What is the Cost of Bad Data?
Discover the hidden impact of bad data on your business's bottom line in this article.

The cost of bad data cannot be underestimated. Organizations heavily rely on data to make informed decisions, drive operational efficiency, and maintain strong customer relationships. However, bad data can have serious financial repercussions, impact business decisions, and even lead to compliance and legal issues. Understanding the concept of bad data is crucial to mitigate risks and enhance the overall data quality within an organization.
Understanding the Concept of Bad Data
Data is considered "bad" when it is inaccurate, incomplete, inconsistent, or outdated. Inaccurate data contains errors or mistakes, while incomplete data lacks necessary information. Inconsistent data differs across different sources or databases, making it difficult to align and analyze. Outdated data refers to information that is no longer relevant or up-to-date.
Defining Bad Data
To comprehend the cost of bad data, it is important to define what bad data entails. Bad data can range from incorrect customer information, such as misspelled names and outdated addresses, to faulty product or inventory data that may lead to inaccurate supply chain management and poor customer satisfaction. Additionally, bad data can also include duplicate records, data entry errors, and invalid or missing data fields.
Common Sources of Bad Data
Bad data can originate from various sources in an organization. One of the main sources is human error during data entry or data migration processes. Typos, incorrect formatting, and lack of validation measures contribute to the introduction of bad data. For example, a simple typo in a customer's email address can lead to failed communication attempts and missed opportunities for engagement.
Another source of bad data is data integration and system integration issues, where data from different systems or departments fails to align. Incomplete or inconsistent data merging can result in bad data. This can occur when different departments within an organization use different data formats or have varying data entry standards. For instance, if the sales department records customer addresses in a different format than the marketing department, it can lead to inconsistent and inaccurate data.
Furthermore, external data sources, such as data acquired from third-party vendors, can also introduce bad data if not properly vetted and validated. Organizations often rely on external data to supplement their own datasets, but if this data is not thoroughly checked for accuracy and reliability, it can contaminate the organization's database. For example, if a company purchases a mailing list from a vendor without verifying the quality of the data, they may end up with outdated or incorrect contact information.
The Financial Impact of Bad Data
Bad data can have substantial financial consequences for organizations. The direct costs of bad data include wasted resources spent on cleaning and correcting data, as well as potential legal costs resulting from non-compliance or lawsuits. Additionally, the effort and time required to fix bad data-related issues can divert valuable resources from core business activities, hampering productivity and profitability.
Moreover, bad data can also lead to missed revenue opportunities. Inaccurate customer information, for example, can result in failed marketing campaigns or ineffective sales strategies. This not only wastes resources but also hinders the organization's ability to capitalize on potential leads and conversions. Furthermore, bad data can impact customer satisfaction and retention, leading to a decline in repeat business and long-term profitability.
Direct Costs of Bad Data
The direct costs of bad data arise from the need to identify and rectify data issues. Organizations may need to allocate significant budgets for data cleansing and data quality tools to identify and correct inaccuracies, inconsistencies, and duplication. Furthermore, the cost of data clean-up projects, system enhancements, staff training, and data governance initiatives adds to the financial burden caused by bad data.
Indirect Costs of Bad Data
Indirect costs associated with bad data are not as apparent but can be equally significant. Inaccurate or incomplete data can lead to inefficient decision-making, resulting in missed business opportunities or poor operational performance. Delayed product launches, supply chain disruptions, and flawed marketing campaigns are just a few examples of the potential consequences. These indirect costs can have long-term negative effects on the organization's reputation and market competitiveness.
Furthermore, the impact of bad data on regulatory compliance should not be underestimated. Non-compliance with data protection regulations can result in hefty fines and legal penalties. Organizations that fail to maintain accurate and secure data risk damaging their reputation and losing the trust of customers and stakeholders. Implementing robust data governance practices and investing in data security measures are essential to mitigate these risks and ensure compliance with evolving data privacy laws.
How Bad Data Affects Business Decisions
Bad data has far-reaching implications for business decisions across all levels of an organization, from strategic planning to day-to-day operations. Understanding how bad data can affect decision-making is crucial to identify and mitigate risks associated with data quality.
It is important to note that the impact of bad data extends beyond just the strategic and operational aspects of a business. Bad data can also have significant implications for marketing decisions. Inaccurate customer information, flawed performance metrics, and unreliable market segmentation can lead to ineffective marketing campaigns and wasted resources. Moreover, bad data can distort customer behavior analysis, resulting in misguided targeting strategies and missed opportunities for customer engagement.
Impact on Strategic Decisions
Strategic decisions made based on bad data can lead to costly mistakes and missed opportunities. Inaccurate market research, faulty customer segmentation, and flawed trend analysis can result in misguided business strategies and a loss of competitive advantage. Furthermore, bad data can hinder accurate forecasting and budget planning, driving organizations towards inefficient resource allocation or missed revenue targets.
Additionally, the repercussions of bad data on strategic decisions can extend to long-term business sustainability. Misinformed strategic choices can weaken a company's position in the market, erode customer trust, and diminish brand reputation. This can have lasting effects on the organization's growth trajectory and overall success in a competitive business landscape.
