Conversational Analytics: Transforming Data Interaction through Natural Language
Discover how conversational analytics is revolutionizing the way we interact with data through natural language.
The ability to effectively interact with and derive insights from vast amounts of information is paramount for businesses. Traditional methods of data interaction, such as manual analysis and structured queries, are time-consuming and often inaccessible to non-technical users. However, with the advent of conversational analytics, the landscape is rapidly changing. By leveraging natural language processing (NLP) technologies, conversational analytics enables users to access and analyze data through simple, human-like conversations. In this article, we will explore the definition, importance, and key components of conversational analytics, as well as its transformational impact on data interaction. We will also delve into the future of this exciting field, challenges faced during implementation, and effective solutions for successful adoption.
Understanding Conversational Analytics
Conversational analytics can be broadly defined as the use of natural language to interact with and analyze complex datasets. The ability to communicate with machines in a conversational manner opens up new possibilities for businesses across various industries. By providing a user-friendly interface, conversational analytics democratizes access to data, allowing both technical and non-technical users to obtain insights effortlessly. Moreover, it eliminates the need for extensive training or knowledge of query languages, making data exploration and analysis more accessible to a broader audience.
Definition and Importance of Conversational Analytics
At its core, conversational analytics is all about transforming traditional data interaction by harnessing the power of natural language. It leverages NLP algorithms to understand and interpret user queries, enabling users to request data and receive actionable insights through a conversation-like interface. The importance of conversational analytics lies in its ability to bridge the gap between humans and machines, allowing for a more intuitive and efficient interaction with data. By enabling users to speak or type in plain language, conversational analytics reduces the cognitive load associated with traditional data interaction methods, promoting faster and more meaningful insights.
The Role of Natural Language Processing in Conversational Analytics
Natural Language Processing plays a pivotal role in enabling conversational analytics. NLP algorithms analyze and understand the context, syntax, and semantics of user queries, allowing machines to respond intelligently. By employing techniques such as entity recognition, sentiment analysis, and named entity recognition, NLP enables conversational analytics platforms to extract relevant information from unstructured data and provide dynamic responses to user queries. This sophisticated processing of language data is what differentiates conversational analytics from traditional data interaction methods, enabling users to engage in productive and efficient conversations with their data.
One of the key benefits of natural language processing in conversational analytics is its ability to handle complex queries. Traditional data interaction methods often require users to have a deep understanding of query languages and database structures. This can be a significant barrier for non-technical users who may not have the necessary skills or knowledge. However, with conversational analytics powered by NLP, users can simply express their data needs in natural language, without having to worry about the technicalities behind the scenes. This empowers a wider range of users to explore and analyze data, leading to more diverse insights and perspectives.
Furthermore, natural language processing in conversational analytics enables the extraction of valuable insights from unstructured data. Unstructured data, such as text documents, social media posts, and customer reviews, often contains valuable information that can provide a deeper understanding of customer sentiment, market trends, and emerging patterns. By applying NLP techniques, conversational analytics platforms can analyze and interpret this unstructured data, uncovering hidden patterns and relationships that may not be immediately apparent. This allows businesses to gain a more comprehensive and holistic view of their data, leading to more informed decision-making and strategic planning.
The Transformation of Data Interaction
The advent of conversational analytics represents a significant shift in how users interact with data. Gone are the days of complex queries and manual data exploration. Instead, users can now have meaningful conversations with data, enabling faster decision-making and deeper insights.
Traditional Data Interaction Vs. Conversational Analytics
Traditional data interaction methods, such as writing SQL queries or using business intelligence tools, require users to have a strong understanding of databases, query languages, and data structures. This poses a barrier to entry for non-technical users, limiting their ability to explore and derive insights from data.
However, with the rise of conversational analytics, this barrier is being torn down. Imagine a scenario where a marketing manager wants to analyze the performance of a recent email campaign. In the traditional approach, they would need to write complex SQL queries to extract the relevant data, manipulate it, and then generate reports. This process could take hours or even days, delaying critical decision-making.
Conversational analytics, on the other hand, simplifies the interaction process by allowing users to express their queries in natural language. Instead of writing code, the marketing manager can simply ask questions like, "What was the open rate of our last email campaign?" or "Which demographic segment had the highest click-through rate?" The system understands the intent behind these questions and retrieves the relevant data, presenting it in an easily digestible format.
The Impact of Conversational Analytics on Data Interaction
The impact of conversational analytics on data interaction cannot be understated. By enabling users to interact with data in a conversational manner, the barriers between humans and machines are dismantled. Users are no longer limited by their technical skills or the constraints of query languages.
Moreover, conversational analytics opens up a world of possibilities for collaboration and decision-making within organizations. Imagine a team meeting where stakeholders from different departments come together to discuss a new product launch. In the past, these meetings would often be dominated by technical experts who had the ability to extract and analyze data. Non-technical team members would have to rely on their expertise and interpretation of the data presented.
With conversational analytics, everyone in the room can actively participate in the data-driven discussion. They can ask questions, explore different scenarios, and gain insights in real-time. This not only enhances collaboration but also ensures that decisions are based on accurate and up-to-date information.
Furthermore, conversational analytics breaks down silos between technical and non-technical teams. It fosters a data-driven culture where everyone has the opportunity to engage with and analyze data independently. This democratization of data empowers individuals at all levels of an organization to make informed decisions and drive innovation.
