Data Observability Tool Comparison: Soda vs. great expectations
In the world of data management and analytics, ensuring the quality and reliability of your data is paramount. That's where data observability tools come into play. These tools help you monitor, validate, and maintain the integrity of your data pipelines, so you can make informed decisions and trust the insights derived from your data. In this article, we will compare two popular data observability tools: Soda and Great Expectations.
Understanding Data Observability
Data observability refers to the practice of monitoring and verifying the quality and health of your data in real-time. It involves continuously collecting, processing, and analyzing data to detect anomalies, errors, or inconsistencies. By ensuring data observability, organizations can proactively identify and address issues, ensuring the accuracy and reliability of their data-driven insights.
The Importance of Data Observability
Data observability is crucial for several reasons. Firstly, it helps organizations maintain data integrity, ensuring that the data being used for analysis or decision-making is accurate and reliable. This is especially important in industries such as finance, healthcare, and e-commerce where even small errors can have significant consequences.
Secondly, data observability enables organizations to detect and address data quality issues in real-time. By monitoring data pipelines and identifying anomalies or inconsistencies, organizations can take immediate corrective action, preventing potential business disruptions and minimizing the impact on operations.
Lastly, data observability promotes transparency and trust in data-driven decision-making. When stakeholders have confidence in the accuracy and reliability of the data, they are more likely to trust the insights derived from it, leading to better decision-making and improved business outcomes.
Key Features of Data Observability Tools
Data observability tools offer a range of features aimed at monitoring, validating, and ensuring the quality of your data. These features may include:
- Data Monitoring: Tools provide real-time monitoring of data pipelines, enabling you to detect issues or anomalies as they occur.
- Data Validation: Tools offer various validation techniques to ensure that data meets predefined criteria, such as schema validation or business rule validation.
- Data Quality Metrics: Tools provide metrics and indicators to measure and track the quality of your data, allowing you to identify areas for improvement.
- Alerts and Notifications: Tools can send alerts and notifications when data anomalies or errors are detected, enabling proactive action.
- Data Lineage: Tools provide visibility into the origin and transformation of your data, allowing you to trace and understand data flows.
- Collaboration and Documentation: Tools offer features for collaboration and documentation, facilitating knowledge sharing and maintaining data observability practices.
Let's dive deeper into each of these key features to understand how they contribute to data observability:
1. Data Monitoring: Real-time monitoring of data pipelines allows organizations to keep a close eye on the health of their data. By continuously monitoring data as it flows through various stages, organizations can quickly identify any issues or anomalies that may arise. This proactive approach ensures that potential problems are detected and addressed before they can impact the accuracy and reliability of data-driven insights.
2. Data Validation: Data validation is a critical aspect of data observability. Tools that offer validation techniques help organizations ensure that the data being processed meets predefined criteria. This can include checking data against predefined schemas, verifying business rules, or validating data formats. By validating data, organizations can ensure that only high-quality and accurate data is used for analysis and decision-making.
3. Data Quality Metrics: Metrics and indicators provided by data observability tools allow organizations to measure and track the quality of their data. These metrics can include measures such as data completeness, accuracy, consistency, and timeliness. By monitoring these metrics, organizations can identify areas for improvement and take proactive steps to enhance data quality.
4. Alerts and Notifications: Data observability tools can send alerts and notifications when anomalies or errors are detected in the data. These alerts enable organizations to take immediate action, minimizing the impact of potential issues. By receiving real-time notifications, data teams can quickly investigate and resolve data quality issues, ensuring that data-driven insights are based on accurate and reliable information.
5. Data Lineage: Understanding the origin and transformation of data is crucial for data observability. Data lineage features provided by observability tools allow organizations to trace the journey of data from its source to its destination. This visibility into data flows helps organizations identify any potential bottlenecks, transformations, or data manipulations that may impact data quality. By understanding data lineage, organizations can ensure the integrity and reliability of their data throughout its lifecycle.
