What is dbt Semantic Layer for Metrics Definitions?
Discover the power of dbt Semantic Layer for Metrics Definitions in this comprehensive article.

In the world of data analytics, dbt (data build tool) Semantic Layer has emerged as a powerful framework for defining and managing metrics in a structured and efficient manner. This article aims to provide a comprehensive understanding of the basics, key components, functionality, benefits, and challenges associated with the dbt Semantic Layer specifically for metrics definitions.
Understanding the Basics of dbt Semantic Layer
The dbt Semantic Layer plays a critical role in transforming raw data into meaningful insights and measurable metrics. By employing a unified approach, it facilitates the seamless integration of data and enables organizations to define, organize, and manage metrics consistently across various analytical applications and reporting platforms.
Organizations leveraging the dbt Semantic Layer benefit from enhanced data governance and data quality, as the layer serves as a centralized hub for defining and maintaining key business metrics. This centralized approach ensures that all users are working with the same definitions and calculations, leading to improved data consistency and accuracy in decision-making processes.
The Role of dbt in Data Transformation
dbt, as a data transformation tool, assists in extracting data from different sources, transforming it according to business requirements, and loading it into a reliable destination for analysis. In conjunction with the Semantic Layer, dbt allows data engineers and analysts to collaborate effectively and streamline the data transformation process.
Furthermore, dbt's incremental processing capabilities enable organizations to efficiently process only the data that has changed since the last transformation, reducing processing time and improving overall data processing efficiency. This incremental approach is particularly beneficial for large datasets where processing all data from scratch would be time-consuming and resource-intensive.
Defining Semantic Layer in the Context of dbt
In the context of dbt, the Semantic Layer refers to a logical abstraction that sits on top of the data warehouse or data lake. It acts as a standardized layer that defines and documents metrics, calculations, definitions, and business rules, making them readily accessible and understandable to users across the organization.
Moreover, the Semantic Layer in dbt provides a level of abstraction that shields end-users from the complexities of underlying data structures and transformations. This abstraction simplifies the data consumption process, allowing business users to focus on deriving insights from data rather than worrying about the technical intricacies of data processing and transformation.
Key Components of dbt Semantic Layer
The dbt Semantic Layer comprises several essential components that collectively contribute to its effectiveness in managing metric definitions and facilitating data analysis.
Metrics Definitions in dbt Semantic Layer
The core component of the Semantic Layer is the metrics definitions. These definitions provide a clear and concise explanation of key performance indicators (KPIs), business metrics, and calculations. By documenting these metrics, data consumers gain a deeper understanding of how they are derived, leading to improved data literacy and decision-making.
The Importance of a Well-Structured Semantic Layer
In order to reap the benefits of the dbt Semantic Layer, it is essential to establish a well-structured foundation. This involves defining a clear taxonomy, establishing data governance and ownership, and ensuring consistent naming conventions and metadata management. A robust and organized Semantic Layer sets the stage for efficient data analysis and serves as a reliable source of truth for the entire organization.
Furthermore, a well-structured Semantic Layer enables scalability and flexibility in data modeling and analysis. As the organization grows and data complexity increases, having a solid foundation allows for easier integration of new data sources and metrics. This adaptability ensures that the Semantic Layer remains relevant and valuable in meeting evolving business needs.
Data Lineage and Impact Analysis
Another critical aspect of the dbt Semantic Layer is the ability to track data lineage and perform impact analysis. Understanding where data comes from, how it is transformed, and where it is used is essential for ensuring data accuracy and reliability. With robust data lineage capabilities, organizations can trace the journey of data from its source to its consumption, providing transparency and confidence in analytical outputs.
The Functionality of dbt Semantic Layer
The functionality provided by the dbt Semantic Layer goes beyond merely defining metrics. It empowers organizations with the ability to derive insights, analyze data, and make informed business decisions. Let's explore some key aspects of this functionality.
One of the significant advantages of utilizing the dbt Semantic Layer is its capacity to streamline data governance processes. By centralizing metric definitions and data transformations, organizations can ensure data quality and consistency throughout their analytics ecosystem. This centralized approach not only enhances data reliability but also simplifies the maintenance and management of data assets.
How dbt Semantic Layer Enhances Data Analysis
By leveraging the Semantic Layer, data analysts can access standardized metric definitions that are consistent across various reporting and analytical tools. This consistency minimizes discrepancies and ensures accurate and reliable analysis, enabling stakeholders to have confidence in the insights derived from the data.
Moreover, the dbt Semantic Layer promotes collaboration and knowledge sharing among data teams. By establishing a common language for defining metrics and data transformations, organizations can foster cross-functional collaboration and accelerate the development of data-driven solutions. This collaborative environment not only enhances productivity but also encourages innovation and continuous improvement in data analytics practices.
The Role of dbt Semantic Layer in Business Intelligence
Business intelligence (BI) heavily relies on data consistency and standardization. Through the Semantic Layer, dbt facilitates the creation of a unified view of metrics, thereby eliminating data silos and enabling seamless integration between BI tools and platforms. This integration empowers organizations to derive meaningful insights and drive data-informed decision-making.
