Data Quality Dimensions: Do They Matter in 2024?
Explore the relevance of data quality dimensions in 2024
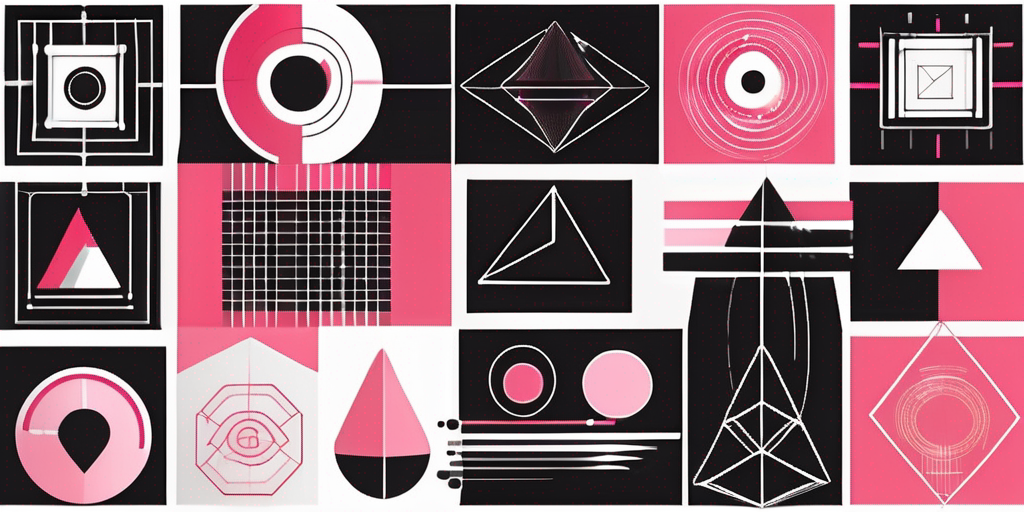
In today's data-driven world, the importance of data quality cannot be overstated. Organizations rely on accurate and reliable data to make informed decisions, drive business growth, and gain a competitive edge. However, as technology continues to advance at an unprecedented pace, the data landscape is constantly evolving, raising the question: do data quality dimensions still matter in 2024?
Understanding Data Quality Dimensions
Data quality dimensions refer to the various aspects or characteristics of data that determine its overall quality. These dimensions are used as a framework to assess the reliability, accuracy, completeness, consistency, and relevance of data. By evaluating data through these dimensions, organizations can ensure that their data meets the required standards and is fit for use.
Definition of Data Quality Dimensions
Data quality dimensions can be categorized into several key areas:
- Accuracy: The degree to which data reflects the true values or states of the objects or events it represents.
- Completeness: The extent to which all required data elements and attributes are present.
- Consistency: The absence of contradictory or conflicting values within and across datasets.
- Timeliness: The degree to which data is available and accessible when needed.
- Relevance: The alignment of data with the intended purpose or use case.
- Validity: The extent to which data adheres to predefined rules or constraints.
- Uniqueness: The presence of distinct and non-duplicate data records.
The Importance of Data Quality Dimensions
Data quality dimensions play a crucial role in ensuring the trustworthiness and reliability of data. Poor data quality can adversely affect organizations in several ways:
- Inaccurate insights: Decision-making based on flawed or incomplete data can lead to poor business outcomes and missed opportunities.
- Operational inefficiencies: Data errors and inconsistencies can disrupt business processes, resulting in wasted time and resources.
- Compliance risks: In industries with strict regulatory requirements, non-compliance due to poor data quality can result in legal and financial repercussions.
- Damaged reputation: Providing inaccurate or misleading information to customers erodes trust and damages the organization's reputation.
Furthermore, data quality dimensions are not static; they can evolve and change over time. As technology advances and new data sources emerge, organizations must adapt their data quality frameworks to address these changes. For example, in the era of big data, where organizations collect and analyze vast amounts of data from various sources, the dimension of scalability becomes increasingly important. Scalability refers to the ability of data quality processes and systems to handle large volumes of data without sacrificing accuracy or timeliness.
