Data Quality Fundamentals: Why It Matters in 2024!
Discover the crucial role of data quality in 2024 and why it's a game-changer for businesses.

Data has become the lifeblood of organizations across industries. It drives decision-making, improves operational efficiency, and enables companies to gain a competitive edge. However, the quality of data cannot be taken for granted. In 2024 and beyond, data quality will play a pivotal role in ensuring the success and sustainability of businesses. This article explores the concept of data quality, its importance, the future trends, strategies for ensuring data quality, and overcoming challenges associated with it.
Understanding the Concept of Data Quality
Data quality refers to the accuracy, completeness, consistency, and reliability of data. In simpler terms, it determines the extent to which data can be trusted to make informed business decisions. Achieving high data quality requires a comprehensive understanding of its key components.
Defining Data Quality
Defining data quality involves establishing specific criteria and standards that data must meet. These criteria typically include factors such as accuracy, validity, timeliness, relevance, and uniqueness. Data quality standards serve as benchmarks for evaluating and improving the overall quality of data.
Key Components of Data Quality
Data quality can be assessed based on several key components. These components include:
- Data Accuracy: Data accuracy measures how closely the data reflects the reality it represents. Accuracy can be determined by comparing data against reliable sources or conducting data validation exercises.
- Data Completeness: Completeness refers to the presence of all required data fields and attributes. Incomplete data can lead to gaps in decision-making and inaccurate analysis.
- Data Consistency: Consistency ensures that data remains stable and coherent across different systems, databases, or time periods. Inconsistencies can result in duplication, conflicts, or discrepancies.
- Data Timeliness: Timeliness emphasizes the need for data to be available and up-to-date when required. Outdated or delayed data can hinder decision-making processes and lead to missed opportunities.
- Data Relevance: Relevance determines the degree to which data is applicable and useful for a specific purpose or analysis. Irrelevant data can lead to erroneous conclusions or misguided actions.
- Data Uniqueness: Uniqueness ensures that each data record is distinct and free from duplication. Duplicate data can skew analysis, impact data integrity, and hinder accurate decision-making.
Understanding the concept of data quality is crucial for organizations in today's data-driven world. It goes beyond just having data; it is about having reliable and trustworthy data that can drive meaningful insights and actions. Data accuracy, one of the key components of data quality, is particularly important. Imagine a scenario where a company relies on inaccurate data to make critical business decisions. The consequences could be disastrous, leading to financial losses, damaged reputation, and missed opportunities.
Data completeness is another vital aspect of data quality. Incomplete data can create blind spots in decision-making processes, as important information might be missing. For example, if a marketing team is analyzing customer data to develop targeted campaigns, incomplete data could result in inaccurate segmentation and ineffective marketing strategies.
Data consistency is essential to ensure that data remains reliable and coherent across different systems and time periods. Inconsistencies in data can arise due to various reasons, such as data entry errors or integration issues between different databases. These inconsistencies can lead to confusion and conflicts, making it challenging to draw accurate conclusions from the data.
Timeliness is another critical component of data quality. In today's fast-paced business environment, having access to real-time or near real-time data is crucial for making informed decisions. Outdated or delayed data can hinder decision-making processes, as it may not reflect the current state of affairs. This can result in missed opportunities or delayed actions, impacting the organization's competitiveness.
Data relevance is about ensuring that the data being used is applicable and useful for a specific purpose or analysis. Irrelevant data can lead to misleading insights and misguided actions. It is important to carefully select and filter the data to ensure its relevance and avoid drawing incorrect conclusions.
Lastly, data uniqueness plays a significant role in maintaining data quality. Duplicate data can introduce errors and inconsistencies in analysis, impacting the integrity of the data. It is crucial to identify and eliminate duplicate records to ensure accurate decision-making and reliable insights.
The Importance of Data Quality in 2024
In this era of data-driven decision-making, organizations cannot afford to overlook the importance of data quality. It plays a significant role in various aspects of business operations.
With the exponential growth of data in the digital age, the quality of data has become paramount. Data quality encompasses several factors, including accuracy, completeness, consistency, and timeliness. Organizations must implement robust data quality management practices to ensure that their data is reliable and trustworthy.
Role of Data Quality in Decision Making
Data quality directly influences the accuracy and reliability of decision-making processes. Decision-makers heavily rely on data to gain insights, identify trends, and assess business performance. By ensuring high data quality, organizations can make well-informed decisions and mitigate the risks associated with poor-quality data.
Furthermore, in today's competitive landscape, the speed at which decisions are made is crucial. High data quality ensures that decision-makers have access to real-time, accurate information, enabling them to respond swiftly to market changes and stay ahead of the competition.
Impact of Data Quality on Business Performance
High-quality data is instrumental in driving business performance. It enables organizations to identify and capitalize on market opportunities, optimize processes, enhance customer experiences, and make data-driven strategic decisions. In contrast, poor-quality data can lead to inaccurate analysis, flawed strategies, and costly mistakes.
Moreover, data quality is not just a concern for internal decision-making processes. It also impacts external stakeholders, such as customers and partners. Ensuring data quality throughout the entire data lifecycle builds trust and credibility with stakeholders, fostering strong relationships and enhancing the organization's reputation in the market.
