Backfilling Data Guide: The Ultimate Manual for 2024
Looking to master the art of backfilling data in 2024? Our comprehensive guide has got you covered! From essential techniques to advanced strategies, this ultimate manual will equip you with the knowledge and skills to effectively backfill data for optimal results.
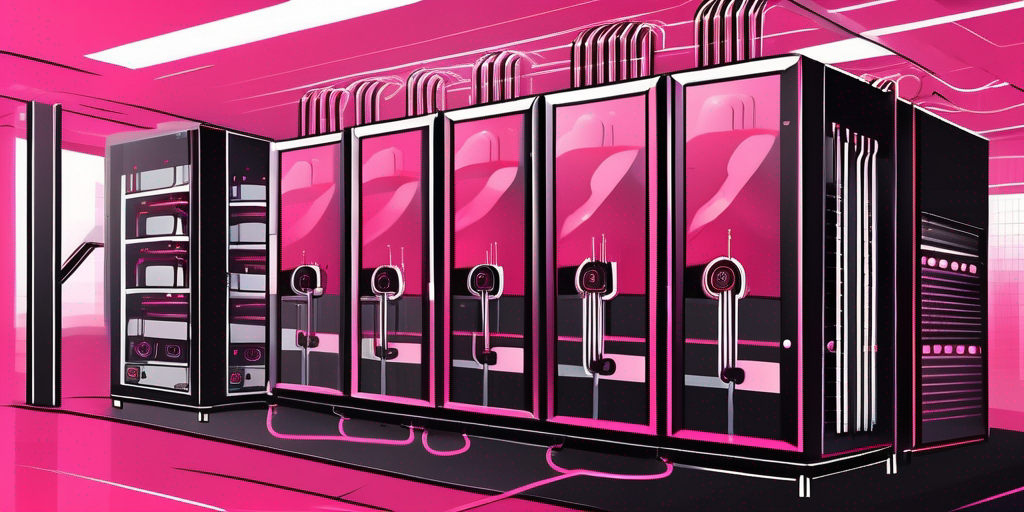
Backfilling data is an essential process in the world of data management. It involves filling in gaps in data sets with historical data, ensuring a complete and accurate record for analysis. This guide will delve into the intricacies of backfilling data, its importance, and how to effectively execute it.
Understanding Backfilling Data
Backfilling data is a process that involves filling in missing or incomplete data in a data set with historical data. This is often necessary when new metrics are introduced, or when data is lost or corrupted. The goal is to create a complete and accurate historical record for analysis and decision-making.
Backfilling data can be a complex process, depending on the size and nature of the data set. It requires a thorough understanding of the data, the sources of the data, and the tools and techniques used to manage and analyze the data.
Why Backfilling Data is Important
Backfilling data is crucial for several reasons. First, it ensures the completeness and accuracy of the data set, which is critical for reliable analysis and decision-making. Incomplete or inaccurate data can lead to erroneous conclusions and poor decisions.
Second, backfilling data allows for the analysis of trends over time. Without a complete historical record, it would be impossible to accurately identify and analyze long-term trends and patterns in the data.
How to Backfill Data
The process of backfilling data can vary depending on the specific circumstances, but there are some general steps that are typically followed. These steps include identifying the gaps in the data, sourcing the historical data, validating the data, and then integrating the data into the data set.
It's important to note that backfilling data should be done carefully and methodically to ensure the accuracy and integrity of the data set. Mistakes can lead to inaccurate data and misleading analysis.
Identifying Data Gaps
The first step in backfilling data is to identify the gaps in the data set. This involves analyzing the data set to identify any missing or incomplete data. This can be done using data analysis tools and techniques, such as data profiling and data quality assessment.
Once the gaps have been identified, it's important to understand why the data is missing or incomplete. This could be due to a variety of reasons, such as data corruption, data loss, or the introduction of new metrics.
Sourcing Historical Data
Once the data gaps have been identified and understood, the next step is to source the historical data to fill in the gaps. This can be a complex process, depending on the nature of the data and the sources of the data.
The historical data may come from a variety of sources, such as databases, data warehouses, data lakes, or external data sources. It's important to ensure that the historical data is accurate, reliable, and relevant to the data gap.
Validating the Data
Before integrating the historical data into the data set, it's crucial to validate the data. This involves checking the data for accuracy, consistency, and integrity. Data validation techniques, such as data cleansing and data verification, can be used to ensure the quality of the data.
Data validation is a critical step in the backfilling process. If the historical data is not validated, it could introduce errors and inaccuracies into the data set, compromising the reliability of the data and the analysis.
Integrating the Data
The final step in the backfilling process is to integrate the historical data into the data set. This involves merging the data into the existing data set, ensuring that the data is properly aligned and formatted.
Data integration can be a complex process, especially for large data sets or data sets with complex structures. Data integration tools and techniques, such as data mapping and data transformation, can be used to facilitate the integration process.
Challenges in Backfilling Data
While backfilling data is a crucial process in data management, it's not without its challenges. These challenges can range from sourcing accurate historical data to ensuring the integrity of the data set after the backfilling process.
One of the main challenges in backfilling data is sourcing accurate and reliable historical data. If the historical data is not accurate or reliable, it could compromise the integrity of the data set and lead to inaccurate analysis and decision-making.
Ensuring Data Integrity
Another challenge in backfilling data is ensuring the integrity of the data set after the backfilling process. This involves ensuring that the data is accurate, consistent, and complete after the historical data has been integrated.
Data integrity can be ensured through various data management practices, such as data validation, data cleansing, and data governance. These practices help to maintain the quality of the data and ensure the reliability of the data analysis and decision-making.
Conclusion
Backfilling data is a critical process in data management, ensuring the completeness and accuracy of data sets for reliable analysis and decision-making. While it can be a complex process, with the right understanding, tools, and techniques, it can be effectively executed.
As we move further into the digital age, the importance of backfilling data will only continue to grow. By understanding and effectively executing the backfilling process, organizations can ensure the integrity of their data, enabling them to make informed decisions based on accurate and complete data.
You might also like
Get in Touch to Learn More
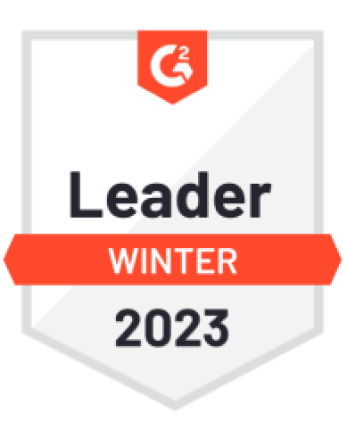
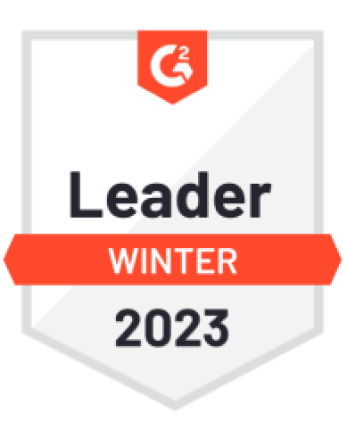

“[I like] The easy to use interface and the speed of finding the relevant assets that you're looking for in your database. I also really enjoy the score given to each table, [which] lets you prioritize the results of your queries by how often certain data is used.” - Michal P., Head of Data