Another dimension that has gained prominence in recent years is the dimension of ethicality. With the growing concern over data privacy and security, organizations must ensure that their data collection and usage practices align with ethical standards. This includes obtaining informed consent from individuals, anonymizing data to protect privacy, and implementing robust security measures to prevent unauthorized access or breaches.
By considering these additional dimensions, organizations can enhance their data quality frameworks and address the evolving challenges and opportunities in the data-driven landscape. It is essential to continuously evaluate and improve data quality to maintain a competitive edge and make informed decisions based on reliable and trustworthy data.
The Evolution of Data Quality Dimensions
Over the years, data quality dimensions have evolved to keep pace with technological advancements and changing business needs. As organizations continue to rely heavily on data-driven insights to make strategic decisions, the importance of maintaining high-quality data has become increasingly evident.
One significant aspect of the evolution of data quality dimensions is the shift towards a more holistic approach. While accuracy and completeness remain fundamental, modern data quality frameworks now emphasize a broader set of dimensions to ensure that data is not only correct but also reliable, relevant, and usable across various applications and systems.
Data Quality Dimensions in the Past
Traditionally, data quality dimensions focused primarily on accuracy and completeness. The ability to ensure data accuracy and completeness was considered crucial in driving informed decision-making and enabling organizations to meet regulatory requirements. However, as data volumes increased and new technologies emerged, the scope of data quality dimensions expanded.
Moreover, in the past, data quality efforts were often siloed within specific departments or business units, leading to inconsistencies and redundancies in data management practices. This fragmented approach limited the organization's ability to leverage data as a strategic asset fully.
Current Trends in Data Quality Dimensions
In recent years, there has been a growing recognition of the importance of additional dimensions, such as consistency, timeliness, relevance, validity, and uniqueness. Organizations are now striving to ensure that their data is not only accurate and complete but also consistently defined, up-to-date, relevant to their business objectives, valid according to established rules, and free from duplicates.
Furthermore, the emergence of big data and advanced analytics has underscored the need for data quality dimensions that address the scalability and agility requirements of modern data environments. Organizations are investing in technologies and processes that can adapt to the dynamic nature of data and ensure that quality standards are maintained throughout the data lifecycle.
Data Quality Dimensions in 2024
Looking ahead to 2024, it is clear that data quality dimensions will continue to play a critical role in organizations' data strategies. However, several changes and challenges are expected to shape the future of data quality.
Predicted Changes in Data Quality Dimensions
As technologies like artificial intelligence (AI), machine learning (ML), and big data analytics become more prevalent, new data quality dimensions may emerge. For example, explainability and interpretability dimensions may gain importance as organizations seek to understand the algorithms and models driving their AI-based decision-making processes.
Moreover, with the rise of Internet of Things (IoT) devices and edge computing, data quality dimensions related to real-time data processing and edge analytics are likely to become more prominent. Ensuring data accuracy, completeness, and timeliness in these fast-paced environments will be crucial for organizations striving to gain valuable insights from their data.
The Impact of Technology on Data Quality Dimensions
While technology advancements present opportunities for improved data quality, they also pose challenges. The increasing complexity of data sources, formats, and structures may make it more difficult to ensure data accuracy and consistency. Organizations must invest in robust data governance frameworks, advanced data integration tools, and automated data quality monitoring to overcome these challenges.
Furthermore, the growing emphasis on data privacy and security in the wake of stringent regulations like the General Data Protection Regulation (GDPR) and the California Consumer Privacy Act (CCPA) adds another layer of complexity to data quality dimensions. Organizations need to incorporate data anonymization, encryption, and access controls into their data quality strategies to protect sensitive information and comply with data protection laws.
The Relevance of Data Quality Dimensions in 2024
Some may argue that advancements in technology, such as AI and ML, render traditional data quality dimensions obsolete. However, this viewpoint overlooks the fundamental purpose and value of data quality dimensions in ensuring the reliability and usefulness of data.
