Data Observability Tool Comparison: great expectations vs. Lightup
Data observability is a critical aspect of any data-driven organization. It ensures that the data being used for analysis and decision-making is accurate, reliable, and trustworthy. In this article, we will compare two popular data observability tools: Great Expectations and Lightup. We will explore the key components of data observability, the features and drawbacks of both tools, and provide a detailed comparison to help you choose the right tool for your needs.
Understanding Data Observability
Data observability is the process of monitoring, measuring, and ensuring the quality and integrity of data. It involves continuously assessing data for accuracy, completeness, and consistency. The goal is to identify and resolve issues such as data discrepancies, anomalies, and data quality problems. With data observability, organizations can trust that their data is reliable and make informed decisions based on it.
The Importance of Data Observability
Data is the lifeblood of modern organizations. It drives decision-making, improves operational efficiency, and enables innovation. However, data is prone to errors, inconsistencies, and biases. Without proper observability, organizations risk making decisions based on faulty or incomplete data, which can have severe consequences.
Data observability ensures that the data used for analysis and decision-making is accurate, complete, and reliable. It enables organizations to detect and resolve data issues in real-time, preventing costly mistakes and incorrect insights. With data observability, organizations can have confidence in their data and trust that it is providing reliable information.
Key Components of Data Observability
Data observability comprises various components that work together to ensure the quality and integrity of data:
- Monitoring: Continuous monitoring of data pipelines, processes, and systems to identify any deviations, anomalies, or errors.
- Validation: Verifying data against predefined rules, expectations, or constraints to ensure its accuracy, completeness, and consistency.
- Alerting: Sending real-time alerts or notifications when data quality issues or anomalies are detected, allowing for immediate action to be taken.
- Documentation: Documenting data expectations, assumptions, and dependencies to create a shared understanding among data consumers and stakeholders.
- Testing: Running automated tests to validate data quality, identify issues, and ensure the correctness of data transformations and calculations.
- Visualization: Visualizing data quality metrics, anomalies, and trends to provide a clear and intuitive view of data health.
- Collaboration: Facilitating collaboration and communication among data engineers, data scientists, and business stakeholders to address data issues and improve data quality.
Introduction to Great Expectations
Great Expectations is a widely used open-source data observability tool. It provides a framework for defining, managing, and validating data expectations. Great Expectations allows data teams to define rules or expectations about the structure, type, range, and quality of data. These expectations can be applied to different data sources, including relational databases, CSV files, and big data platforms.
Features of Great Expectations
Great Expectations offers a range of features that make it a powerful tool for data observability:
- Expectation Library: Great Expectations provides a rich library of predefined expectations that can be easily customized to fit specific data requirements.
- Data Profiling: It enables comprehensive data profiling and statistical analysis to understand data distributions, uniqueness, and missing values.
- Data Documentation: Great Expectations automatically generates data documentation, including data dictionaries and data lineage, making it easier to understand and collaborate on data.
- Data Validation: It allows users to define and validate data against custom rules, ensuring data integrity and quality.
- Continuous Integration: Great Expectations integrates with popular CI/CD tools, enabling automated data validation and monitoring.
Pros and Cons of Great Expectations
Like any tool, Great Expectations has its pros and cons that you should consider:
Pros:
- Open-source and community-driven, offering flexibility and extensive support.
- Easy to integrate with existing data pipelines and workflows.
- Provides a wide range of predefined expectations and customizable rules.
- Offers comprehensive data profiling and documentation capabilities.
Cons:
- Requires technical expertise to set up and configure initially.
- Steep learning curve, especially for users new to data observability concepts.
- Limited support for certain data sources and platforms.
Introduction to Lightup
Lightup is a data observability platform designed to help organizations monitor and validate the quality of their data pipelines. It provides a centralized dashboard for tracking data issues, identifying anomalies, and improving data quality. Lightup supports a variety of data sources, including relational databases and cloud-based data warehouses.
Features of Lightup
Lightup offers several key features that make it a compelling choice for data observability:
- Live Monitoring: Lightup provides real-time monitoring of data pipelines, generating alerts and notifications when data issues or anomalies are detected.
- Data Validation: It allows users to define validation rules and checks to ensure the quality and correctness of data.
- Customizable Dashboards: Lightup offers customizable dashboards and visualizations to track data quality, trends, and anomalies.
- Collaboration Tools: It facilitates collaboration among data teams, enabling efficient communication and issue resolution.
- Automated Remediation: Lightup can automatically trigger remediation actions, such as rerunning failed pipelines or notifying relevant stakeholders.
Pros and Cons of Lightup
Let's take a look at the pros and cons of using Lightup:
Pros:
- User-friendly interface, making it accessible to both technical and non-technical users.
- Offers comprehensive data monitoring and alerting capabilities.
- Enables collaboration and issue resolution within the platform.
- Provides customizable dashboards for visualizing data quality metrics.
Cons:
- May require additional setup and configuration to integrate with certain data sources and platforms.
