LLM for Data Analysis: Harnessing Large Language Models for Insights
Learn how large language models (LLMs) are changing data analysis and helping businesses find valuable information.
As data sets grow larger, companies are looking for new methods to extract valuable insights from their vast amounts of information. Large Language Models (LLMs) are a new technology that could change how we do data analysis. While LLMs don't cut it for end-to-end data analytics yet, they show great promise in areas like data discovery. In this article, we'll explore LLMs, their role in data analysis, and what their impact might be in the future.
Understanding the Basics of Large Language Models
What are Large Language Models?
An LLM is an AI model trained on huge amounts of text. These models can generate human-like text and understand the context and meaning behind words and sentences. They are able to predict the next word in a sentence, understand grammar and syntax, and even generate entire paragraphs of text.
LLMs are making waves in fields like natural language processing, machine learning, and artificial intelligence. They use deep learning architectures and are often pre-trained on extensive datasets to improve their language understanding capabilities. Models like GPT-4 (Generative Pre-trained Transformer 4) have gained significant attention for their ability to perform a wide range of language-related tasks accurately.
How LLMs Help with Data Analysis
LLMs can change the way we extract insights from data in their ability to process vast amounts of unstructured data, such as text documents, social media posts, customer reviews, and more. This creates new possibilities for analyzing and understanding natural language data.
Businesses can use LLMs for sentiment analysis, topic modeling, text summarization, and even language translation tasks. Their ability to understand and create human-like text helps companies automate processes that involve analyzing and generating text data. This can improve efficiency, accuracy, and scalability in data analysis.

How LLMs Change Data Analysis
New Approaches to Data Analysis
LLMs bring a new level of sophistication to data analysis techniques. Traditional methods often rely on rule-based algorithms that require explicit instructions. LLMs, however, can learn patterns and relationships within the data on their own. This means that analysts can focus on asking the right questions and let the LLM handle the complex task of understanding the context and extracting meaningful insights.
Benefits of Using LLMs for Data Analysis
Using LLMs in data analysis has several advantages. First, LLMs can process unstructured data, which makes up a significant portion of available information. By analyzing text data, organizations can better understand customer sentiment, identify emerging trends, and extract critical information. However, it's important to note that LLMs may struggle with complex reasoning or domain-specific knowledge, and organizations should be cautious about potential hallucinated answers.
LLMs are also good at complex language tasks such as text classification and sentiment analysis. They can accurately categorize documents, identify sentiments, and provide valuable insights for decision-making.
Moreover, LLMs can create human-like text, making them useful for content creation, chatbots, and automated customer service. This helps businesses improve their operations and customer interactions.
Furthermore, LLMs can be fine-tuned for specific industries or use cases, allowing organizations to leverage their domain expertise and tailor the models to their unique needs. This customization makes the insights from LLMs more relevant and actionable, leading to better decision-making and planning.
A Closer Look at LLM Techniques for Data Analysis
Text Classification with LLMs
Text classification is a fundamental task in data analysis. LLMs are good at this because they can understand and categorize text based on its content. This allows organizations to automatically classify support tickets, filter spam emails, and even detect fake news or hate speech.
One big advantage of using LLMs for text classification is that they can learn from huge amounts of text data. This allows them to recognize patterns and relationships that might not be obvious to human analysts. By training LLMs on large datasets, organizations can make their text classification more accurate and efficient, which leads to better decisions and smoother processes.
Sentiment Analysis Using LLMs
Sentiment analysis is another important part of data analysis. Understanding customer sentiment can provide valuable insights for improving products and services. LLMs can analyze the sentiment expressed in customer reviews, social media posts, or survey responses, helping organizations find areas of improvement and make data-driven decisions.
LLMs are particularly good at sentiment analysis because they can understand the nuances of human language and context. By using LLMs for sentiment analysis, organizations can better comprehend customer feedback and preferences, which helps with marketing campaigns, product improvements, and overall customer satisfaction. LLMs can also help businesses track their reputation, notice changes in customer sentiment over time, and quickly address customer concerns.
Challenges in Using LLMs for Data Analysis and How to Solve Them
Dealing with Data Privacy Concerns
As with any advanced data analysis method, using Large Language Models (LLMs) comes with challenges. One big concern is data privacy. LLMs need access to large amounts of training data to work well. However, organizations must handle sensitive data carefully and follow data privacy rules.