In today's data-driven world, the importance of data quality dimensions cannot be overstated. As we look towards 2024 and beyond, the significance of these dimensions will only continue to grow. With the exponential increase in data volume and sources, maintaining high-quality data is crucial for organizations to gain a competitive edge, drive innovation, and foster customer trust.
Why Data Quality Dimensions Will Still Matter
While the specific dimensions may evolve, the underlying principles of data quality will remain steadfast. Regardless of the technology used, organizations will always need accurate, complete, consistent, timely, relevant, valid, and unique data to make informed decisions and achieve their business objectives.
Moreover, as data becomes more interconnected and interdependent across systems and platforms, the need for robust data quality dimensions becomes even more pressing. Inaccurate or inconsistent data can have far-reaching consequences, impacting not only decision-making processes but also regulatory compliance and customer satisfaction.
Potential Challenges and Solutions
As organizations strive to maintain data quality in an increasingly complex data landscape, they will face challenges such as data privacy concerns, data governance issues, and data integration complexities. To address these challenges, organizations must implement comprehensive data governance frameworks, establish clear policies and procedures, invest in data quality tools and technologies, and cultivate a data-driven culture across the organization.
Furthermore, the rise of edge computing and IoT devices introduces new challenges to data quality, as data is generated and processed at the edge of the network. Organizations will need to adapt their data quality strategies to encompass edge data sources, ensuring that data is accurate, secure, and compliant with regulations.
The Future of Data Quality Management
In addition to the evolving data quality dimensions, the future of data quality management will see innovations aimed at optimizing data quality processes and ensuring continuous improvement.
Innovations in Data Quality Management
Advanced technologies, such as automated data profiling, data cleansing, and data integration, will simplify and streamline data quality management tasks. AI and ML algorithms will be leveraged to identify, prioritize, and resolve data quality issues in real-time, reducing the manual effort required and improving overall efficiency.
Preparing for the Future of Data Quality Management
To prepare for the future of data quality management, organizations must prioritize data quality initiatives, invest in robust data quality management platforms, and foster a data-centric culture. This includes providing adequate training and resources to data stewards, promoting data literacy among employees, and setting clear data quality goals and metrics.
In conclusion, data quality dimensions will continue to be of paramount importance in 2024 and beyond. Despite the rapid technological advancements, the need for accurate, reliable, and relevant data remains a critical factor for organizations to drive successful decision-making, operational efficiency, and regulatory compliance. By embracing evolving data quality dimensions, organizations can harness the power of data and excel in the data-driven future.
You might also like
Get in Touch to Learn More
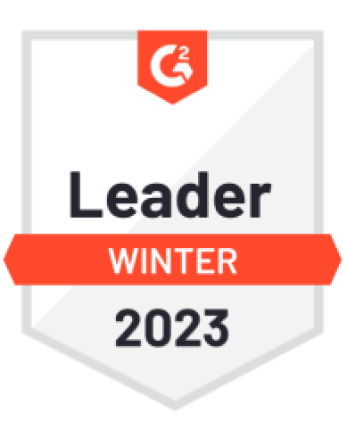
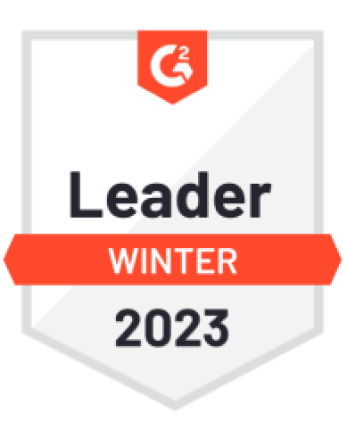

“[I like] The easy to use interface and the speed of finding the relevant assets that you're looking for in your database. I also really enjoy the score given to each table, [which] lets you prioritize the results of your queries by how often certain data is used.” - Michal P., Head of Data