How To Improve Data Quality In 12 Actionable Steps?
Learn 12 actionable steps to enhance data quality in your business operations.
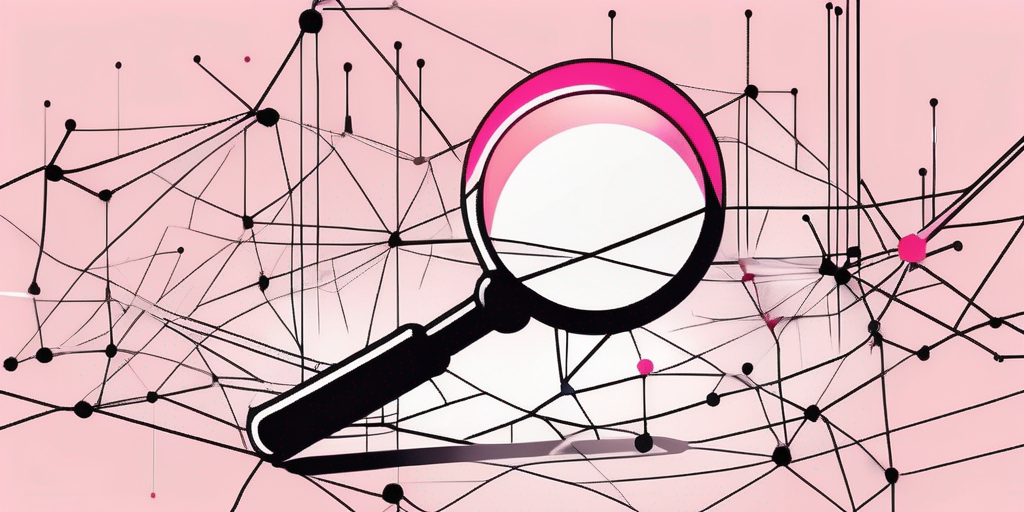
Today, the quality of data holds paramount importance for businesses of all sizes and industries. Accurate and reliable data is the foundation upon which effective decision making and strategic planning are built. It enables organizations to gain valuable insights, optimize operations, and enhance customer experiences. However, poor data quality can have detrimental consequences, ranging from compromised decision making to financial losses and damage to organizational reputation. To avoid such pitfalls, organizations must prioritize the improvement of data quality through a systematic approach comprising various steps and measures. In this article, we will delve into the intricacies of data quality and provide you with a comprehensive guide on how to improve it in 12 actionable steps.
Understanding the Importance of Data Quality
Data plays a pivotal role in decision making, both at the operational and strategic levels. It forms the basis of crucial business processes, including market analysis, customer segmentation, product development, and resource allocation. High-quality data empowers organizations to make informed decisions, identify emerging trends, and seize new opportunities. Conversely, poor data quality can lead to faulty insights, flawed strategies, and failed initiatives. It is therefore imperative for organizations to recognize the significance of data quality and its impact on overall business performance.
The Role of Data in Decision Making
Data serves as the backbone of decision making in organizations. From simple everyday choices to complex strategic plans, data provides the necessary information to support rational and evidence-based decision making. By analyzing data, organizations can identify patterns, understand customer preferences, and predict future trends. This enables them to make data-driven decisions, where intuition is complemented by empirical evidence. Thus, data quality directly influences the reliability and accuracy of decision making processes.
Consequences of Poor Data Quality
The repercussions of poor data quality can be far-reaching and multifaceted. Firstly, inaccurate or incomplete data can lead to faulty analysis and misinterpretation of trends, potentially resulting in misguided strategies and inefficient resource allocation. Secondly, poor data quality can undermine customer relationships and satisfaction when information is incorrect, outdated, or inconsistent. This can lead to missed sales opportunities, negative brand perception, and customer attrition. Finally, regulatory compliance can be compromised if data is not accurate, reliable, or properly secured. Non-compliance can lead to severe penalties, legal disputes, and damage to an organization's reputation.