Reducing Bias in LLMs
Another challenge when using LLMs in data analysis is potential bias. LLMs are trained on large amounts of text data, which may contain biases from the source material. It's important to carefully choose the training data and use methods to detect and reduce bias to ensure fair analysis. Additionally, organizations must consider the risk of spreading false information if users can't trust the generated results.
Making Sure We Can Understand the Model's Decisions
Another challenge in using LLMs for data analysis is understanding how they make decisions. Because they're complex and have many parameters, LLMs can be hard to interpret. This makes it difficult to understand how they reach specific conclusions. Organizations need to find ways to explain the LLM's reasoning to build trust in its results.
Managing Computational Resources
Finally, the computational resources needed to train and use LLMs for data analysis can be substantial. Training large language models requires powerful computing infrastructure and uses a lot of energy. Organizations need to consider the environmental impact of running LLMs at scale and find ways to use resources efficiently while maintaining performance.
The Future of LLMs in Data Analysis
What's Next for LLMs and Data Analysis
LLMs and data analysis are changing quickly and leading to new developments in artificial intelligence (AI). As technology improves, LLMs will likely get better at understanding language and handling larger datasets. This will help them provide more accurate and detailed insights, changing how we do data analysis.
AI and machine learning are driving these improvements in LLMs. As AI becomes more advanced and is used in more industries, the combination of LLMs and other AI techniques will create new possibilities. Using LLMs with other machine learning methods will improve their analysis capabilities and help with more complex tasks like anomaly detection, predictive modeling, and natural language processing.
How AI and Machine Learning are Improving LLMs
The future of LLMs in data analysis is closely tied to AI and machine learning. As AI technologies continue to develop, the combination of LLMs and other AI techniques will create new opportunities. Combining LLMs with other machine learning algorithms can further improve their capabilities and enable more advanced data analysis tasks, such as anomaly detection and predictive modeling. However, the immediate future may lie in using LLMs for natural language search and asset curation, rather than generating new analyses from scratch. This approach can help organizations maximize the use of existing analytics assets, improving efficiency and accuracy while maintaining better control over data governance.
By using LLMs, organizations can find valuable information in their data. From understanding customer sentiment to predicting trends, LLMs can transform data analysis. As technology continues to advance, LLMs will become an essential tool for data analysts, helping them find deeper insights and make better decisions.
As you start to use Large Language Models for data analysis, you're beginning a journey towards better insights and smarter decisions. CastorDoc offers a reliable AI Assistant for Analytics to help with your strategic needs. Try CastorDoc today to experience the future of data analysis, where self-service analytics, data literacy, and getting the most value from your data become reality. CastorDoc helps your business teams use data effectively while reducing the workload on your data professionals. Start using CastorDoc today to unlock the full potential of your data.
You might also like
Get in Touch to Learn More
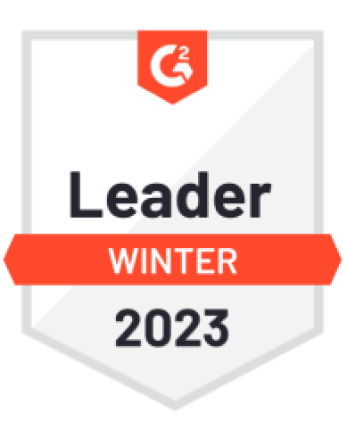
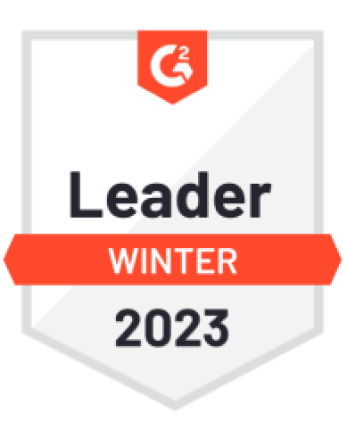

“[I like] The easy to use interface and the speed of finding the relevant assets that you're looking for in your database. I also really enjoy the score given to each table, [which] lets you prioritize the results of your queries by how often certain data is used.” - Michal P., Head of Data