Key Components of Conversational Analytics
Conversational analytics comprises several key components that work together to facilitate seamless data interaction.
Natural Language Understanding in Conversational Analytics
At the heart of conversational analytics lies natural language understanding (NLU). NLU algorithms enable machines to comprehend human language by deciphering the meaning, intent, and context of user queries. By utilizing techniques such as machine learning, deep learning, and semantic analysis, NLU algorithms empower conversational analytics platforms to extract insights from user queries and provide relevant responses. This ability to understand and respond to user input in a natural and meaningful way is what sets conversational analytics apart from traditional query-based approaches.
Speech Recognition and its Role in Conversational Analytics
While text-based interactions are prevalent in conversational analytics, speech recognition is also an important component of the technology. Speech recognition algorithms convert spoken language into text form, enabling users to engage in verbal conversations with their data. This functionality is especially beneficial for users on-the-go or those who prefer a hands-free experience. By combining speech recognition with NLP capabilities, conversational analytics platforms provide users with a comprehensive and multimodal interaction with their data.
The Future of Conversational Analytics
The future of conversational analytics is an exciting one, with numerous trends poised to shape the industry and revolutionize data interaction.
Predicted Trends in Conversational Analytics
As conversational analytics continues to evolve, several trends are expected to take center stage. One notable trend is the integration of conversational analytics with voice assistants and smart home devices. This convergence will enable users to effortlessly access and analyze data through voice commands, further blurring the line between human and machine interaction. Moreover, advancements in NLP and machine learning will enhance the understanding and context-awareness of conversational analytics platforms, enabling more nuanced and insightful responses to user queries.
The Potential Impact of Conversational Analytics on Various Industries
Conversational analytics has the potential to revolutionize various industries by simplifying data interaction and driving data-driven decision-making. In the healthcare sector, for example, conversational analytics can enable clinicians to easily access patient records, extract relevant medical insights, and make informed treatment decisions. In the retail industry, conversational analytics can help businesses gain a deep understanding of customer preferences, optimize pricing strategies, and deliver personalized shopping experiences. These are just a few examples of the transformative impact that conversational analytics can have on industries across the board.
Challenges and Solutions in Implementing Conversational Analytics
Despite the significant benefits, implementing conversational analytics can present challenges that organizations need to address to ensure successful adoption.
Common Challenges in Adopting Conversational Analytics
One common challenge is the complexity of developing and training conversational analytics models. NLP algorithms require large amounts of data and extensive computational resources for training, making it challenging for organizations to embark on their conversational analytics journey. Additionally, ensuring data privacy and security while engaging in conversational analytics poses another challenge, as organizations must handle and protect sensitive information appropriately.
Effective Solutions for Implementing Conversational Analytics
To overcome these challenges, organizations can leverage cloud-based platforms and services that provide ready-to-use conversational analytics capabilities. These platforms offer pre-trained models, eliminating the need for extensive development and training efforts. Additionally, organizations should implement robust data governance and security measures to safeguard the confidentiality and integrity of their data. By partnering with trusted providers and investing in appropriate infrastructure, organizations can overcome implementation challenges and unlock the full potential of conversational analytics.
In conclusion, conversational analytics is revolutionizing the way we interact with data. By leveraging natural language processing technologies, this innovative approach enables users to engage in conversations with their data, opening up new possibilities for analysis and insights. As the field continues to evolve, the future holds exciting trends and transformative potential for various industries. However, organizations must navigate the challenges associated with implementation and ensure the security and privacy of their data. By embracing conversational analytics and leveraging its key components, organizations can unleash the power of natural language and transform data interaction into a seamless and intuitive experience.
Ready to harness the power of conversational analytics and transform how your business interacts with data? CastorDoc is here to guide you into the future of data-driven decision-making. Our AI Agent for Analytics is designed to provide your teams with instant, reliable data answers, enabling self-service analytics and fostering a culture of data literacy. With CastorDoc, you can maximize the ROI of your data stack and give your business users the autonomy and trust they need to leverage data effectively. Don't let the complexities of data analysis hold you back. Try CastorDoc today and experience the ease of conversational analytics for your strategic business challenges.
You might also like
Get in Touch to Learn More
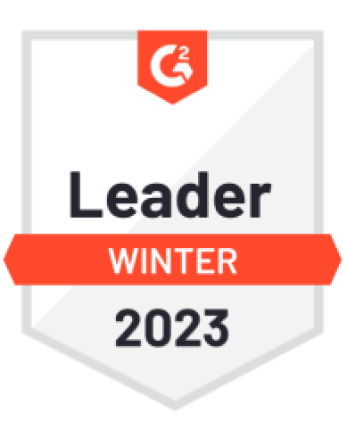
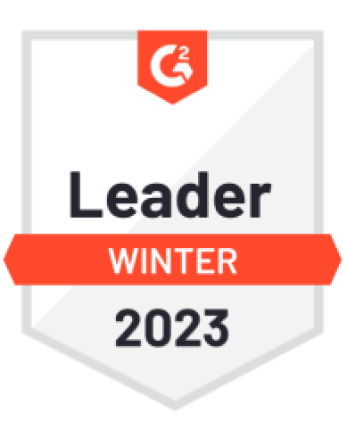

“[I like] The easy to use interface and the speed of finding the relevant assets that you're looking for in your database. I also really enjoy the score given to each table, [which] lets you prioritize the results of your queries by how often certain data is used.” - Michal P., Head of Data