6. Collaboration and Documentation: Collaboration and documentation features offered by data observability tools facilitate knowledge sharing and the maintenance of data observability practices. These features allow data teams to collaborate effectively, share insights, and document best practices. By fostering collaboration and documentation, organizations can ensure that data observability practices are consistently followed, leading to improved data quality and better decision-making.
In conclusion, data observability is essential for organizations to maintain data integrity, detect and address data quality issues, and promote transparency and trust in data-driven decision-making. Data observability tools provide a range of features that enable organizations to monitor, validate, and ensure the quality of their data. By leveraging these tools and their key features, organizations can proactively manage their data, leading to more accurate insights and improved business outcomes.
An Introduction to Soda
Soda is a data observability tool that focuses on ensuring data quality and reliability. It offers a range of features designed to monitor, validate, and maintain the health of your data pipelines.
Overview of Soda's Functionality
Soda provides a user-friendly interface for monitoring data pipelines and detecting anomalies or errors. Its intuitive dashboard allows users to visualize data quality metrics and track the health of their data pipelines in real-time. Soda integrates seamlessly with popular data storage and processing platforms, making it easy to set up and configure.
One of Soda's key strengths is its extensive library of data validation rules. These rules allow users to define and enforce data quality requirements, ensuring that data meets specific criteria. Additionally, Soda provides built-in connectors for common data sources, making it easy to validate data from various systems and formats.
Pros and Cons of Using Soda
Like any tool, Soda has its strengths and limitations. Some pros of using Soda include:
- Intuitive User Interface: Soda's user-friendly interface makes it easy for users to navigate and monitor their data pipelines.
- Extensive Validation Rules Library: Soda provides a wide range of pre-defined validation rules, saving users time and effort in defining their own rules.
- Integration with Popular Data Platforms: Soda seamlessly integrates with popular data storage and processing platforms, ensuring compatibility with existing infrastructure.
However, it's essential to consider some potential cons when evaluating Soda:
- Limited Customization: While Soda offers a comprehensive set of validation rules, customization options may be limited for specific use cases.
- Cost: Soda's pricing structure may not be suitable for all budgets, especially for smaller organizations or startups.
An Introduction to Great Expectations
Great Expectations is another data observability tool that focuses on data quality assurance and validation. It offers a comprehensive suite of features to ensure the accuracy and reliability of your data pipelines.
Overview of Great Expectations' Functionality
Great Expectations provides a robust framework for defining and enforcing data expectations. Its flexible architecture allows users to create custom validation rules and metrics to suit their specific requirements. Great Expectations integrates seamlessly with popular data storage and processing platforms, making it easy to implement and maintain.
One of Great Expectations' notable features is its support for data documentation. It generates comprehensive data profiling reports and documentation, enabling users to understand and communicate the structure and expectations of their data.
Pros and Cons of Using Great Expectations
Great Expectations offers several advantages that make it a popular choice among data professionals. Some pros of using Great Expectations include:
- Customization and Flexibility: Great Expectations allows users to define custom validation rules and metrics, providing greater flexibility for complex data requirements.
- Data Documentation: Great Expectations generates detailed data profiling reports and documentation, facilitating data understanding and collaboration among stakeholders.
- Active Community and Support: Great Expectations has a vibrant community and offers comprehensive documentation and support resources, making it easier to get started and troubleshoot any issues.
However, there are a few considerations to keep in mind when evaluating Great Expectations:
- Learning Curve: Great Expectations may have a steeper learning curve for users unfamiliar with its concepts and terminology.
- Integration Challenges: While Great Expectations integrates well with popular data platforms, some integration challenges may arise when working with less common or custom data sources.
Detailed Comparison Between Soda and Great Expectations
Now that we've explored the key features and functionality of Soda and Great Expectations individually, let's dive deeper into their comparison across various aspects.
Comparing User Interface and Ease of Use
Soda's user interface is often praised for its simplicity and ease of use. Its intuitive dashboard and visualizations make it easy for users to monitor data pipelines and identify anomalies. Great Expectations, while offering a more comprehensive set of features, may have a steeper learning curve due to its flexibility and customizability.
