Data Mart vs Data Lake: What's Right for Your Team in 2024?
Discover the key differences between data mart and data lake, and find out which one is the best fit for your team in 2024.
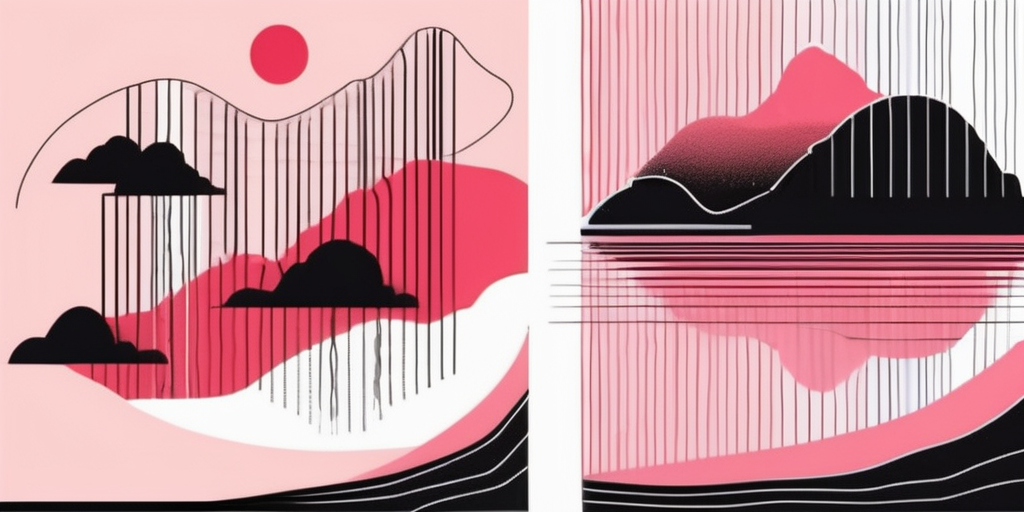
In the fast-paced world of data management, it is essential for organizations to have efficient systems in place to store and analyze their vast amounts of data. Two popular options that have emerged in recent years are data marts and data lakes. Although they both serve the purpose of enabling data-driven decision making, understanding their differences and determining which one suits your team's needs can be a challenging task. In this article, we will explore the basics of data marts and data lakes, delve into their key differences, and provide insights for evaluating your team's requirements. Additionally, we will discuss the pros and cons of both options to help you make an informed decision.
Understanding the Basics: Data Mart and Data Lake
Defining Data Mart
A data mart is a subset of a data warehouse that focuses on a specific area or department within an organization. It is designed to provide targeted information to support the decision-making process of a particular team or business unit. Data marts are structured repositories that store pre-aggregated and cleansed data, making it easier for business users to access and analyze relevant information.
Data marts are often tailored to the needs of a particular business function, such as sales, marketing, or finance. By focusing on a specific area, data marts can provide more specialized and detailed insights compared to a general-purpose data warehouse. This targeted approach allows organizations to efficiently analyze data related to a particular aspect of their operations, leading to more informed decision-making and strategic planning.
Defining Data Lake
On the other hand, a data lake is a vast pool of raw and unprocessed data from multiple sources within and outside an organization. Unlike a data mart, data lakes do not enforce any data structure or schema, allowing for more flexibility and scalability. Data lakes are typically built on distributed systems and support a wide variety of data types, including structured, semi-structured, and unstructured data.
Within a data lake, organizations can store massive amounts of data in its original form, without the need for upfront transformation or integration. This raw data can include everything from customer transactions and social media interactions to sensor data and log files. The flexibility of data lakes enables organizations to store diverse data sets without predefined schemas, making it easier to explore and analyze data in its native format.
Key Differences Between Data Mart and Data Lake
Data Structure and Storage
One of the significant differences between data marts and data lakes is their approach to data structure and storage. Data marts have a pre-defined structure and schema where data is organized in a way that aligns with the specific needs of a team or business unit. This structured approach allows for easier data management and ensures that the data is readily accessible for analysis.
On the other hand, data lakes embrace a "schema-on-read" methodology, meaning that the structure and schema are only applied when the data is accessed or queried. This flexibility allows for the storage of raw and unprocessed data, which can be beneficial for exploratory analysis and advanced analytics. However, it also means that data lakes require additional processing steps before the data can be used effectively.
Furthermore, data marts store cleansed and pre-aggregated data, which enables faster query response times and promotes better performance for analytical purposes. This optimized structure allows for quick and efficient analysis, making data marts ideal for scenarios where speed is of the essence. However, this also means that data marts may not be suitable if your team requires access to raw and unprocessed data for more in-depth exploration.
Accessibility and User Interface
When it comes to accessibility and user interface, data marts offer a more user-friendly experience compared to data lakes. Since data marts are designed for specific teams or business units, they are tailored to the needs of those users. This customization includes providing a user interface that is intuitive and easy to navigate, making it simpler for non-technical users to access and interact with the data.
Data lakes, on the other hand, provide a more flexible but less user-friendly environment. While data lakes offer unlimited storage capacity and the ability to retain vast amounts of data, accessing and querying this data requires technical expertise. Data lakes are commonly utilized by data engineers and data scientists who possess the skills to explore and analyze raw data using programming languages and tools.
However, it is worth noting that advancements in data lake technologies have led to the development of user-friendly interfaces and tools that bridge the gap between technical and non-technical users. These tools provide a more intuitive way to interact with data lakes, making them more accessible to a broader range of users.
Speed and Performance
The speed and performance of data marts and data lakes differ significantly due to their approach to data storage and processing. Data marts, with their pre-aggregated and structured data, are optimized for fast and efficient query response times. This makes data marts particularly suitable for scenarios where quick and interactive analysis is required.