Consequences for Operational Decisions
Operational decisions rely heavily on accurate and real-time data. Bad data can impede efficient inventory management, order processing, and supply chain operations. Mismatched product inventory, delayed shipments, and unsatisfied customers are just a few consequences that can arise from relying on bad data. Inefficient operational decisions, fueled by bad data, can lead to increased costs, reduced customer satisfaction, and ultimately damage the organization's bottom line.
Moreover, the impact of bad data on operational decisions can extend to employee productivity and morale. Inaccurate data used for workforce planning, scheduling, and performance evaluation can lead to confusion, frustration, and decreased motivation among employees. This can create a ripple effect throughout the organization, affecting overall operational efficiency and hindering the achievement of business goals.
Bad Data and Customer Relationships
Customer relationships are vital for the success and growth of any organization. Bad data can significantly impact customer perceptions and retention rates, ultimately affecting the bottom line.
Ensuring the accuracy and quality of data is crucial in today's data-driven business landscape. Organizations rely on data to understand their customers, personalize experiences, and make informed decisions. However, when bad data infiltrates systems, it can lead to a cascade of negative consequences that reverberate throughout the entire customer journey.
Influence on Customer Perception
Bad data can result in incorrect customer information being stored in databases, leading to a flawed understanding of customer preferences, behavior, and needs. This can result in targeting the wrong audience with ineffective marketing campaigns or offering irrelevant products or services. Inconsistent communication and messaging due to bad data can erode customer trust and damage the organization's reputation in the market.
Moreover, inaccurate data can distort customer segmentation efforts, causing businesses to misinterpret market trends and consumer behavior. This misalignment can lead to missed opportunities for growth and innovation, as organizations may base their strategies on flawed insights derived from unreliable data sources.
Effect on Customer Retention
Customer retention depends heavily on personalized and timely interactions. Bad data can hinder organizations' ability to provide a tailored customer experience. Duplicate communications, incorrect contact details, and outdated preferences can annoy customers, leading to churn and lost business opportunities. Furthermore, bad data can hinder customer service efforts, as representatives may not have accurate information readily available, resulting in frustrated customers and diminished loyalty.
Additionally, in today's hyper-competitive market landscape, where customer experience is a key differentiator, organizations cannot afford to overlook the impact of bad data on their ability to deliver seamless and personalized interactions. Customers expect businesses to anticipate their needs and preferences, and any misstep resulting from erroneous data can lead to a swift decline in customer satisfaction and advocacy.
The Role of Bad Data in Compliance and Legal Issues
Compliance with laws and regulations is critical for organizations operating in today's complex business environment. Bad data can pose serious compliance challenges and expose organizations to legal risks.
Compliance Challenges Due to Bad Data
Regulatory requirements demand accurate and up-to-date data in areas such as financial reporting, customer privacy, and data security. Failure to comply with these requirements due to bad data can result in penalties, fines, reputational damage, and even legal actions. Inadequate data controls, poor data governance practices, and insufficient data quality management increase the likelihood of non-compliance.
Legal Risks Associated with Bad Data
Bad data can lead to various legal risks, particularly concerning customer data privacy. Mishandling or exposing sensitive customer information due to bad data practices can result in violating privacy laws and regulations. Legal actions from affected customers or regulatory bodies can lead to significant financial repercussions, damage to brand reputation, and potential business closures. Proper data protection measures, including data quality management, are necessary to mitigate these legal risks.
Overall, the cost of bad data is immeasurable, both financially and operationally. Organizations must prioritize data quality initiatives, establish robust data governance practices, and invest in technologies and processes to minimize the risks associated with bad data. Failure to do so can have severe consequences, compromising the organization's competitive position, customer relationships, and compliance with legal obligations.
You might also like
Get in Touch to Learn More
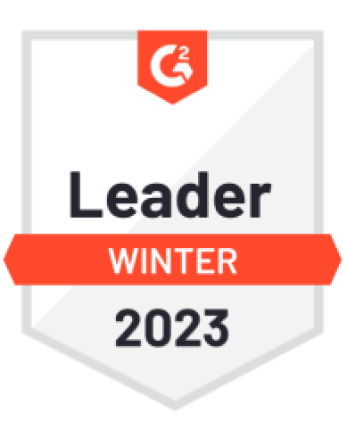
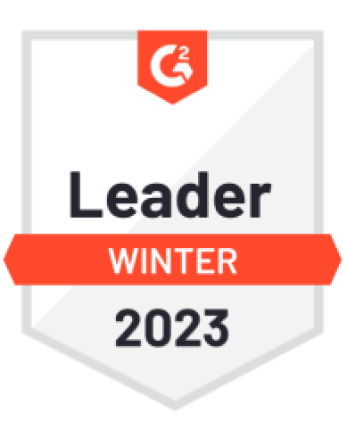

“[I like] The easy to use interface and the speed of finding the relevant assets that you're looking for in your database. I also really enjoy the score given to each table, [which] lets you prioritize the results of your queries by how often certain data is used.” - Michal P., Head of Data