How to Achieve an Optimal Hybrid Data Governance Model?
Discover the key strategies and best practices for creating a successful hybrid data governance model in this comprehensive article.

Hybrid data governance has emerged as a critical framework for organizations seeking to effectively manage their data assets. By combining the strengths of both centralized and decentralized governance models, companies can achieve an optimal approach that balances control and flexibility. In this article, we will delve into the various aspects of hybrid data governance and explore how organizations can implement this model successfully.
Understanding Hybrid Data Governance
Before we delve into the details, let's first define what hybrid data governance entails. It refers to an approach that combines elements of both centralized and decentralized data governance models. In this model, certain aspects of data management are centrally controlled, while others are delegated to individual business units or departments.
By adopting a hybrid approach, organizations can strike a balance between data standardization and agility. It enables them to leverage the advantages of centralized governance, such as data quality management and security, while also allowing business units to have some autonomy in managing their data.
Defining Hybrid Data Governance
Hybrid data governance is about establishing a structured framework that defines the roles, responsibilities, and processes involved in managing data across the organization. It encompasses policies, procedures, and tools that enable effective data governance while adapting to the evolving needs of the business.
The hybrid model typically involves creating a centralized data governance team that sets the overarching policies and standards. At the same time, it empowers individual business units to manage and govern their data within the established guidelines.
Within this framework, data governance teams work closely with business units to understand their specific data needs and requirements. They collaborate to develop tailored data management strategies that align with the organization's overall goals and objectives.
The Importance of Hybrid Data Governance
Now that we understand what hybrid data governance involves, let's explore why it is important for organizations in today's data-driven landscape. One of the main advantages of a hybrid approach is its ability to accommodate diverse data requirements and use cases.
With the increasing volume and variety of data that organizations deal with, it is crucial to have a governance model that can handle both structured and unstructured data. Hybrid data governance facilitates this by allowing flexibility in data management practices while ensuring adherence to established data governance principles.
Furthermore, hybrid data governance promotes collaboration and accountability within the organization. By involving different stakeholders and departments in the governance process, it fosters a sense of ownership and responsibility for data quality and compliance.
Moreover, hybrid data governance enables organizations to adapt to changing regulatory landscapes. With data privacy and security regulations becoming more stringent, organizations need a governance model that can keep up with these evolving requirements. The hybrid approach allows for the implementation of centralized controls and standards, ensuring compliance, while also allowing business units to address specific regulatory needs.
In addition, hybrid data governance supports innovation and agility. By giving business units some autonomy in managing their data, organizations can foster a culture of experimentation and exploration. This empowers teams to discover new insights and opportunities that can drive business growth and competitive advantage.
In conclusion, hybrid data governance offers organizations a flexible and adaptable approach to managing their data. By combining the strengths of centralized and decentralized governance models, it enables organizations to achieve data standardization, security, and compliance, while also promoting innovation and agility. It is a strategic framework that empowers organizations to harness the full potential of their data assets in today's data-driven landscape.
Key Components of a Hybrid Data Governance Model
Now that we have established the importance of hybrid data governance, let's dive into its key components. These components are essential for ensuring the success of the hybrid approach:
Data Quality Management
Data quality is at the core of effective data governance. In a hybrid model, it is crucial to establish robust data quality management practices that ensure consistency, accuracy, and integrity of data across the organization. This involves defining data quality metrics, implementing data cleansing and validation processes, and establishing data stewardship roles and responsibilities.
For example, organizations can implement automated data quality checks to identify and rectify any inconsistencies or errors in the data. This could include validating data against predefined rules, conducting regular data audits, and implementing data profiling techniques to gain insights into data quality issues. By prioritizing data quality management, organizations can ensure that the data used for decision-making is reliable and trustworthy.
Data Privacy and Security
In today's data-centric world, data privacy and security are of paramount importance. A hybrid data governance model needs to incorporate strong data privacy and security measures to protect sensitive information. This includes defining access controls, implementing encryption protocols, and ensuring compliance with relevant data protection regulations.
Organizations can adopt a multi-layered approach to data privacy and security in a hybrid model. This could involve implementing role-based access controls, where users are granted access to data based on their roles and responsibilities within the organization. Additionally, organizations can leverage encryption techniques such as data masking and tokenization to protect sensitive data at rest and in transit. Regular security audits and vulnerability assessments can also help identify and address any potential security gaps.
Data Integration and Interoperability
Effective data integration and interoperability are vital for seamless data management and analytics. In a hybrid model, organizations need to establish processes and technologies that enable data integration across different systems and platforms. This involves defining data integration standards, implementing data integration tools, and ensuring data interoperability for efficient data sharing and analysis.
One way organizations can achieve data integration and interoperability in a hybrid model is through the use of application programming interfaces (APIs). APIs allow different systems and applications to communicate and exchange data in a standardized manner. By implementing APIs, organizations can streamline data integration processes and enable real-time data sharing between on-premises and cloud-based systems.
