dbt Data Lineage: How It Works and How to Leverage It
Discover the ins and outs of dbt data lineage in this article.
%202.png)
Data lineage is like a family tree for your data. It shows where your data comes from, how it changes, and where it ends up. This helps companies make sure their data is accurate and trustworthy. Let's dive into what data lineage is all about, how a tool called dbt helps with it, and how you can use dbt to get the most out of your data.
Understanding the Concept of Data Lineage
Think of data lineage as a map that shows the journey of your data. It's not just about knowing where your data is now, but also understanding where it's been and how it's changed along the way. This information is super helpful for making sure your data is reliable and secure.
By capturing metadata and lineage information, organizations can gain valuable insights into the quality, dependencies, and relationships of their data assets.
Data lineage is also a critical component in data governance frameworks. Data lineage not only aids in understanding the flow of data but also assists in ensuring data security. .When a company has a clear picture of its data's journey, it can make better decisions using trustworthy information. This way, data lineage helps improve overall data management.
Defining Data Lineage
Data lineage works in two directions. Going forward, it shows how your data changes at each step. Going backward, it helps you find out where your data originally came from. This is really useful for checking if your data is accurate and trustworthy.
These functions help businesses improve their data discovery processes and enhances collaboration among teams.
The Importance of Data Lineage in Data Management
Good data lineage is crucial for keeping your data high-quality and following the rules. It helps companies spot and fix problems quickly, like when data doesn't match up or has errors. It's also really important for meeting legal requirements and compliance, as it shows that your data is valid and reliable.
In today's world of big data, data lineage is like a compass. It helps data experts work together more easily, understand how different pieces of data are connected, and keep everything accurate across all the different systems they use.
An Introduction to dbt (Data Build Tool)
dbt, which stands for Data Build Tool, is a free tool that helps data teams transform, test, and use their data for analysis. It's like a Swiss Army knife for data professionals, making it easier to build and maintain reliable data processes.
dbt is a game-changer in the world of data analytics. It provides a solid platform for data pros to streamline their work and collaborate better. By using SQL (a language for working with databases), dbt caters specifically to what data analysts and engineers need.
What is dbt?
At its heart, dbt is a tool that uses SQL to help data analysts and engineers work with their data. It's designed to make it easy for different types of data professionals to work together on complex data projects.
With dbt, data teams can break free from old-school data processing methods. It lets them write code that's easy to understand and works really well, making sure data is processed quickly and accurately.
Key Features and Benefits of dbt
dbt has several key features that make it great for working with data:
- Modularity: It lets you reuse code, so different teams can share and use common data transformations.
- Version Control: It works with systems like Git, so teams can manage changes to their code and work together effectively.
- Testing Framework: It has built-in testing, so you can automatically check if your data models are working correctly.
- Documentation Generation: It automatically creates documentation, making it easier to understand and maintain your data models.
Plus, dbt's modularity means data teams can create a library of standard SQL transformations. This saves time and effort when developing new data pipelines, as you don't have to start from scratch each time.
How dbt Supports Data Lineage
dbt's unique approach to transforming data makes it great for supporting data lineage. It keeps track of metadata and lineage, giving you detailed insights into how your data is transformed and processed.
The Role of dbt in Data Lineage
dbt stores information about data models, transformations, and dependencies in its internal catalog. This catalog lets organizations trace the lineage of each piece of data, understand how it's transformed, and identify potential issues. By using this lineage information, data teams can ensure data accuracy, improve data governance, and make it easier for people to analyze data together.
dbt's Approach to Data Lineage
dbt's approach to data lineage is based on creating a map of how different data models depend on each other. Each transformation in dbt is treated as a separate model, and the relationships between these models are clearly defined. This map-based approach allows dbt to capture the complete lineage of data and show how it flows through the pipeline.
dbt doesn't just show you the current state of your data lineage. It also keeps track of historical information, so you can see how data transformations have changed over time. This is super helpful for auditing and for understanding how changes impact downstream analyses.
Enhancing Data Governance with dbt
One of the big benefits of dbt's data lineage capabilities is that it helps improve data governance practices. By clearly showing how data is transformed and where it comes from, data governance teams can set up policies and procedures to ensure data quality and compliance. This proactive approach helps organizations maintain trust in their data and meet regulatory requirements.

