its production line. Operational data lineage would track how this data is captured, processed, and stored in the company's data infrastructure. It would provide insights into the systems and applications involved in the data flow, ensuring that the data is efficiently processed and available for real-time monitoring and analysis. Operational data lineage helps organizations identify any bottlenecks or delays in data processing, allowing them to take corrective actions and optimize their operational processes.
Business Data Lineage
Business data lineage focuses on the business aspects of data, such as data usage, data owners, and data governance policies. It helps organizations understand how data is used to support business processes, regulatory requirements, and analytical insights. Business data lineage enables organizations to establish data ownership, implement data access controls, and ensure compliance with data privacy regulations.
Imagine a scenario where a financial institution collects customer transaction data for fraud detection and regulatory reporting. Business data lineage would track how this data is used across different business units, such as risk management, compliance, and customer analytics. It would provide insights into the data owners responsible for maintaining the data's integrity and ensuring its compliance with regulatory requirements. Business data lineage helps organizations establish a clear understanding of data ownership, enabling them to implement appropriate data access controls and ensure compliance with data privacy regulations.
Choosing the Right Data Lineage for Your Business
Choosing the right data lineage for your business depends on several factors. It is essential to consider your organization's specific requirements, data environment, and goals. However, there are some common factors that organizations should consider when evaluating different data lineage solutions.
Factors to Consider
When selecting a data lineage solution, consider factors such as scalability, ease of implementation, integration capabilities, metadata management, data visualization, and reporting capabilities. It is crucial to ensure that the chosen solution aligns with your organization's IT infrastructure and data management strategy.
Potential Challenges and Solutions
Implementing a data lineage solution may come with challenges, such as data complexity, data governance practices, and organizational resistance to change. To overcome these challenges, organizations should invest in proper data governance practices, provide adequate training to users, and establish clear communication channels to address any concerns. It is also essential to monitor and evaluate the effectiveness of the implemented data lineage solution regularly.
The Future of Data Lineage
The future of data lineage is closely intertwined with emerging trends in data management, technology advancements, and regulatory requirements. To stay ahead in the data-driven landscape, organizations should be aware of these trends and adapt their data lineage strategies accordingly.
Emerging Trends in Data Lineage
One emerging trend in data lineage is the increasing adoption of metadata-driven approaches. Metadata-driven data lineage solutions leverage metadata repositories to capture and analyze data lineage information. These solutions provide a holistic view of data lineage across various systems, making it easier to manage and govern data effectively.
The Role of AI and Machine Learning in Data Lineage
Artificial Intelligence (AI) and Machine Learning (ML) technologies are transforming the data lineage landscape. AI and ML algorithms can analyze vast amounts of data and automatically identify data lineage patterns, relationships, and anomalies. This helps organizations improve data quality, detect data lineage issues, and make informed business decisions.
In conclusion, data lineage plays a crucial role in data management, ensuring data reliability, accuracy, and compliance. Organizations can benefit from understanding the different types of data lineage and choosing the right solution that aligns with their specific needs. As technology continues to advance and data environments become more complex, automating data lineage processes and leveraging metadata-driven approaches will become increasingly vital. Embracing emerging trends, such as AI and ML, will further enhance organizations' ability to manage data lineage effectively and gain valuable insights from their data.
You might also like
Get in Touch to Learn More
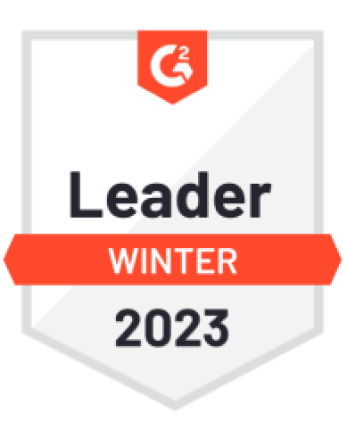
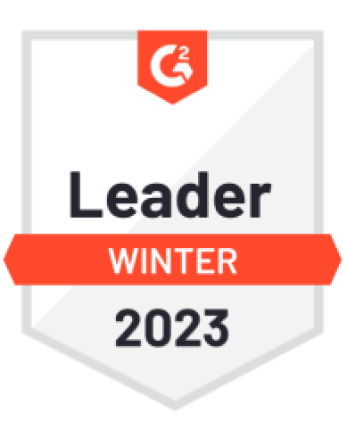

“[I like] The easy to use interface and the speed of finding the relevant assets that you're looking for in your database. I also really enjoy the score given to each table, [which] lets you prioritize the results of your queries by how often certain data is used.” - Michal P., Head of Data