Data lakes, on the other hand, may experience performance challenges when dealing with vast amounts of raw and unprocessed data. The need to apply a schema on read and potentially process a large volume of data can result in slower query performance. However, with proper implementation and optimization, data lakes can still provide high-performance analytics for data scientists and analysts.
Moreover, data lakes offer the advantage of scalability, allowing organizations to store and process massive amounts of data without worrying about storage limitations. This scalability ensures that data lakes can handle the ever-growing volume of data generated by modern businesses, enabling organizations to make data-driven decisions based on a comprehensive and holistic view of their data.
Evaluating Your Team's Needs
Determining Your Data Volume
One crucial factor to consider when deciding between a data mart and a data lake is the volume of data your team needs to store and analyze. Data marts are suitable for smaller to medium-sized datasets that require targeted analysis and quick access. They are structured repositories optimized for specific business needs, making them ideal for teams looking to gain insights into particular aspects of their operations efficiently. Data marts are often used by departments such as sales, marketing, or finance to analyze data related to their functions.
Conversely, if your team deals with large volumes of data or anticipates future growth, a data lake may be a better choice due to its scalability and ability to handle diverse data types. Data lakes offer a more flexible and cost-effective solution for storing vast amounts of raw data in its native format. This raw data can then be transformed and analyzed as needed, providing organizations with the agility to adapt to changing business requirements and explore new insights.
Assessing Your Team's Technical Skills
Another important consideration is the technical skills available within your team. Data marts are designed for business users and do not require extensive programming knowledge or data engineering expertise. They typically offer user-friendly interfaces and tools that empower non-technical users to access and analyze data independently. This accessibility can lead to faster decision-making and increased efficiency in day-to-day operations.
On the other hand, data lakes require a more technical skill set, including proficiency in programming languages like SQL, Python, or R, as well as experience in data engineering and data processing. Teams considering a data lake solution should assess their current capabilities and evaluate the need for additional training or hiring to effectively leverage the full potential of a data lake environment.
Identifying Your Business Goals
Finally, understanding your team's business goals is crucial for making the right decision. Data marts are well-suited for teams focused on specific business processes or departments that require quick and targeted analysis. By providing predefined data models and reports tailored to specific use cases, data marts empower decision-makers to extract actionable insights promptly.
On the other hand, data lakes are ideal for organizations seeking to enable data exploration, advanced analytics, and machine learning capabilities across diverse datasets. They support a more holistic approach to data analysis, allowing teams to uncover hidden patterns, perform complex analytics, and drive innovation through data-driven decision-making.
Pros and Cons of Data Mart
Benefits of Using Data Mart
- Provides a structured and intuitive environment for business users
- Enables faster query response times and performance
- Supports targeted analysis and decision-making processes
- Easier to implement and maintain compared to data lakes
Drawbacks of Using Data Mart
- May limit access to raw and unprocessed data, hindering exploratory analysis
- May require additional data integration efforts to combine data from various sources
- Limited scalability compared to data lakes
Pros and Cons of Data Lake
Advantages of Using Data Lake
- Offers storage for vast amounts of raw and unprocessed data
- Provides flexibility and scalability to handle diverse data types
- Enables exploration and analysis of data with various schemas
- Supports advanced analytics and machine learning capabilities
Disadvantages of Using Data Lake
- Requires technical expertise to access and analyze data
- Potential performance challenges when dealing with large volumes of raw data
- Lack of user-friendly interfaces for non-technical users
In conclusion, when deciding between a data mart and a data lake for your team's needs in 2024, it is crucial to consider factors such as data volume, technical skills, and business goals. Data marts provide structured and tailored environments for targeted analysis, while data lakes offer flexibility and scalability for handling diverse datasets. Assessing your team's needs and evaluating the pros and cons of each option will enable you to make an informed decision and empower your team with the right data management solution.
You might also like
Get in Touch to Learn More
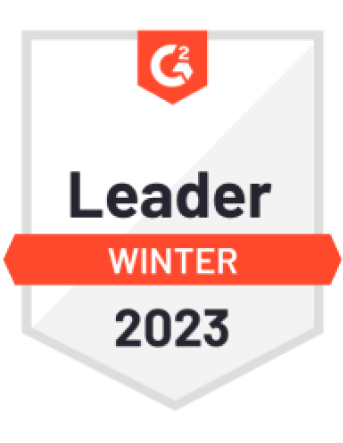
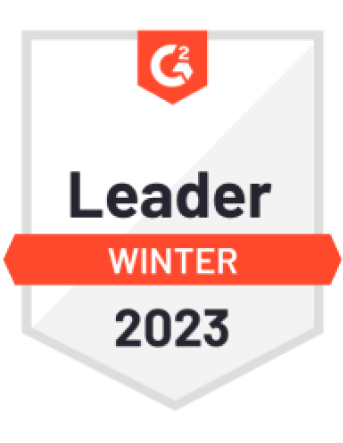
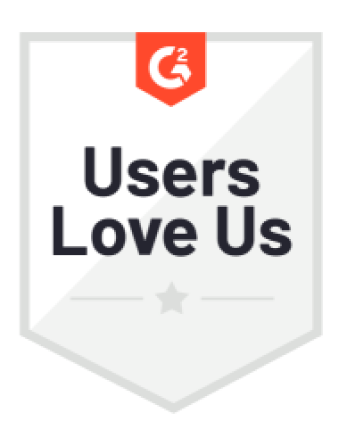
“[I like] The easy to use interface and the speed of finding the relevant assets that you're looking for in your database. I also really enjoy the score given to each table, [which] lets you prioritize the results of your queries by how often certain data is used.” - Michal P., Head of Data