Leveraging dbt for Effective Data Lineage
To get the most out of dbt for data lineage, you need to follow some steps and best practices. By doing this, organizations can optimize the benefits of dbt and ensure their data is reliable and traceable.
Steps to Implement dbt for Data Lineage
- Define Data Models: Start by identifying the key data models and transformations you want to track. Create separate dbt models for each transformation.
- Establish Dependencies: Define how the data models are related using dbt's map-based approach. This ensures accurate tracing of the data lineage.
- Capture Metadata: Use dbt's built-in tools to capture metadata and lineage information. This includes using dbt's catalog and integrating with external metadata stores.
- Track Transformations: Make sure each transformation is properly documented within dbt to capture the logic, purpose, and impact of the transformation on the data.
- Validate Data Quality: Set up automated tests within dbt to check the accuracy, completeness, and consistency of the data transformations.
Best Practices for Maximizing dbt's Data Lineage Capabilities
To get the most out of dbt for data lineage, consider these best practices:
- Consistency: Use consistent naming conventions and coding standards across your data models and transformations.
- Version Control: Use version control systems to track changes and manage how your data models and transformations evolve over time.
- Documentation: Keep your documentation up to date, with clear explanations and examples for each data model and transformation.
- Collaboration: Encourage different teams to work together by sharing SQL transformations and taking advantage of dbt's modularity.
Overcoming Challenges in dbt Data Lineage
While dbt is a powerful tool for data lineage, you might face some challenges when implementing and using it. Being aware of these challenges and how to solve them is key to ensuring a smooth data lineage process.
Common Issues in dbt Data Lineage
- Incomplete Lineage: Sometimes, the lineage information captured by dbt might be incomplete due to missing dependencies or undocumented transformations.
- Complex Transformations: dbt might struggle with very complex transformations that involve multiple steps or complicated logic.
- Performance Impact: Tracking lineage for large datasets can sometimes slow down dbt or cause delays in generating lineage reports.
Solutions for dbt Data Lineage Challenges
To overcome these challenges in dbt data lineage, try these solutions:
- Data Profiling: Thoroughly examine your data to identify any missing dependencies or incomplete lineage information in dbt.
- Modular Transformations: Break down complex transformations into smaller, more manageable models to improve performance and make them easier to maintain.
- Optimization: Use dbt's caching and incremental features to reduce the impact on performance when working with large datasets.
In conclusion, dbt is a powerful tool for managing data lineage, helping organizations track and understand their data's journey. While challenges may come up, being proactive and using optimization techniques can help overcome these hurdles, making dbt a valuable asset for data lineage.
Now that you understand the power of data lineage and tools like dbt, why not take your data management to the next level? CastorDoc combines the benefits of data lineage with AI-powered assistance, making it easier than ever to track, understand, and leverage your data.
Our platform integrates advanced governance, cataloging, and lineage capabilities - similar to what we've discussed with dbt - but adds a user-friendly AI assistant to the mix. This creates a powerful tool for enabling self-service analytics across your organization.Don't wait to turn your data into valuable business insights - Try CastorDoc today and experience the future of data management.
You might also like
Get in Touch to Learn More
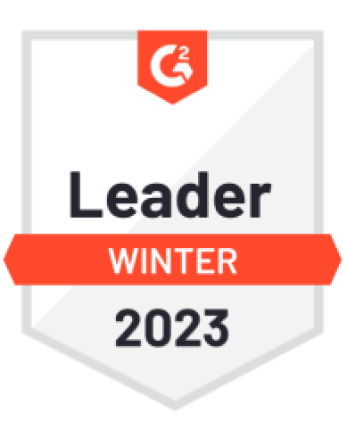
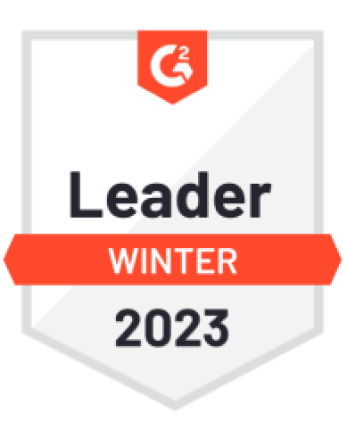

“[I like] The easy to use interface and the speed of finding the relevant assets that you're looking for in your database. I also really enjoy the score given to each table, [which] lets you prioritize the results of your queries by how often certain data is used.” - Michal P., Head of Data