Exploring Multi-Agent LLM Systems for Complex Solutions
Discover the power of multi-agent LLM systems in tackling complex problems.
Today, the need for efficient and effective solutions has become paramount. Organizations across various industries are constantly seeking ways to enhance their problem-solving capabilities and optimize their operations. One such avenue that has gained significant attention is the use of Multi-Agent LLM (Learning, Logic, and Optimization) systems.
Understanding Multi-Agent LLM Systems
Definition and Function of LLM Systems
At its core, an LLM system combines learning algorithms with mathematical optimization techniques to tackle complex problems. It leverages the power of logic and reasoning to find the most optimal solutions in a given scenario. These systems are designed to adapt and improve their performance over time, making them invaluable tools for decision-making in dynamic environments.
One of the key strengths of LLM systems lies in their ability to handle uncertainty and ambiguity inherent in real-world problems. By incorporating probabilistic reasoning and machine learning algorithms, these systems can make informed decisions even in situations with incomplete information. This adaptability is crucial for applications in fields such as autonomous vehicles, healthcare diagnostics, and financial forecasting.
The Role of Multi-Agent Systems in LLM
In the context of LLM systems, the term "multi-agent" refers to the use of multiple intelligent agents working collaboratively towards a common goal. Each agent in the system possesses a specialized knowledge or expertise, and their combined efforts enable the system to handle intricate challenges more effectively. By harnessing the collective intelligence and diverse perspectives of these agents, multi-agent LLM systems can achieve superior results.
Furthermore, the decentralized nature of multi-agent systems allows for scalability and robustness in complex environments. Unlike traditional centralized systems, where a single point of failure can disrupt the entire operation, multi-agent LLM systems distribute the workload among agents, reducing the risk of system-wide failures. This distributed approach also enhances the system's flexibility, as new agents can be easily added or removed to adapt to changing requirements or environments.
The Architecture of Multi-Agent LLM Systems
Key Components of Multi-Agent LLM Systems
A multi-agent LLM system comprises several key components that work in synergy to achieve optimal outcomes. The first essential element is the learning component, which enables the system to acquire knowledge from data and past experiences. The logical component handles reasoning and inference, allowing the system to make informed decisions. Lastly, the optimization component employs mathematical techniques to find the most suitable solutions based on predefined criteria.
Furthermore, within the learning component, various machine learning algorithms such as supervised learning, unsupervised learning, and reinforcement learning can be utilized to train the agents within the system. These algorithms enable the agents to recognize patterns, make predictions, and continuously improve their decision-making processes based on feedback received.
Designing Efficient Multi-Agent LLM Systems
Designing an efficient multi-agent LLM system requires careful consideration of various factors. Firstly, the system architecture should foster effective communication and coordination among the agents. This entails establishing clear channels for information exchange and defining protocols for collaborative decision-making. Additionally, the learning algorithms should be designed to adapt and evolve as the system encounters new challenges and receives feedback from its environment. Finally, by optimizing the computational resources and distribution of tasks, the performance and scalability of the system can be significantly enhanced.
Moreover, when designing the communication channels for multi-agent systems, it is crucial to consider the security and privacy of data exchanged between agents. Implementing encryption techniques and access control mechanisms can help safeguard sensitive information and prevent unauthorized access. Furthermore, incorporating mechanisms for conflict resolution and consensus building among agents can enhance the overall efficiency and effectiveness of decision-making processes within the system.
Advantages of Using Multi-Agent LLM Systems
Enhancing Problem-Solving Capabilities
One of the primary benefits of utilizing multi-agent LLM systems is their ability to tackle complex problems with a high degree of efficiency. By leveraging the expertise of multiple intelligent agents, these systems can analyze vast amounts of data and generate solutions that would be beyond the capacity of a single agent or traditional approaches. The collaborative nature of multi-agent systems allows for a more comprehensive understanding of the problem at hand, resulting in innovative solutions and improved decision-making.
Furthermore, the use of multi-agent LLM systems promotes diversity in problem-solving approaches. Each agent within the system may possess unique algorithms, heuristics, or learning mechanisms, contributing to a rich pool of strategies for addressing complex issues. This diversity not only enhances the robustness of the system but also encourages creativity and exploration of unconventional solutions.
Improving System Flexibility and Scalability
Traditional systems often struggle to adapt to changing requirements and dynamic environments. However, multi-agent LLM systems excel in this regard. Their inherent flexibility enables them to continuously learn and adapt, ensuring that they remain effective even as circumstances evolve. Moreover, these systems can be easily scaled by adding or removing agents as needed, allowing organizations to optimize their resources and address increasing complexity.
In addition to their adaptability, multi-agent LLM systems exhibit self-organization capabilities, where agents can autonomously reconfigure themselves to better suit the current task or environment. This self-organizing behavior not only enhances system efficiency but also reduces the need for manual intervention in system management, freeing up human resources for more strategic tasks.
Challenges in Implementing Multi-Agent LLM Systems
Dealing with System Complexity
Implementing multi-agent LLM systems presents challenges due to the inherent complexity involved. Coordinating the actions and information exchange among multiple agents within the system can be daunting. Ensuring that the agents work harmoniously towards a common goal requires careful design and well-defined protocols. Additionally, managing the increasing complexity as more agents are added to the system necessitates a robust framework that can handle the growing intricacy.