Furthermore, organizations can leverage data virtualization technologies to create a unified view of data from disparate sources. Data virtualization enables organizations to access and analyze data from various systems without the need for physical data movement or replication. This not only simplifies data integration but also improves data agility and reduces the time and effort required for data analysis.
Steps to Implementing a Hybrid Data Governance Model
Implementing a hybrid data governance model requires careful planning and execution. Let's explore the key steps involved:
Assessing Your Current Data Governance
The first step is to assess your organization's existing data governance practices. This involves evaluating the strengths and weaknesses of your current approach and identifying areas that can benefit from the hybrid model. It is important to involve key stakeholders from different departments to ensure a comprehensive assessment.
During the assessment phase, it is crucial to conduct thorough data profiling and analysis to understand the quality, integrity, and usage of your organization's data. This step will help in identifying data gaps, redundancies, and inconsistencies that need to be addressed through the hybrid data governance model.
Planning Your Hybrid Data Governance Strategy
Once you have assessed your current data governance, the next step is to develop a comprehensive strategy for implementing the hybrid model. This involves defining the roles and responsibilities of the centralized data governance team and individual business units, establishing data governance policies and standards, and selecting the appropriate tools and technologies to support the hybrid approach.
Furthermore, as part of the planning phase, it is essential to create a data governance roadmap that outlines the timeline for implementing the hybrid model, key milestones, and success criteria. This roadmap will serve as a guiding document to ensure a structured and organized approach to transitioning to the new governance model.
Executing Your Hybrid Data Governance Plan
With a well-defined strategy in place, it's time to execute your hybrid data governance plan. This involves implementing the necessary processes and technologies, training your staff on the new governance model, and establishing communication channels to ensure effective collaboration between the centralized governance team and business units.
Additionally, during the execution phase, it is important to monitor and measure the effectiveness of the hybrid data governance model through key performance indicators (KPIs) and regular audits. This continuous evaluation will help in identifying any gaps or areas for improvement, allowing for timely adjustments to ensure the success of the hybrid data governance implementation.
Overcoming Challenges in Hybrid Data Governance
While hybrid data governance offers numerous benefits, it is not without its challenges. Let's explore some key challenges and how to overcome them:
Dealing with Data Silos
One challenge in a hybrid model is the risk of creating data silos within different business units. To overcome this, organizations need to establish strong data integration practices and promote cross-functional collaboration. This includes implementing data integration tools, establishing data sharing protocols, and fostering a culture of data transparency and collaboration.
Ensuring Compliance and Security
Compliance and security are critical in any data governance model. In a hybrid approach, it is important to establish robust procedures and safeguards to ensure compliance with relevant data protection regulations and mitigate security risks. This includes regular audits, implementing data encryption, and conducting employee training on data privacy and security best practices.
Managing Change in the Organization
Implementing a hybrid data governance model often requires significant organizational change. To successfully manage this change, organizations need to communicate effectively, involve key stakeholders from the beginning, and provide sufficient training and support to staff. It is also essential to monitor and measure the impact of the hybrid model and make necessary adjustments along the way.
In conclusion, implementing a hybrid data governance model is a complex undertaking but offers significant benefits for organizations seeking to optimize their data management practices. By striking the right balance between centralized control and decentralized flexibility, organizations can achieve an optimal approach that promotes data quality, privacy, and security while enabling agility and innovation.
You might also like
Get in Touch to Learn More
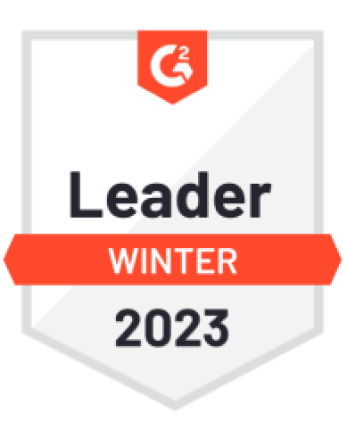
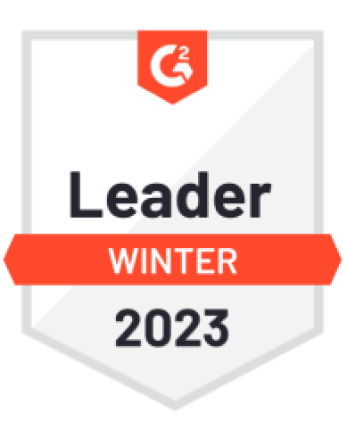

“[I like] The easy to use interface and the speed of finding the relevant assets that you're looking for in your database. I also really enjoy the score given to each table, [which] lets you prioritize the results of your queries by how often certain data is used.” - Michal P., Head of Data