Furthermore, the dbt Semantic Layer plays a crucial role in enhancing data governance and regulatory compliance efforts within organizations. By establishing clear data lineage and audit trails for metric definitions and transformations, dbt helps organizations meet regulatory requirements and maintain data integrity. This proactive approach not only mitigates risks associated with data misuse but also instills trust and transparency in data-driven decision-making processes.
The Benefits of Using dbt Semantic Layer for Metrics Definitions
Implementing dbt Semantic Layer for metrics definitions offers numerous benefits that foster data-driven cultures and improve the efficiency of data workflows.
When organizations adopt dbt Semantic Layer for defining metrics, they not only enhance data consistency but also pave the way for improved data governance. The centralized nature of the Semantic Layer ensures that all metrics are defined and calculated in a standardized manner, aligning with industry best practices and regulatory requirements. This standardized approach not only boosts trust in the data but also simplifies compliance with data privacy regulations such as GDPR and CCPA.
Improving Data Consistency with dbt Semantic Layer
Consistency is a crucial factor in any data-driven organization. By centralizing metric definitions and calculations in the Semantic Layer, dbt ensures that all stakeholders have access to the same definition and understanding of metrics. This eradicates the confusion and inconsistencies that can arise when different teams use their own definitions or calculations.
Furthermore, the dbt Semantic Layer acts as a single source of truth for metrics, eliminating the need for manual reconciliations across disparate data sources. This not only reduces the risk of errors in reporting but also accelerates decision-making processes by providing accurate and up-to-date information to stakeholders.
Streamlining Data Processes with dbt Semantic Layer
One of the primary advantages of the dbt Semantic Layer is its ability to streamline and automate data processes. By defining metrics once in the Semantic Layer, organizations can save time and effort when creating reports, dashboards, or visualizations. This streamlining of processes enables data practitioners to focus their efforts on analysis rather than repetitive data preparation tasks.
Moreover, the automation capabilities of the dbt Semantic Layer extend beyond just metric definitions. Organizations can leverage dbt to orchestrate complex data pipelines, perform data transformations, and ensure data quality, all within a unified environment. This holistic approach not only enhances operational efficiency but also empowers data teams to deliver insights at a faster pace, driving business agility and innovation.
Challenges and Solutions in Implementing dbt Semantic Layer
While the dbt Semantic Layer provides significant advantages for metrics definitions, its implementation can present challenges. Recognizing these challenges and having effective strategies in place to overcome them is crucial for successful adoption.
Common Issues in dbt Semantic Layer Implementation
During the implementation of the dbt Semantic Layer, some common challenges may arise. These can include difficulties in defining and aligning metrics across different teams, ensuring data accuracy and integrity, and addressing organizational resistance to change. Awareness of these challenges allows organizations to devise targeted solutions and strategies.
Effective Strategies for Overcoming Implementation Challenges
To ensure a successful implementation of the dbt Semantic Layer, organizations must employ effective strategies. These strategies can include strong stakeholder engagement, comprehensive training programs, clear communication, and an iterative approach towards refining and expanding the Semantic Layer. These approaches help organizations overcome potential hurdles and drive the adoption and success of the Semantic Layer.
Conclusion
In conclusion, the dbt Semantic Layer for metrics definitions offers a powerful solution for organizations seeking to standardize and manage their metrics effectively. By leveraging this framework, organizations can enhance data analysis, improve data consistency, streamline data processes, and ultimately make more informed decisions based on reliable and accurate metrics. Admittedly, the implementation of the dbt Semantic Layer may present challenges, but with careful planning, effective strategies, and a commitment to data excellence, organizations can overcome these hurdles and unlock the full potential of their data-driven initiatives.
You might also like
Get in Touch to Learn More
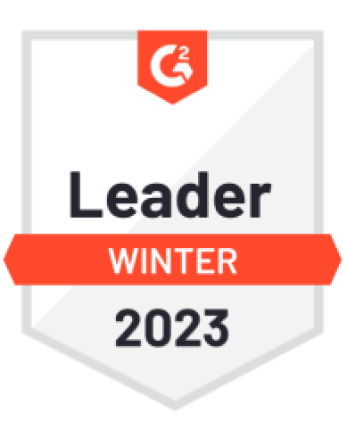
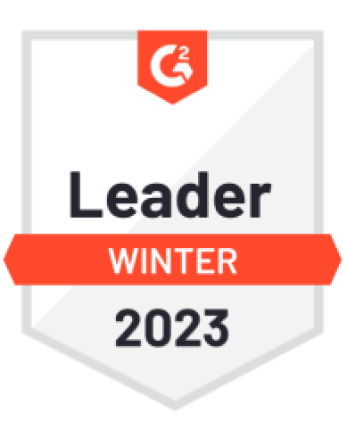

“[I like] The easy to use interface and the speed of finding the relevant assets that you're looking for in your database. I also really enjoy the score given to each table, [which] lets you prioritize the results of your queries by how often certain data is used.” - Michal P., Head of Data