Comparing Data Monitoring Capabilities
Both Soda and Great Expectations provide robust data monitoring capabilities. They allow users to set up real-time alerts and notifications for anomalies or errors, ensuring proactive detection and resolution. However, Great Expectations' extensive support for defining custom expectations and metrics gives users more control and flexibility in monitoring their data.
Comparing Data Validation Features
When it comes to data validation, both Soda and Great Expectations excel. Soda's extensive library of pre-defined validation rules and built-in connectors make it easy to validate data from various sources. Great Expectations, on the other hand, shines in its ability to define custom validation rules and metrics, providing greater flexibility for complex data requirements.
Comparing Integration and Compatibility
Both Soda and Great Expectations seamlessly integrate with popular data storage and processing platforms, ensuring compatibility with existing infrastructure. However, there may be instances where custom data sources or less common platforms require additional effort for integration. Users should evaluate compatibility based on their specific ecosystem and requirements.
Pricing: Soda vs. Great Expectations
Understanding the pricing structures of Soda and Great Expectations is essential when making a decision.
Understanding Soda's Pricing Structure
Soda offers a tiered pricing structure based on usage and additional features required. Pricing plans typically include different levels of data monitoring, validation, and support services. Users should assess their needs and budget to choose the most suitable pricing plan.
Understanding Great Expectations' Pricing Structure
Great Expectations follows an open-source community edition model, allowing users to access and use the tool for free. Additionally, Great Expectations offers enterprise-level support and additional features through a paid subscription plan. Organizations should evaluate their support requirements and the value of the additional features before opting for a paid plan.
Conclusion
Soda and Great Expectations are both powerful data observability tools that can help organizations ensure the quality and reliability of their data. While Soda offers an intuitive interface and a comprehensive library of validation rules, Great Expectations provides greater flexibility and customization options. Ultimately, the choice between these tools depends on specific business requirements, integration preferences, and budget considerations. By selecting the right data observability tool, organizations can drive better decision-making, improve data quality, and maintain a solid foundation for their data-driven initiatives.
While tools like Soda and Great Expectations are pivotal in ensuring data quality and observability, integrating them with a comprehensive governance platform like CastorDoc can elevate your data management to new heights. CastorDoc's advanced cataloging, lineage capabilities, and user-friendly AI assistant create a seamless environment for self-service analytics. Whether you're looking to streamline data governance, enhance regulatory compliance, or empower business users through intuitive data accessibility, CastorDoc is your go-to solution. Ready to explore how CastorDoc complements these tools and revolutionizes data management? Check out more tools comparisons here and discover the full potential of your data with CastorDoc.
You might also like
Get in Touch to Learn More
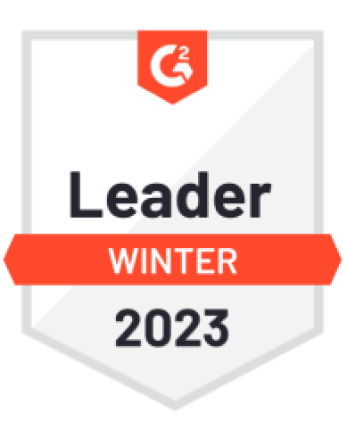
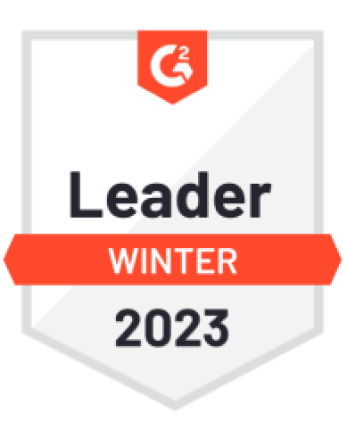

“[I like] The easy to use interface and the speed of finding the relevant assets that you're looking for in your database. I also really enjoy the score given to each table, [which] lets you prioritize the results of your queries by how often certain data is used.” - Michal P., Head of Data