The Ultimate Guide to Boosting ROI with Data Quality
Learn how to maximize your ROI through effective data quality strategies with this guide.
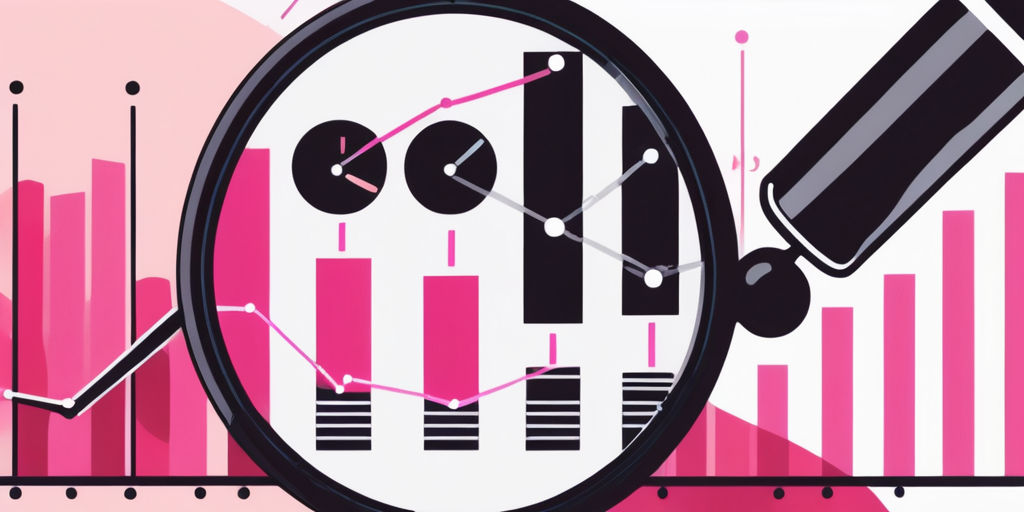
In today's data-driven world, organizations are constantly striving to maximize their return on investment (ROI). Yet, many fail to recognize the crucial role that data quality plays in achieving this goal. Data quality, defined as the accuracy, completeness, consistency, and reliability of data, is the foundation upon which successful businesses are built. Understanding the impact of data quality on ROI is essential for any organization looking to stay competitive in today's fast-paced market.
Understanding the Impact of Data Quality on ROI
At the core of the relationship between data quality and business success lies the notion that high-quality data leads to better decision-making. When an organization has access to accurate and reliable data, it can make informed strategic choices, identify market trends, and uncover hidden opportunities. On the other hand, poor data quality can lead to misguided decisions, ineffective marketing campaigns, and missed revenue-generating opportunities.
One of the key metrics for measuring ROI on data quality is the data accuracy rate. Accurate data ensures that organizations are targeting the right audience, delivering personalized marketing messages, and optimizing their customer experience. Additionally, data completeness, consistency, and reliability also contribute to the overall ROI by improving operational efficiency, reducing costs, and mitigating risks.
Furthermore, data quality plays a crucial role in enhancing customer satisfaction and loyalty. When businesses have clean and reliable data, they can provide personalized services, anticipate customer needs, and build long-lasting relationships. This leads to increased customer retention rates, higher customer lifetime value, and positive word-of-mouth referrals.
Another aspect to consider is the impact of data quality on regulatory compliance. In industries where data privacy and security are paramount, maintaining high data quality standards is essential to ensure compliance with laws and regulations. By having accurate and up-to-date data, organizations can avoid costly fines, legal issues, and reputational damage.
7 Proven Steps to Calculate ROI on Data Quality
Calculating the return on investment for data quality initiatives may seem like a daunting task, but with the right approach, it can be simplified into seven proven steps.
Implementing a Data Quality Measurement Framework
The first step towards calculating ROI on data quality is establishing a measurement framework. This framework should outline the key metrics, data quality goals, and data quality assessment methodologies specific to your organization. By aligning your data quality efforts with measurable objectives, you can effectively track progress and demonstrate tangible results.