- Less flexibility compared to open-source solutions like Great Expectations.
- Limited customization options for defining validation rules and expectations.
Detailed Comparison Between Great Expectations and Lightup
Comparison of Key Features
Both Great Expectations and Lightup offer a range of features that contribute to data observability. Let's compare some of their key features:
Great Expectations | Lightup | |
---|---|---|
Validation | ✓ | ✓ |
Data Profiling | ✓ | - |
Data Documentation | ✓ | - |
Continuous Integration | ✓ | - |
Real-time Monitoring | - | ✓ |
Customizable Dashboards | - | ✓ |
While Great Expectations offers more flexibility and extensibility with its open-source nature, Lightup provides a user-friendly interface and real-time monitoring capabilities.
Comparison of User Experience
When it comes to user experience, Great Expectations and Lightup offer different approaches:
Great Expectations:
Great Expectations requires a certain level of technical expertise and familiarity with data observability concepts. It offers powerful customization options but has a steeper learning curve.
Lightup:
Lightup aims to provide an intuitive and user-friendly experience, making it accessible to both technical and non-technical users. Its interface and visualizations are designed to simplify the process of monitoring and improving data quality.
Comparison of Performance
Both Great Expectations and Lightup aim to deliver reliable performance for data observability. The performance can vary depending on various factors, such as the complexity of data expectations, the scale of data pipelines, and the underlying infrastructure.
Great Expectations, being an open-source tool, offers flexibility to optimize and fine-tune performance according to specific requirements. Lightup, on the other hand, provides a cloud-based platform that manages the infrastructure and scaling requirements, relieving organizations of the need to manage their own infrastructure.
Choosing the Right Data Observability Tool
Factors to Consider
When choosing between Great Expectations and Lightup, consider the following factors:
- Technical Expertise: Assess the technical skills and familiarity of your team with data observability concepts and tools.
- Customization Requirements: Determine the level of customization and extensibility needed for your data expectations and validation rules.
- Data Source Compatibility: Check if the tool supports your existing data sources and platforms.
- User Interface and Experience: Evaluate the user interface and experience of each tool to ensure it aligns with your team's requirements and capabilities.
- Integration and Ecosystem: Consider the integration options and ecosystem of each tool, such as compatibility with existing data pipelines, frameworks, and tools.
- Scalability and Performance: Assess the scalability and performance requirements of your data observability needs and how each tool caters to them.
- Cost and Licensing: Consider the cost implications and licensing requirements of each tool.
Making the Decision
The decision between Great Expectations and Lightup ultimately depends on your specific requirements and constraints. Both tools offer valuable capabilities for data observability, but they differ in terms of flexibility, user experience, and compatibility. Consider conducting a proof of concept or trial evaluation to assess each tool's fit for your organization before making a final decision.
In conclusion, data observability is a crucial aspect of any data-driven organization. By comparing Great Expectations and Lightup, we have explored the key components, features, and drawbacks of each tool. Remember to consider factors such as technical expertise, customization requirements, and user experience when making your decision. Choose the tool that best aligns with your organization's goals and requirements, and invest in data observability to ensure the quality and reliability of your data.
As you consider the right data observability tool for your organization, remember that the journey doesn't end there. With CastorDoc, you can take your data management to the next level. CastorDoc's advanced governance, cataloging, and lineage capabilities, combined with a user-friendly AI assistant, provide a powerful platform for businesses to enable self-service analytics. It's designed to help data teams maintain control and visibility while empowering business users to find and utilize data with confidence. Ready to revolutionize how your organization manages and leverages data? Check out more tools comparisons here and discover how CastorDoc can complement your data observability and governance strategy.
You might also like
Get in Touch to Learn More
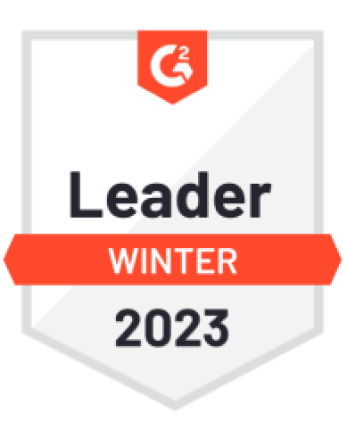
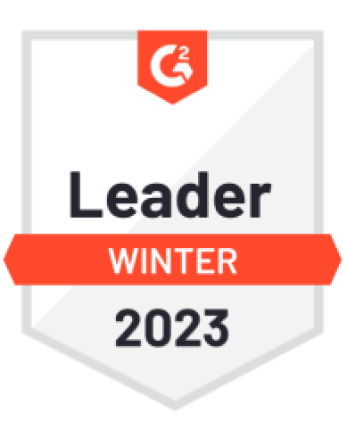

“[I like] The easy to use interface and the speed of finding the relevant assets that you're looking for in your database. I also really enjoy the score given to each table, [which] lets you prioritize the results of your queries by how often certain data is used.” - Michal P., Head of Data