The Future of Data Quality
As technology continues to advance at a rapid pace, the future of data quality is bound to evolve. Organizations need to adapt to emerging trends to stay ahead in this data-centric era.
Predicted Trends in Data Quality
The future of data quality is expected to witness several trends:
- Automation: With the help of advanced analytics and artificial intelligence, organizations can automate data quality processes, including data profiling, validation, and cleansing.
- Real-Time Data Quality Monitoring: The ability to monitor data quality in real-time ensures proactive identification and resolution of issues, minimizing the impact on business operations.
- Integration of Data Quality into Agile Development: Incorporating data quality measures into agile development methodologies allows for continuous improvement and flexibility in handling evolving data requirements.
How Technology is Shaping Data Quality
Technology plays a crucial role in driving improvements in data quality. Advanced tools and techniques are being developed to enhance data quality management:
- Data Quality Tools: Software solutions enable organizations to assess, monitor, and improve data quality by automating data cleansing, validation, and profiling processes.
- Master Data Management: Master data management systems provide a single source of truth, ensuring data consistency, integrity, and accuracy across the organization.
- Data Governance: Data governance frameworks facilitate the establishment of data quality policies, processes, and controls to ensure data integrity and compliance.
Strategies for Ensuring Data Quality
Organizations need to adopt effective strategies to maintain and enhance data quality:
Best Practices for Data Quality Management
Implementing the following best practices can significantly improve data quality:
- Data Quality Assessment: Regularly assess data quality against predefined standards, identify gaps, and take corrective actions.
- Data Validation and Cleansing: Validate data for accuracy, completeness, and consistency, and cleanse it by removing duplicate, outdated, or irrelevant records.
- Data Integration: Establish robust data integration processes to ensure seamless data flow across systems and eliminate data silos.
- Data Quality Training: Train employees on data quality concepts, tools, and techniques to cultivate a data-driven culture.
Tools and Techniques for Data Quality Assurance
Several tools and techniques can be employed to ensure data quality:
- Data Profiling: Analyze the content, structure, and quality of data to gain insights into its characteristics and identify anomalies.
- Data Cleansing: Apply automated cleansing techniques, including standardization, deduplication, and normalization, to improve data accuracy and integrity.
- Data Monitoring: Continuously monitor data quality to identify patterns, trends, and outliers, allowing prompt corrective actions.
Overcoming Challenges in Data Quality
Even with the best strategies and tools in place, organizations may encounter challenges in maintaining and improving data quality.
Common Data Quality Issues and Solutions
Some common data quality issues are:
- Inaccurate and Inconsistent Data Entry: Implement data validation rules, conduct regular training, and enforce data quality standards to minimize errors during data entry.
- Data Integration Challenges: Establish well-defined data integration processes, use data integration tools, and ensure proper data mapping and transformation to overcome integration challenges.
- Data Decay: Adopt technologies and processes to capture and update data in real-time, minimizing the risk of stale or outdated information.
Mitigating Risks Associated with Poor Data Quality
To mitigate the risks associated with poor data quality, organizations should:
- Establish Data Governance Frameworks: Develop robust data governance frameworks that define roles, responsibilities, and processes for ensuring data quality and compliance.
- Conduct Regular Data Audits: Regularly audit data to identify gaps, assess data quality, and take proactive measures to address issues.
- Implement Data Quality Controls: Establish data quality controls at various stages of the data lifecycle to ensure consistent adherence to data quality standards.
In conclusion, data quality is fundamental to the success of organizations in 2024 and beyond. It forms the basis for accurate decision-making, drives business performance, and helps organizations stay competitive. As technology continues to evolve, organizations must embrace emerging trends, adopt effective strategies, and overcome challenges to ensure high-quality data. By doing so, organizations can navigate the data-driven landscape with confidence, empowering themselves with reliable insights and actionable intelligence.
You might also like
Get in Touch to Learn More
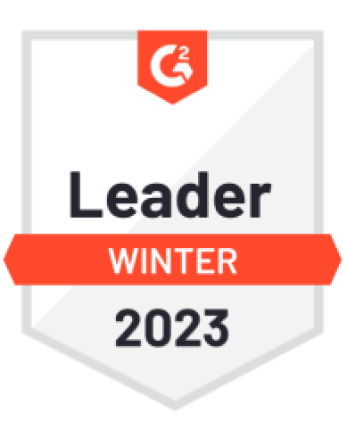
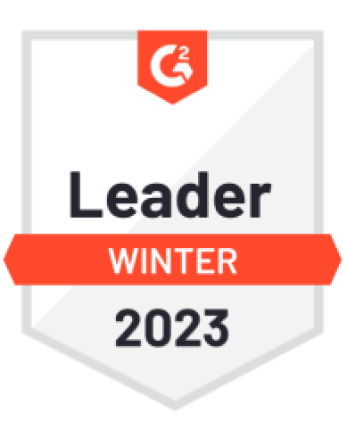

“[I like] The easy to use interface and the speed of finding the relevant assets that you're looking for in your database. I also really enjoy the score given to each table, [which] lets you prioritize the results of your queries by how often certain data is used.” - Michal P., Head of Data