Creating a robust data quality measurement framework involves identifying relevant KPIs (Key Performance Indicators) that reflect the impact of data quality on your business operations. These KPIs could include metrics like data accuracy rates, completeness levels, timeliness of data updates, and overall data consistency. By defining and tracking these KPIs, you can gain valuable insights into the effectiveness of your data quality initiatives over time.
Analyzing the Cost of Poor Data Quality
Understanding the cost implications of poor data quality is essential for calculating ROI. Assessing the direct and indirect costs associated with data inaccuracy, such as customer churn, marketing inefficiencies, and compliance penalties, provides a baseline for measuring the financial impact of data quality improvement initiatives.
In addition to quantifying the financial costs of poor data quality, it is important to consider the non-financial impacts as well. Poor data quality can lead to reputational damage, loss of customer trust, and missed business opportunities. By taking a holistic view of the consequences of data inaccuracy, organizations can better appreciate the true value of investing in data quality improvement efforts.
Demonstrating the Value of Data Quality Investments
Once you have established a measurement framework and analyzed the cost of poor data quality, it is crucial to demonstrate the value of data quality investments. This can be done by quantifying the benefits of data quality improvement initiatives, such as increased customer satisfaction, higher conversion rates, improved operational efficiency, and reduced error rates.
Furthermore, showcasing the positive impact of data quality enhancements on key business processes can help secure buy-in from stakeholders across the organization. By highlighting real-world examples of how improved data quality leads to better decision-making, streamlined operations, and enhanced customer experiences, you can build a compelling case for continued investment in data quality initiatives.
Uncovering the Financial Consequences of Poor Data Quality
Poor data quality can have substantial financial consequences for organizations. These consequences extend beyond the direct costs associated with data inaccuracies and encompass various hidden costs that are often overlooked.
The Hidden Costs of Data Inaccuracy
One of the hidden costs of data inaccuracy is the impact on customer trust and loyalty. When customers encounter inaccurate data, such as incorrect contact information or billing errors, it erodes their trust in the organization. This can lead to customer dissatisfaction, increased customer churn, and ultimately, lost revenue.
Imagine a scenario where a customer receives a promotional email with an incorrect name or address. Not only does this create a negative impression of the organization's attention to detail, but it also raises concerns about the security and privacy of their personal information. As a result, the customer may hesitate to engage with future marketing campaigns or even consider taking their business elsewhere.
Another financial consequence of poor data quality is the increased operational costs. Organizations with poor data quality often spend more time and resources on data cleansing, manual data entry, and rectifying data errors. These inefficiencies can result in higher labor costs, decreased productivity, and decreased overall profitability.
Consider the example of a company that relies on manual data entry for their sales process. If the data is riddled with errors, sales representatives may waste valuable time correcting mistakes or contacting the wrong leads. This not only hampers their ability to close deals but also diverts their attention from revenue-generating activities. The cumulative effect of these inefficiencies can be detrimental to the organization's financial health.
Mitigating Risks Associated with Poor Data Quality
In addition to the financial consequences, poor data quality also carries significant risks for organizations. These risks include compliance violations, data breaches, and reputational damage. Non-compliance penalties, legal fees, and the long-term impact on brand reputation can severely impact the bottom line.
Imagine a scenario where an organization fails to comply with data protection regulations and experiences a data breach. Apart from the immediate financial costs associated with investigating the breach and notifying affected individuals, the long-term consequences can be devastating. Customers may lose trust in the organization's ability to protect their data, leading to a decline in customer acquisition and retention. The resulting reputational damage can take years to repair and can have a lasting impact on the organization's financial wellbeing.
By investing in data quality improvement initiatives, organizations can mitigate these risks and safeguard their financial wellbeing. Implementing robust data governance frameworks, conducting regular data audits, and leveraging advanced data quality tools can help identify and rectify data inaccuracies before they escalate into costly problems. Furthermore, organizations that prioritize data quality demonstrate their commitment to excellence, which can enhance customer trust, loyalty, and ultimately, their bottom line.