Moreover, poor data quality can have a detrimental impact on operational efficiency. Inaccurate or inconsistent data can lead to delays in decision making, as employees may need to spend additional time verifying and correcting the data. This can result in missed opportunities, increased costs, and decreased productivity. Additionally, poor data quality can hinder collaboration and communication within an organization. When different departments or teams rely on different sets of data, it can lead to confusion, conflicts, and a lack of alignment in goals and strategies.
Furthermore, the importance of data quality extends beyond the boundaries of individual organizations. In today's interconnected world, organizations often rely on data from external sources, such as suppliers, partners, and industry databases. If the quality of this external data is compromised, it can have a ripple effect on the accuracy and reliability of an organization's own data. This can undermine the trustworthiness of the entire data ecosystem and hinder effective decision making across the board.
Defining Data Quality Metrics
Before embarking on the journey to improve data quality, it is crucial to define relevant metrics that will be used to assess and monitor data quality. These metrics serve as benchmarks against which the effectiveness of data quality improvement efforts will be measured. While there are numerous metrics available, organizations should focus on key dimensions that align with their specific business objectives.
Establishing a robust framework for data quality metrics involves a comprehensive understanding of the organization's data landscape. This includes identifying the sources of data, data flows, storage mechanisms, and data usage patterns. By conducting a thorough data assessment, organizations can pinpoint areas of improvement and tailor their data quality metrics to address specific challenges and opportunities.
Accuracy and Consistency
Accuracy and consistency are fundamental metrics to evaluate data quality. Accuracy refers to the correctness and precision of data, ensuring that the information reflects the actual state of affairs. Consistency ensures that data is uniform and coherent across different sources and systems, avoiding contradictions or discrepancies. By setting benchmarks for accuracy and consistency, organizations can ensure that their data is reliable and trustworthy.
Implementing data validation processes and data governance practices can help maintain high levels of accuracy and consistency. Data validation involves verifying the integrity and quality of data at various touchpoints, such as data entry, extraction, transformation, and loading. On the other hand, data governance establishes policies and procedures to ensure data is managed effectively and consistently across the organization, promoting data quality and integrity.
Completeness and Uniqueness
Completeness measures the extent to which data contains all the required information for the intended purpose. It ensures that no crucial data elements are missing, enabling comprehensive analysis and decision making. Uniqueness, on the other hand, verifies that each record within a dataset is distinct and free from duplicates. By prioritizing completeness and uniqueness, organizations can enhance the comprehensiveness and reliability of their data.
Utilizing data profiling tools and data quality checks can help identify gaps in data completeness and detect duplicate records. Data profiling tools analyze the structure, content, and relationships within datasets, providing insights into data quality issues such as missing values or redundant entries. By leveraging these tools, organizations can proactively address data quality issues and improve the overall accuracy and reliability of their data assets.
Step-by-Step Guide to Improving Data Quality
Now that we understand the importance of data quality and the metrics used to evaluate it, let us delve into the practical steps organizations can take to improve their data quality. The following 12 actionable steps provide a comprehensive framework to enhance data quality across the organization.
Step 1: Data Audit
The first step in improving data quality is to conduct a thorough data audit. This involves assessing the current state of data quality, identifying areas of improvement, and understanding data dependencies and interrelationships. By gaining a holistic view of existing data assets, organizations can lay the foundation for targeted improvement initiatives.
Step 2: Establishing Data Governance
Data governance is a formalized framework of policies, processes, and roles that ensures the effective management and control of data throughout its lifecycle. By establishing data governance, organizations can enforce data quality standards, define ownership and accountability, and streamline data-related processes. This step sets the groundwork for sustainable data quality improvement.
Step 3: Data Cleansing
Data cleansing involves the identification and correction of errors, inconsistencies, and redundancies within datasets. This step typically involves automated tools and algorithms that can detect and rectify common data quality issues, such as misspellings, formatting errors, and missing values. Data cleansing ensures that the data is accurate, complete, and consistent.
Step 4: Data Validation
Data validation verifies the integrity and accuracy of data through various validation techniques. This includes validating data against predefined rules, performing cross-validation between different datasets, and ensuring that data adheres to specific format requirements. By validating data, organizations can identify and correct inconsistencies, ensuring that data meets the defined quality standards.