One key aspect of dealing with system complexity in multi-agent LLM systems is the need for effective resource management. As the number of agents grows and the interactions between them become more intricate, resource allocation becomes a critical issue. Balancing the computational resources, memory usage, and communication bandwidth among the agents is essential to prevent bottlenecks and ensure optimal system performance. This requires sophisticated algorithms and mechanisms to dynamically adjust resource allocation based on the changing demands and priorities within the system.
Overcoming Communication Barriers
Communication is a crucial aspect of multi-agent LLM systems. Efficient information exchange and coordination are essential for the agents to collaborate effectively. However, achieving seamless communication among agents can be challenging, especially when they operate in different domains or have diverse knowledge representations. Bridging these communication barriers requires standardized communication protocols and mechanisms that enable effective knowledge sharing and understanding.
In addition to standardized communication protocols, addressing communication barriers in multi-agent LLM systems also involves considering the impact of environmental factors on information exchange. External factors such as network latency, packet loss, and system failures can significantly affect the reliability and timeliness of communication between agents. Implementing robust error detection and correction mechanisms, as well as adaptive communication strategies that can mitigate the impact of environmental disturbances, is crucial for maintaining the overall system performance and resilience in the face of unpredictable conditions.
Future Trends in Multi-Agent LLM Systems
Predicted Developments in LLM Technology
The field of LLM systems continues to evolve rapidly, with advancements in technology shaping its future trajectory. Forecasts suggest that the integration of machine learning algorithms with optimization techniques will become more commonplace. This integration will enable LLM systems to not only learn from data but also optimize their decision-making processes based on the desired outcomes. By leveraging machine learning algorithms, organizations can enhance their ability to analyze complex data sets, identify patterns, and make informed decisions.
Furthermore, the utilization of deep learning techniques, which have proven highly effective in various domains, is anticipated to play a pivotal role in further enhancing the capabilities of LLM systems. Deep learning algorithms, inspired by the structure and function of the human brain, allow LLM systems to learn complex representations of data and extract meaningful insights. This ability to automatically learn hierarchical representations will enable organizations to tackle increasingly complex problems and unlock new opportunities.
The Impact of AI on Multi-Agent LLM Systems
Artificial Intelligence (AI) is expected to have a transformative impact on multi-agent LLM systems. As AI techniques mature and become more sophisticated, they will empower agents within these systems to acquire deeper insights, make more accurate predictions, and generate more optimal solutions. Reinforcement learning, in particular, holds immense potential for enhancing the decision-making capabilities of multi-agent LLM systems.
Reinforcement learning is a type of machine learning where an agent learns to make decisions by interacting with an environment and receiving feedback in the form of rewards or punishments. By applying reinforcement learning to multi-agent LLM systems, organizations can create intelligent agents that learn from their experiences and adapt their strategies to achieve desired outcomes. This adaptive decision-making capability will enable organizations to navigate complex and dynamic environments with ease, making them more agile and responsive to changing circumstances.
To sum up, multi-agent LLM systems offer a promising avenue for addressing complex challenges in a diverse range of industries. By harnessing the power of learning, logic, and optimization, these systems enable organizations to enhance their problem-solving capabilities, improve flexibility and scalability, and overcome the limitations of conventional approaches. However, implementing multi-agent LLM systems does present certain challenges, including system complexity and communication barriers. Nevertheless, by embracing predicted developments and the integration of AI, organizations can pave the way for a future of intelligent decision-making and transformative solutions.
Ready to harness the full potential of multi-agent LLM systems and transform your organization's approach to complex problem-solving? CastorDoc is here to lead the way. As the most reliable AI Agent for Analytics, CastorDoc empowers your teams with instant, trustworthy data answers, enabling self-service analytics and maximizing the ROI of your data stack. Experience the power of informed decision-making with the autonomy and trust that CastorDoc provides. Try CastorDoc today and take the first step towards a future of intelligent decision-making and transformative solutions.
You might also like
Get in Touch to Learn More
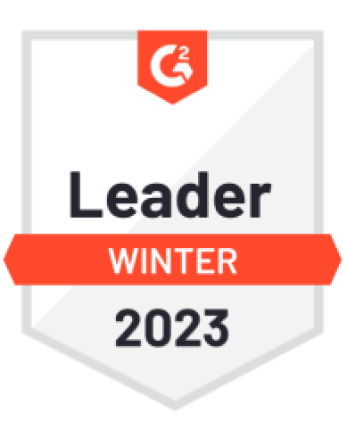
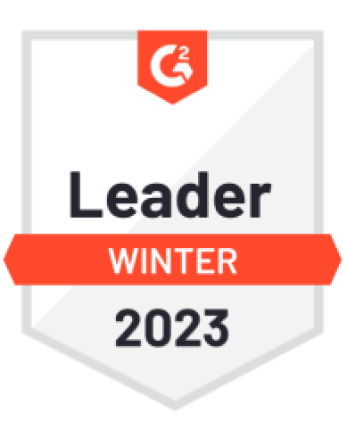

“[I like] The easy to use interface and the speed of finding the relevant assets that you're looking for in your database. I also really enjoy the score given to each table, [which] lets you prioritize the results of your queries by how often certain data is used.” - Michal P., Head of Data