Strategies to Enhance Data Quality for Optimal ROI
Enhancing data quality is a continuous process that requires a combination of best practices and leveraging technology. By implementing the following strategies, organizations can optimize their ROI on data quality.
Best Practices for Data Cleansing and Enrichment
Data cleansing and enrichment are essential steps towards improving data quality. By regularly cleansing data, organizations ensure that they maintain accurate and up-to-date information. Furthermore, enriching data with additional attributes, such as demographic information, purchase history, or social media profiles, provides valuable insights for targeted marketing and personalization efforts.
Leveraging Technology for Data Quality Improvement
The advancements in technology have revolutionized the field of data quality improvement. Organizations can leverage data quality tools and automated processes to identify, cleanse, and validate data. Additionally, implementing master data management (MDM) solutions allows organizations to establish a single source of truth, ensuring data consistency and eliminating data redundancies.
The Tangible Value of Enhanced Data Quality
While the benefits of data quality improvement may seem intangible at first, numerous real-life examples demonstrate its tangible value.
Real-Life Examples of ROI from Data Quality Initiatives
Company XYZ, a leading e-commerce retailer, implemented a data quality improvement initiative focused on cleaning and enriching customer data. The result was an increase in customer satisfaction, a 20% reduction in customer churn, and a 15% increase in revenue from personalized marketing campaigns. The ROI on the data quality initiative was calculated at 300% within the first year.
Another example is Company ABC, a multinational financial services firm. By implementing a comprehensive data quality measurement framework and leveraging technology for data validation and cleansing, they were able to eliminate duplicate customer records, improve compliance, and significantly reduce operational costs. The ROI on their data quality initiative surpassed 500% within two years.
Conclusion
Boosting ROI with data quality is not a luxury but a necessity for organizations seeking a competitive edge in today's data-driven marketplace. By understanding the impact of data quality on ROI, implementing a data quality measurement framework, and leveraging strategies to enhance data quality, organizations can unlock the tangible value of data quality improvement initiatives. The financial consequences of poor data quality can be severe, but with the right approach, organizations can mitigate risks, drive operational efficiency, and maximize their return on investment. Investing in data quality is an investment in success.
Ready to take the next step in enhancing your data quality and maximizing your ROI? Try CastorDoc today and experience the power of advanced governance, cataloging, and lineage capabilities combined with a user-friendly AI assistant. CastorDoc's robust data catalog and intuitive AI copilot are designed to streamline your data governance lifecycle, ensuring high-quality data and compliance with ease. Whether you're a data professional seeking control and visibility or a business user needing accessible and understandable data, CastorDoc is your partner in driving informed decision-making and achieving success in the data-driven world.
You might also like
Get in Touch to Learn More
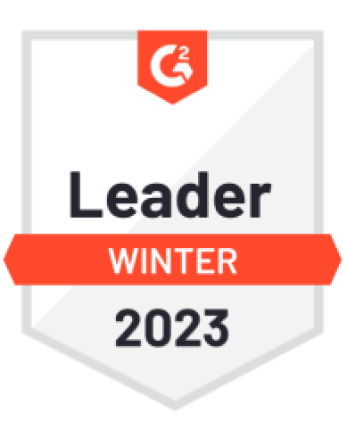
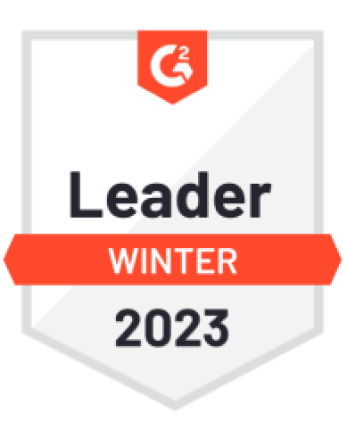

“[I like] The easy to use interface and the speed of finding the relevant assets that you're looking for in your database. I also really enjoy the score given to each table, [which] lets you prioritize the results of your queries by how often certain data is used.” - Michal P., Head of Data