Step 5: Data Integration
Data integration involves combining data from multiple sources into a unified and consistent format. This step ensures that disparate datasets are harmonized, eliminating data silos and enabling a holistic view of information. By integrating data, organizations can improve data accuracy, completeness, and accessibility, supporting more robust decision making.
Step 6: Data Enrichment
Data enrichment involves enhancing existing datasets by adding additional information from external sources. This can include demographic data, market trends, or geospatial information, depending on the specific needs of the organization. By enriching data, organizations can enhance the quality and depth of insights derived from the data.
Step 7: Data Monitoring
Data monitoring is an ongoing process that ensures the continuous assessment and improvement of data quality. By implementing automated monitoring mechanisms, organizations can detect issues and anomalies in real-time, enabling timely corrective actions. Data monitoring provides organizations with a proactive approach to maintaining data quality.
Step 8: Data Security
Data security is paramount for ensuring data integrity, confidentiality, and availability. Organizations must implement robust security measures, including access controls, encryption, and regular security audits. By prioritizing data security, organizations can prevent unauthorized access, data breaches, and other security incidents that could compromise data quality.
Step 9: Data Training
Data training involves educating employees about the importance of data quality and providing them with the necessary skills to ensure data integrity. This includes training on data entry best practices, data hygiene guidelines, and the use of data quality tools. By empowering employees with data literacy, organizations can foster a culture of data quality and improve overall data governance.
Step 10: Data Maintenance
Data maintenance involves regular updates, cleaning, and validation of datasets to ensure ongoing data quality. This includes periodically revisiting data quality metrics, conducting data quality assessments, and implementing corrective actions as needed. Data maintenance ensures that data remains accurate, complete, and consistent over time.
Step 11: Data Archiving
Data archiving involves the preservation of historical data that is no longer actively used but may still hold value for compliance, analytics, or reference purposes. Archiving data ensures that it is securely stored, easily accessible, and can be retrieved if needed. This step helps organizations maintain data integrity while managing data lifecycle effectively.
Step 12: Continuous Improvement
Improving data quality is an ongoing journey that requires continuous monitoring, evaluation, and improvement. Organizations must establish a feedback loop to capture insights, learn from data quality issues, and refine processes and strategies accordingly. By embracing a culture of continuous improvement, organizations can ensure that data quality remains a top priority.
Improving data quality is a complex and multifaceted endeavor, requiring a comprehensive strategy and commitment at all levels of the organization. By following the 12 actionable steps laid out in this article, organizations can enhance data quality, enable more accurate decision making, and gain a competitive edge in today's data-driven landscape.
You might also like
Get in Touch to Learn More
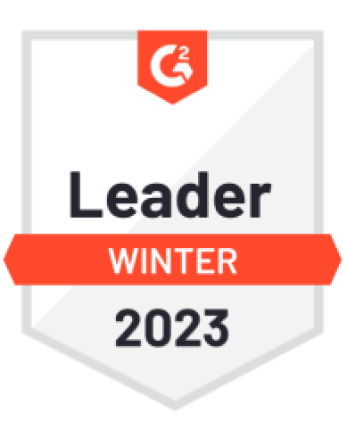
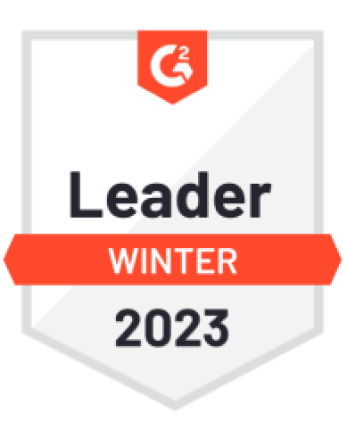

“[I like] The easy to use interface and the speed of finding the relevant assets that you're looking for in your database. I also really enjoy the score given to each table, [which] lets you prioritize the results of your queries by how often certain data is used.” - Michal P., Head of Data